Having as little as 8 points to interpolate between, the resulting plot is not well defined. I.e. a plt.scatter(x,y,c=v)
would look like
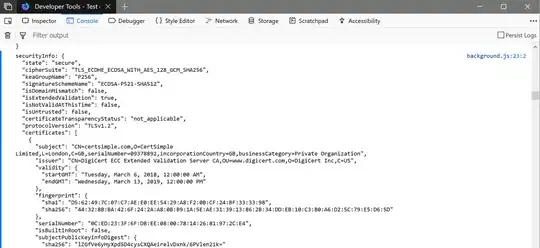
Using a tricontourf
plot like
plt.tricontourf(x,y,v)
would look like
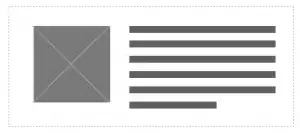
and of course you are free to choose as many levels as you like, e.g. 100
plt.tricontourf(x,y,v,100)
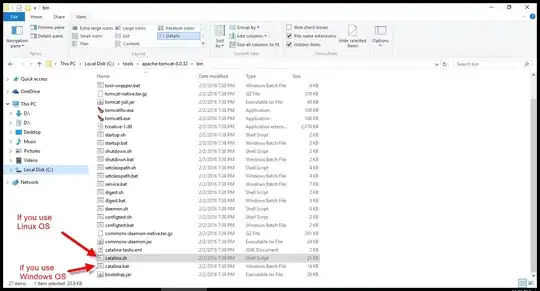
While this looks like the plot in question, I don't think it's well suited for educational purposes, where you would rather teach people about the problem of interpolation and different methods to perform such interpolation.
In that sense, keeping data and plotting seperate is probably a better choice. Hence I would rather do the interpolation first and afterwards display the result. Maybe the Contour plot of irregularly spaced data example is a good starting point here. And an example for the case here could look like
x = [0.0, 0.5, 1.0, 1.0, 1.0, 0.5, 0.0, 0.0];
y = [0.0, 0.0, 0.0, 0.5, 1.0, 1.0, 1.0, 0.5];
v = [0.0, 0.0, 0.0, 0.5, 1.0, 1.0, 1.0, 0.5];
import numpy as np
from scipy.interpolate import griddata
import matplotlib.pyplot as plt
xi = np.linspace(min(x), max(x), 100)
yi = np.linspace(min(y), max(y), 100)
zi = griddata((x, y), v, (xi[None,:], yi[:,None]), method='linear')
plt.pcolormesh(xi,yi,zi)
plt.show()

This uses a pcolormesh
plot, which is easier to grasp for displaying the data on the regular grid, as it does not perform any interpolation itself.