In pandas is best dont loop because slow, here is better use rolling
with custom function:
n = 3
df['new'] = (df['col_a'].rolling(n+1, min_periods=1)
.apply(lambda x: (x[-1] < x[:-1]).sum())
.astype(int))
print (df)
col_a new
0 8.4 0
1 11.3 0
2 7.2 2
3 6.5 3
4 4.5 3
5 8.9 0
If performance is important, use strides:
n = 3
x = np.concatenate([[np.nan] * (n), df['col_a'].values])
def rolling_window(a, window):
shape = a.shape[:-1] + (a.shape[-1] - window + 1, window)
strides = a.strides + (a.strides[-1],)
return np.lib.stride_tricks.as_strided(a, shape=shape, strides=strides)
arr = rolling_window(x, n + 1)
df['new'] = (arr[:, :-1] > arr[:, [-1]]).sum(axis=1)
print (df)
col_a new
0 8.4 0
1 11.3 0
2 7.2 2
3 6.5 3
4 4.5 3
5 8.9 0
Performance: Here is used perfplot
in small window n = 3
:
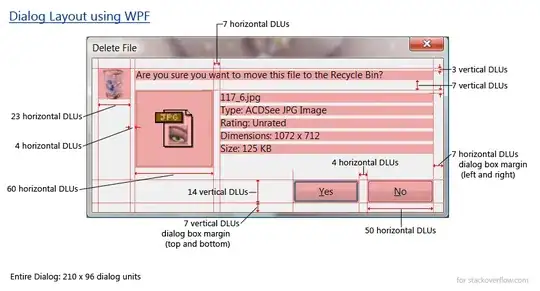
np.random.seed(1256)
n = 3
def rolling_window(a, window):
shape = a.shape[:-1] + (a.shape[-1] - window + 1, window)
strides = a.strides + (a.strides[-1],)
return np.lib.stride_tricks.as_strided(a, shape=shape, strides=strides)
def roll(df):
df['new'] = (df['col_a'].rolling(n+1, min_periods=1).apply(lambda x: (x[-1] < x[:-1]).sum(), raw=True).astype(int))
return df
def list_comp(df):
df['count'] = [(j < df['col_a'].iloc[max(0, i-3):i]).sum() for i, j in df['col_a'].items()]
return df
def strides(df):
x = np.concatenate([[np.nan] * (n), df['col_a'].values])
arr = rolling_window(x, n + 1)
df['new1'] = (arr[:, :-1] > arr[:, [-1]]).sum(axis=1)
return df
def make_df(n):
df = pd.DataFrame(np.random.randint(20, size=n), columns=['col_a'])
return df
perfplot.show(
setup=make_df,
kernels=[list_comp, roll, strides],
n_range=[2**k for k in range(2, 15)],
logx=True,
logy=True,
xlabel='len(df)')
Also I was curious about performance in large window, n = 100
:
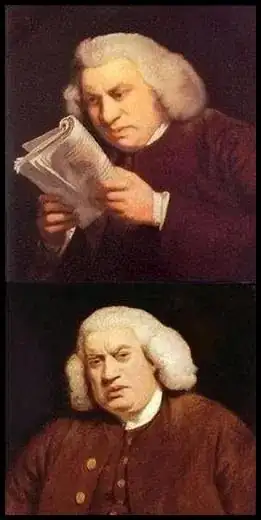