Tjebo's approach was kind of good initially, but after a close look, I found that it found the longest distance between two points on an ellipse. While this is close to what I wanted, it failed with either an irregular shape of the ellipse, or the sparsity of points in the ellipse. This is because it measured the longest distance between two points; whereas what I really wanted is the longest diameter of an ellipse; i.e.: the semi-major axis. See image below for examples/details.
Briefly:
To find/draw density contours of specific density/percentage:
R - How to find points within specific Contour
To get the longest diameter ("semi-major axis") of an ellipse:
https://stackoverflow.com/a/18278767/3579613
For function that returns intercept and slope (as in OP), see last piece of code.
The two pieces of code and images below compare two Tjebo's approach vs. my new approach based on the above posts.
#### Reprex from OP
require(dplyr)
require(ggplot2)
require(MASS)
set.seed(123)
samples <- 10000
r <- 0.9
data <- mvrnorm(n=samples, mu=c(0, 0), Sigma=matrix(c(2, r, r, 2), nrow=2))
x <- data[, 1] # standard normal (mu=0, sd=1)
y <- data[, 2] # standard normal (mu=0, sd=1)
test.df <- data.frame(x = x, y = y)
#### From Tjebo
p <- ggplot(test.df, aes(x, y)) +
geom_density2d(color = 'red', lwd = 0.5, contour = T, h = 2)
p_built <- ggplot_build(p)
p_data <- p_built$data[[1]]
p_maxring <- p_data[p_data[['level']] == min(p_data[['level']]),][,2:3]
coord_mean <- c(x = mean(p_maxring$x), y = mean(p_maxring$y))
p_maxring <- p_maxring %>%
mutate (mean_dev = sqrt((x - mean(x))^2 + (y - mean(y))^2)) #extra column specifying the distance of each point to the mean of those points
p_maxring = p_maxring[round(seq(1, nrow(p_maxring), nrow(p_maxring)/23)),] #### Make a small ellipse to illustrate flaws of approach
coord_farthest <- c('x' = p_maxring$x[which.max(p_maxring$mean_dev)], 'y' = p_maxring$y[which.max(p_maxring$mean_dev)])
# gives the coordinates of the point farthest away from the mean point
farthest_from_farthest <- sqrt((p_maxring$x - coord_farthest['x'])^2 + (p_maxring$y - coord_farthest['y'])^2)
#now this looks which of the points is the farthest from the point farthest from the mean point :D
coord_fff <- c('x' = p_maxring$x[which.max(farthest_from_farthest)], 'y' = p_maxring$y[which.max(farthest_from_farthest)])
farthest_2_points = data.frame(t(cbind(coord_farthest, coord_fff)))
plot(p_maxring[,1:2], asp=1)
lines(farthest_2_points, col = 'blue', lwd = 2)
#### From answer in another post
d = cbind(p_maxring[,1], p_maxring[,2])
r = ellipsoidhull(d)
exy = predict(r) ## the ellipsoid boundary
lines(exy)
me = colMeans((exy))
dist2center = sqrt(rowSums((t(t(exy)-me))^2))
max(dist2center) ## major axis
lines(exy[dist2center == max(dist2center),], col = 'red', lwd = 2)

#### The plot here is made from the data in the reprex in OP, but with h = 0.5
library(MASS)
set.seed(123)
samples <- 10000
r <- 0.9
data <- mvrnorm(n=samples, mu=c(0, 0), Sigma=matrix(c(2, r, r, 2), nrow=2))
x <- data[, 1] # standard normal (mu=0, sd=1)
y <- data[, 2] # standard normal (mu=0, sd=1)
test.df <- data.frame(x = x, y = y)
## MAKE BLUE LINE
p <- ggplot(test.df, aes(x, y)) +
geom_density2d(color = 'red', lwd = 0.5, contour = T, h = 0.5) ## NOTE h = 0.5
p_built <- ggplot_build(p)
p_data <- p_built$data[[1]]
p_maxring <- p_data[p_data[['level']] == min(p_data[['level']]),][,2:3]
coord_mean <- c(x = mean(p_maxring$x), y = mean(p_maxring$y))
p_maxring <- p_maxring %>%
mutate (mean_dev = sqrt((x - mean(x))^2 + (y - mean(y))^2))
coord_farthest <- c('x' = p_maxring$x[which.max(p_maxring$mean_dev)], 'y' = p_maxring$y[which.max(p_maxring$mean_dev)])
farthest_from_farthest <- sqrt((p_maxring$x - coord_farthest['x'])^2 + (p_maxring$y - coord_farthest['y'])^2)
coord_fff <- c('x' = p_maxring$x[which.max(farthest_from_farthest)], 'y' = p_maxring$y[which.max(farthest_from_farthest)])
## MAKE RED LINE
## h = 0.5
## Given the highly irregular shape of the contours, I will use only the largest contour line (0.95) for draing the line.
