For the 2D case there is a paper: Krystek and Anton, Meas, Sci. Technol. 18 (2007) 3438-3442. I am sure that this can be generalized to 3D. The result would avoid an iterative process, but the details are probably quite cumbersome.
As an alternative an iterative solution may look like this:
import matplotlib.pyplot as plt
import mpl_toolkits.mplot3d as m3d
import numpy as np
from random import random
from scipy.optimize import leastsq, fmin
def line_points( s, p, t ):
return [ s * t[0] + p[0], s * t[1] + p[1], s * t[2] + p[2] ]
def weighted_dist( s, p, t, xVec, sigmaVec ):
q = line_points( s, p, t )
d = ( q[0] - xVec[0] )**2 / sigmaVec[0]**2
d += ( q[1] - xVec[1] )**2 / sigmaVec[1]**2
d += ( q[2] - xVec[2] )**2 / sigmaVec[2]**2
return np.sqrt( d )
def weighted_od( p, t, xVec, sigmaVec ):
f = lambda s: weighted_dist( s, p, t, xVec, sigmaVec )
sol = fmin( f, 0, disp=False )
d = weighted_dist( sol[0], p, t, xVec, sigmaVec )
return d
def residuals( params, data, sigmas ): ###data of type [ allx, ally, allz], sigma of type [allsx, allsy, allsz]
px, py, pz, tx, ty, tz = params
out = list()
for x0, y0, z0, sx, sy, sz in zip( *( data + sigmas ) ):
out += [weighted_od( [ py, py, pz ], [ tx, ty, tz ], [ x0, y0, z0 ], [ sx, sy, sz ] ) ]
print sum(out)
return out
myP = np.array( [ 1 , 1, 3 ] )
myT = np.array( [ -1 ,-3, .8 ] )
myT /= np.linalg.norm( myT )
sList = np.linspace( -3, 3, 100 )
lineList = [ line_points( s, myP, myT ) for s in sList]
xData = [p[0] + .2 * ( 2 * random() - 1 ) for p in lineList ]
yData = [p[1] + .4 * ( 2 * random() - 1 ) for p in lineList ]
zData = [p[2] + .8 * ( 2 * random() - 1 ) for p in lineList ]
xyzData = [ xData, yData, zData ]
sssData = [ len(xData) * [.2], len(xData) * [.4], len(xData) * [.8] ]
residuals( [ 1, 1, 3, -1,-3,.8 ], xyzData, sssData )
myFit, err = leastsq(residuals, [ 1, 1, 2 , -1, -2, -1 ], args=( xyzData, sssData ) )
print myFit
fitP = myFit[:3]
fitT = myFit[3:]
fitTN= np.linalg.norm( fitT )
fitT = [ fitT[0] / fitTN, fitT[1] / fitTN, fitT[2] / fitTN ]
fitLineList = [ line_points( s, fitP, fitT ) for s in sList ]
ax = m3d.Axes3D(plt.figure() )
ax.plot( *zip(*lineList) )
ax.plot( *zip(*fitLineList) )
ax.scatter3D( xData, yData, zData )
plt.show()
Providing:
[ 1. 1.00009764 2.98911266 121.35860193 364.44920212
-92.27043484]
and
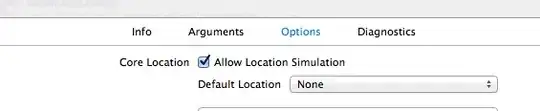
The code surely can be made nicer. One could fit, e.g. theta and phi of the direction vector instead of the three components. A more careful handling of python lists and numpy arrays would help as well, I guess.
For errors and the cov matrix check this