As already emphasized in the comments, pandas does not support "sub-dataframes". For the sake of KISS, I would recommend duplicating those rows (or to manage two separate tables... if really necessary).
The answers in the question you referred to (parsing a dictionary in a pandas dataframe cell into new row cells (new columns)) result in new (frame-wide) columns for each (row-local) "computer name". I doubt that this is what you aim for, considering your domain model.
The abbreviation of pandas can be circumvented by using another output engine, e.g. tabulate (Pretty Printing a pandas dataframe):
# standard pandas output
Vendor BIOS Version Newest BIOS Against M & S W10 Support Computer Location ... Category4 Category5 Category6 Category7 Category8 Category9 Category0
0 Dell Inc. 12.72.9 12.73.9 Yes Yes someName001 12.72.9 ... SomeCategory SomeCategory SomeCategory SomeCategory SomeCategory SomeCategory SomeCategory
1 Dell Inc. 12.72.9 12.73.9 Yes Yes someName002 12.73.9 ... SomeCategory SomeCategory SomeCategory SomeCategory SomeCategory SomeCategory SomeCategory
2 Dell Inc. 12.73.9 12.73.9 Yes Yes someName003 12.73.9 ... SomeCategory SomeCategory SomeCategory SomeCategory SomeCategory SomeCategory SomeCategory
[3 rows x 17 columns]
# tabulate psql (with headers)
+----+------------+----------------+---------------+-----------------+---------------+-------------+------------+--------------+--------------+--------------+--------------+--------------+--------------+--------------+--------------+--------------+--------------+
| | Vendor | BIOS Version | Newest BIOS | Against M & S | W10 Support | Computer | Location | Category1 | Category2 | Category3 | Category4 | Category5 | Category6 | Category7 | Category8 | Category9 | Category0 |
|----+------------+----------------+---------------+-----------------+---------------+-------------+------------+--------------+--------------+--------------+--------------+--------------+--------------+--------------+--------------+--------------+--------------|
| 0 | Dell Inc. | 12.72.9 | 12.73.9 | Yes | Yes | someName001 | 12.72.9 | SomeCategory | SomeCategory | SomeCategory | SomeCategory | SomeCategory | SomeCategory | SomeCategory | SomeCategory | SomeCategory | SomeCategory |
| 1 | Dell Inc. | 12.72.9 | 12.73.9 | Yes | Yes | someName002 | 12.73.9 | SomeCategory | SomeCategory | SomeCategory | SomeCategory | SomeCategory | SomeCategory | SomeCategory | SomeCategory | SomeCategory | SomeCategory |
| 2 | Dell Inc. | 12.73.9 | 12.73.9 | Yes | Yes | someName003 | 12.73.9 | SomeCategory | SomeCategory | SomeCategory | SomeCategory | SomeCategory | SomeCategory | SomeCategory | SomeCategory | SomeCategory | SomeCategory |
+----+------------+----------------+---------------+-----------------+---------------+-------------+------------+--------------+--------------+--------------+--------------+--------------+--------------+--------------+--------------+--------------+--------------+
# tabulate psql
+---+------------+---------+---------+-----+-----+-------------+---------+--------------+--------------+--------------+--------------+--------------+--------------+--------------+--------------+--------------+--------------+
| 0 | Dell Inc. | 12.72.9 | 12.73.9 | Yes | Yes | someName001 | 12.72.9 | SomeCategory | SomeCategory | SomeCategory | SomeCategory | SomeCategory | SomeCategory | SomeCategory | SomeCategory | SomeCategory | SomeCategory |
| 1 | Dell Inc. | 12.72.9 | 12.73.9 | Yes | Yes | someName002 | 12.73.9 | SomeCategory | SomeCategory | SomeCategory | SomeCategory | SomeCategory | SomeCategory | SomeCategory | SomeCategory | SomeCategory | SomeCategory |
| 2 | Dell Inc. | 12.73.9 | 12.73.9 | Yes | Yes | someName003 | 12.73.9 | SomeCategory | SomeCategory | SomeCategory | SomeCategory | SomeCategory | SomeCategory | SomeCategory | SomeCategory | SomeCategory | SomeCategory |
+---+------------+---------+---------+-----+-----+-------------+---------+--------------+--------------+--------------+--------------+--------------+--------------+--------------+--------------+--------------+--------------+
# tabulate plain
Vendor BIOS Version Newest BIOS Against M & S W10 Support Computer Location Category1 Category2 Category3 Category4 Category5 Category6 Category7 Category8 Category9 Category0
0 Dell Inc. 12.72.9 12.73.9 Yes Yes someName001 12.72.9 SomeCategory SomeCategory SomeCategory SomeCategory SomeCategory SomeCategory SomeCategory SomeCategory SomeCategory SomeCategory
1 Dell Inc. 12.72.9 12.73.9 Yes Yes someName002 12.73.9 SomeCategory SomeCategory SomeCategory SomeCategory SomeCategory SomeCategory SomeCategory SomeCategory SomeCategory SomeCategory
2 Dell Inc. 12.73.9 12.73.9 Yes Yes someName003 12.73.9 SomeCategory SomeCategory SomeCategory SomeCategory SomeCategory SomeCategory SomeCategory SomeCategory SomeCategory SomeCategory
You could also use some groupBy(..).apply(..)
