Here is an approach using a KDTree for efficient nearest color lookup. Note that while KDTrees may be pretty advanced, using them is actually quite simple.
import numpy as np
from matplotlib import colors
from scipy.spatial import cKDTree as KDTree
from scipy.misc import face
REDUCED_COLOR_SPACE = True
# borrow a list of named colors from matplotlib
if REDUCED_COLOR_SPACE:
use_colors = {k: colors.cnames[k] for k in ['red', 'green', 'blue', 'black', 'yellow', 'purple']}
else:
use_colors = colors.cnames
# translate hexstring to RGB tuple
named_colors = {k: tuple(map(int, (v[1:3], v[3:5], v[5:7]), 3*(16,)))
for k, v in use_colors.items()}
ncol = len(named_colors)
if REDUCED_COLOR_SPACE:
ncol -= 1
no_match = named_colors.pop('purple')
else:
no_match = named_colors['purple']
# make an array containing the RGB values
color_tuples = list(named_colors.values())
color_tuples.append(no_match)
color_tuples = np.array(color_tuples)
color_names = list(named_colors)
color_names.append('no match')
# get example picture
img = face()
# build tree
tree = KDTree(color_tuples[:-1])
# tolerance for color match `inf` means use best match no matter how
# bad it may be
tolerance = np.inf
# find closest color in tree for each pixel in picture
dist, idx = tree.query(img, distance_upper_bound=tolerance)
# count and reattach names
counts = dict(zip(color_names, np.bincount(idx.ravel(), None, ncol+1)))
print(counts)
import pylab
pylab.imshow(img)
pylab.savefig('orig.png')
pylab.clf()
pylab.imshow(color_tuples[idx])
pylab.savefig('minimal.png' if REDUCED_COLOR_SPACE else 'reduced.png')
Output with full matplotlib named color space:
{'aliceblue': 315, 'antiquewhite': 0, 'aqua': 0, 'aquamarine': 0, 'azure': 0, 'beige': 27, 'bisque': 0, 'black': 88584, 'blanchedalmond': 0, 'blue': 0, 'blueviolet': 0, 'brown': 0, 'burlywood': 76, 'cadetblue': 0, 'chartreuse': 0, 'chocolate': 0, 'coral': 0, 'cornflowerblue': 0, 'cornsilk': 0, 'crimson': 0, 'cyan': 0, 'darkblue': 0, 'darkcyan': 0, 'darkgoldenrod': 0, 'darkgray': 0, 'darkgreen': 4148, 'darkgrey': 71985, 'darkkhaki': 32907, 'darkmagenta': 0, 'darkolivegreen': 90899, 'darkorange': 0, 'darkorchid': 0, 'darkred': 0, 'darksalmon': 0, 'darkseagreen': 30171, 'darkslateblue': 134, 'darkslategray': 108608, 'darkslategrey': 0, 'darkturquoise': 0, 'darkviolet': 0, 'deeppink': 0, 'deepskyblue': 0, 'dimgray': 0, 'dimgrey': 108318, 'dodgerblue': 0, 'firebrick': 0, 'floralwhite': 0, 'forestgreen': 1, 'fuchsia': 0, 'gainsboro': 10438, 'ghostwhite': 736, 'gold': 0, 'goldenrod': 0, 'gray': 0, 'green': 0, 'greenyellow': 0, 'grey': 79835, 'honeydew': 0, 'hotpink': 0, 'indianred': 0, 'indigo': 0, 'ivory': 0, 'khaki': 1056, 'lavender': 4650, 'lavenderblush': 46, 'lawngreen': 0, 'lemonchiffon': 0, 'lightblue': 3, 'lightcoral': 0, 'lightcyan': 0, 'lightgoldenrodyellow': 0, 'lightgray': 11905, 'lightgreen': 2323, 'lightgrey': 0, 'lightpink': 0, 'lightsalmon': 0, 'lightseagreen': 0, 'lightskyblue': 0, 'lightslategray': 0, 'lightslategrey': 31920, 'lightsteelblue': 3590, 'lightyellow': 0, 'lime': 0, 'limegreen': 0, 'linen': 46, 'magenta': 0, 'maroon': 0, 'mediumaquamarine': 0, 'mediumblue': 0, 'mediumorchid': 0, 'mediumpurple': 15, 'mediumseagreen': 0, 'mediumslateblue': 0, 'mediumspringgreen': 0, 'mediumturquoise': 0, 'mediumvioletred': 0, 'midnightblue': 54, 'mintcream': 0, 'mistyrose': 19, 'moccasin': 0, 'navajowhite': 0, 'navy': 0, 'oldlace': 0, 'olive': 0, 'olivedrab': 30828, 'orange': 0, 'orangered': 0, 'orchid': 0, 'palegoldenrod': 1499, 'palegreen': 285, 'paleturquoise': 0, 'palevioletred': 0, 'papayawhip': 0, 'peachpuff': 0, 'peru': 21, 'pink': 0, 'plum': 0, 'powderblue': 0, 'purple': 0, 'rebeccapurple': 0, 'red': 0, 'rosybrown': 2831, 'royalblue': 0, 'saddlebrown': 0, 'salmon': 0, 'sandybrown': 0, 'seagreen': 0, 'seashell': 0, 'sienna': 5, 'silver': 35951, 'skyblue': 0, 'slateblue': 0, 'slategray': 7836, 'slategrey': 0, 'snow': 18, 'springgreen': 0, 'steelblue': 0, 'tan': 3925, 'teal': 0, 'thistle': 10274, 'tomato': 0, 'turquoise': 0, 'violet': 0, 'wheat': 21, 'white': 3, 'whitesmoke': 834, 'yellow': 0, 'yellowgreen': 9292, 'no match': 0}
Output with only basic colors:
{'red': 0, 'green': 403561, 'blue': 3262, 'black': 153782, 'yellow': 225827, 'no match': 0}
Original picture:
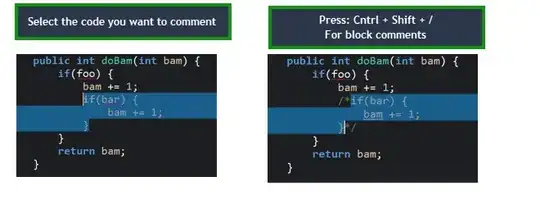
Reduced color version:

Basic color versioin:
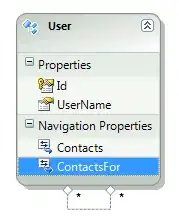