## Thus, average = 1. See function below for details.
ln = long.diam("x", "y", test.df, h = 0.5, average = 1) ## NOTE h = 0.5
## PLOT
ggplot(test.df, aes(x, y)) +
geom_density2d(color = 'red', lwd = 0.5, contour = T, h = 0.5) + ## NOTE h = 0.5
geom_segment((aes(x = coord_farthest['x'], y = coord_farthest['y'],
xend = coord_fff['x'], yend = coord_fff['y'])), col = 'blue', lwd = 2) +
geom_abline(intercept = ln[1], slope = ln[2], color = 'red', lwd = 2) +
coord_equal()
Finally, I came up with the following function to deal with all this. Sorry for the lack of comments/clarity
#### This will return the intercept and slope of the longest diameter (semi-major axis).
####If Average = TRUE, it will average the int and slope across different density contours.
long.diam = function(x, y, df, probs = c(0.95, 0.5, 0.1), average = T, h = 2) {
fun.df = data.frame(cbind(df[,x], df[,y]))
colnames(fun.df) = c("x", "y")
dens = kde2d(fun.df$x, fun.df$y, n = 200, h = h)
dx <- diff(dens$x[1:2])
dy <- diff(dens$y[1:2])
sz <- sort(dens$z)
c1 <- cumsum(sz) * dx * dy
levels <- sapply(probs, function(x) {
approx(c1, sz, xout = 1 - x)$y
})
names(levels) = paste0("L", str_sub(formatC(probs, 2, format = 'f'), -2))
#plot(fun.df$x,fun.df$y, asp = 1)
#contour(dens, levels = levels, labels=probs, add=T, col = c('red', 'blue', 'green'), lwd = 2)
#contour(dens, add = T, col = 'red', lwd = 2)
#abline(lm(fun.df$y~fun.df$x))
ls <- contourLines(dens, levels = levels)
names(ls) = names(levels)
lines.info = list()
for (i in 1:length(ls)) {
d = cbind(ls[[i]]$x, ls[[i]]$y)
exy = predict(ellipsoidhull(d))## the ellipsoid boundary
colnames(exy) = c("x", "y")
me = colMeans((exy)) ## center of the ellipse
dist2center = sqrt(rowSums((t(t(exy)-me))^2))
#plot(exy,type='l',asp=1)
#points(d,col='blue')
#lines(exy[order(dist2center)[1:2],])
#lines(exy[rev(order(dist2center))[1:2],])
max.dist = data.frame(exy[rev(order(dist2center))[1:2],])
line.fit = lm(max.dist$y ~ max.dist$x)
lines.info[[i]] = c(as.numeric(line.fit$coefficients[1]), as.numeric(line.fit$coefficients[2]))
}
names(lines.info) = names(ls)
#plot(fun.df$x,fun.df$y, asp = 1)
#contour(dens, levels = levels, labels=probs, add=T, col = c('red', 'blue', 'green'), lwd = 2)
#abline(lines.info[[1]], col = 'red', lwd = 2)
#abline(lines.info[[2]], col = 'blue', lwd = 2)
#abline(lines.info[[3]], col = 'green', lwd = 2)
#abline(apply(simplify2array(lines.info), 1, mean), col = 'black', lwd = 4)
if (isTRUE(average)) {
apply(simplify2array(lines.info), 1, mean)
} else {
lines.info[[average]]
}
}
Finally, here's the final implementation of the different answers:
library(MASS)
set.seed(123)
samples = 10000
r = 0.9
data = mvrnorm(n=samples, mu=c(0, 0), Sigma=matrix(c(2, r, r, 2), nrow=2))
x = data[, 1] # standard normal (mu=0, sd=1)
y = data[, 2] # standard normal (mu=0, sd=1)
#plot(x, y)
test.df = data.frame(x = x, y = y)
#### Find furthest two points of contour
## BLUE
p <- ggplot(test.df, aes(x, y)) +
geom_density2d(color = 'red', lwd = 2, contour = T, h = 2)
p_built <- ggplot_build(p)
p_data <- p_built$data[[1]]
p_maxring <- p_data[p_data[['level']] == min(p_data[['level']]),][,2:3]
coord_mean <- c(x = mean(p_maxring$x), y = mean(p_maxring$y))
p_maxring <- p_maxring %>%
mutate (mean_dev = sqrt((x - mean(x))^2 + (y - mean(y))^2))
coord_farthest <- c('x' = p_maxring$x[which.max(p_maxring$mean_dev)], 'y' = p_maxring$y[which.max(p_maxring$mean_dev)])
farthest_from_farthest <- sqrt((p_maxring$x - coord_farthest['x'])^2 + (p_maxring$y - coord_farthest['y'])^2)
coord_fff <- c('x' = p_maxring$x[which.max(farthest_from_farthest)], 'y' = p_maxring$y[which.max(farthest_from_farthest)])
#### Find the average intercept and slope of 3 contour lines (0.95, 0.5, 0.1), as in my long.diam function above.
## RED
ln = long.diam("x", "y", test.df)
#### Plot everything. Black line is GLM
ggplot(test.df, aes(x, y)) +
geom_point(color = 'grey') +
geom_density2d(color = 'red', lwd = 1, contour = T, h = 2) +
geom_smooth(method = "glm", se = F, lwd = 1, color = 'black') +
geom_abline(intercept = ln[1], slope = ln[2], col = 'red', lwd = 1) +
geom_segment((aes(x = coord_farthest['x'], y = coord_farthest['y'],
xend = coord_fff['x'], yend = coord_fff['y'])), col = 'blue', lwd = 1) +
coord_equal()
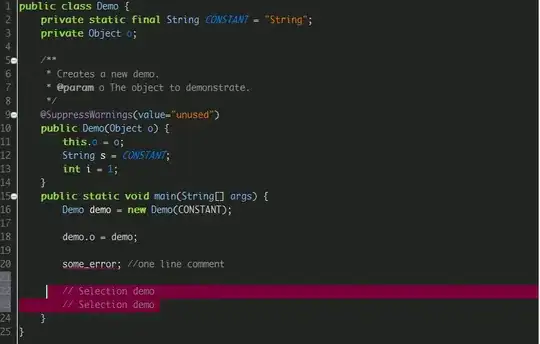