+ string magic to produce a string representation which simply hides the duplicates:
# tabulate + merge manually
+----+--------------+------------+----------------+---------------+-----------------+---------------+-------------+------------+--------------+--------------+
| | Type | Vendor | BIOS Version | Newest BIOS | Against M & S | W10 Support | Computer | Location | Category1 | Category2 |
|----+--------------+------------+----------------+---------------+-----------------+---------------+-------------+------------+--------------+--------------|
| 0 | HexaPlex x50 | Dell Inc. | 12.72.9 | 12.73.9 | Yes | Yes | someName001 | 12.72.9 | SomeCategory | SomeCategory |
| | | | 12.72.9 | | | | someName002 | 12.73.9 | | |
| | | | 12.73.9 | | | | someName003 | 12.73.9 | | |
+----+--------------+------------+----------------+---------------+-----------------+---------------+-------------+------------+--------------+--------------+
Styled output can be generated via the new Styling API which is still provisional and under development:
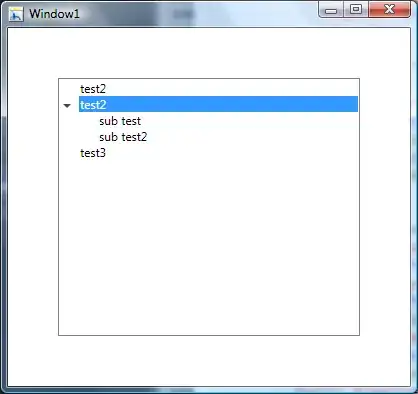
Again, you can use some logic to 'merge' consecutively redundant values in a column (quick example, I assume some more effort could result in much nicer output):
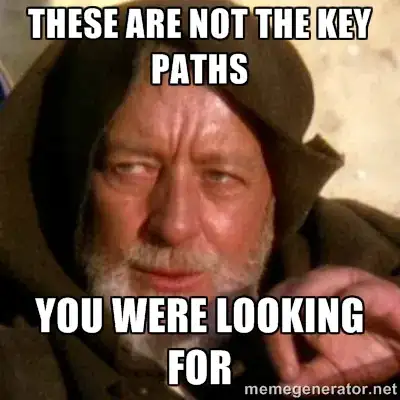
Code for the above examples
import pandas as pd
from tabulate import tabulate
import functools
def pprint(df, headers=True, fmt='psql'):
# https://stackoverflow.com/questions/18528533/pretty-printing-a-pandas-dataframe
print(tabulate(df, headers='keys' if headers else '', tablefmt=fmt))
df = pd.DataFrame({
'Type': ['HexaPlex x50'] * 3,
'Vendor': ['Dell Inc.'] * 3,
'BIOS Version': ['12.72.9', '12.72.9', '12.73.9'],
'Newest BIOS': ['12.73.9'] * 3,
'Against M & S': ['Yes'] * 3,
'W10 Support': ['Yes'] * 3,
'Computer': ['someName001', 'someName002', 'someName003'],
'Location': ['12.72.9', '12.73.9', '12.73.9'],
'Category1': ['SomeCategory'] * 3,
'Category2': ['SomeCategory'] * 3,
'Category3': ['SomeCategory'] * 3,
'Category4': ['SomeCategory'] * 3,
'Category5': ['SomeCategory'] * 3,
'Category6': ['SomeCategory'] * 3,
'Category7': ['SomeCategory'] * 3,
'Category8': ['SomeCategory'] * 3,
'Category9': ['SomeCategory'] * 3,
'Category0': ['SomeCategory'] * 3,
})
print("# standard pandas print")
print(df)
print("\n# tabulate tablefmt=psql (with headers)")
pprint(df)
print("\n# tabulate tablefmt=psql")
pprint(df, headers=False)
print("\n# tabulate tablefmt=plain")
pprint(df, fmt='plain')
def merge_cells_for_print(rows, ls='\n'):
result = pd.DataFrame()
for col in rows.columns:
vals = rows[col].values
if all([val == vals[0] for val in vals]):
result[col] = [vals[0]]
else:
result[col] = [ls.join(vals)]
return result
print("\n# tabulate + merge manually")
pprint(df.groupby('Type').apply(merge_cells_for_print).reset_index(drop=True))
# https://pandas.pydata.org/pandas-docs/stable/style.html
# https://pandas.pydata.org/pandas-docs/version/0.22.0/generated/pandas.io.formats.style.Styler.apply.html#pandas.io.formats.style.Styler.apply
def highlight_lower(ref, col):
return [f'color: {"red" if hgl else ""}' for hgl in col < ref]
def merge_duplicates(col):
vals = col.values
return [''] + ['color: transparent' if curr == pred else '' for pred, curr in zip(vals[1:], vals)]
with open('only_red.html', 'w+') as f:
style = df.style
style = style.apply(functools.partial(highlight_lower, df['Newest BIOS']),
subset=['BIOS Version'])
f.write(style.render())
with open('red_and_merged.html', 'w+') as f:
style = df.style
style = style.apply(functools.partial(highlight_lower, df['Newest BIOS']),
subset=['BIOS Version'])
style = style.apply(merge_duplicates)
f.write(style.render())