An alternative approach, using a common base class with virtual methods:
#include <iostream>
struct IntOp {
virtual int get() = 0;
};
struct ConstInt: IntOp {
int n;
explicit ConstInt(int n): n(n) { }
virtual int get() override { return n; }
};
struct MultiplyIntInt: IntOp {
IntOp *pArg1, *pArg2;
MultiplyIntInt(IntOp *pArg1, IntOp *pArg2): pArg1(pArg1), pArg2(pArg2) { }
virtual int get() override { return pArg1->get() * pArg2->get(); }
};
int main()
{
ConstInt i3(3), i4(4);
MultiplyIntInt i3muli4(&i3, &i4);
std::cout << i3.get() << " * " << i4.get() << " = " << i3muli4.get() << '\n';
return 0;
}
Output:
3 * 4 = 12
Live Demo on coliru
As I mentioned std::function
in post-answer conversation with OP, I fiddled a bit with this idea and got this:
#include <iostream>
#include <functional>
struct MultiplyIntInt {
std::function<int()> op1, op2;
MultiplyIntInt(std::function<int()> op1, std::function<int()> op2): op1(op1), op2(op2) { }
int get() { return op1() * op2(); }
};
int main()
{
auto const3 = []() -> int { return 3; };
auto const4 = []() -> int { return 4; };
auto rand100 = []() -> int { return rand() % 100; };
MultiplyIntInt i3muli4(const3, const4);
MultiplyIntInt i3muli4mulRnd(
[&]() -> int { return i3muli4.get(); }, rand100);
for (int i = 1; i <= 10; ++i) {
std::cout << i << ".: 3 * 4 * rand() = "
<< i3muli4mulRnd.get() << '\n';
}
return 0;
}
Output:
1.: 3 * 4 * rand() = 996
2.: 3 * 4 * rand() = 1032
3.: 3 * 4 * rand() = 924
4.: 3 * 4 * rand() = 180
5.: 3 * 4 * rand() = 1116
6.: 3 * 4 * rand() = 420
7.: 3 * 4 * rand() = 1032
8.: 3 * 4 * rand() = 1104
9.: 3 * 4 * rand() = 588
10.: 3 * 4 * rand() = 252
Live Demo on coliru
With std::function<>
, class methods, free-standing functions, and even lambdas can be used in combination. So, there is no base class anymore needed for nodes. Actually, even nodes are not anymore needed (explicitly) (if a free-standing function or lambda is not counted as "node").
I must admit that graphical dataflow programming was subject of my final work in University (though this is a long time ago). I remembered that I distinguished
- demand-driven execution vs.
- data-driven execution.
Both examples above are demand-driven execution. (The result is requested and "pulls" the arguments.)
So, my last sample is dedicated to show a simplified data-driven execution (in principle):
#include <iostream>
#include <vector>
#include <functional>
struct ConstInt {
int n;
std::vector<std::function<void(int)>> out;
ConstInt(int n): n(n) { eval(); }
void link(std::function<void(int)> in)
{
out.push_back(in); eval();
}
void eval()
{
for (std::function<void(int)> &f : out) f(n);
}
};
struct MultiplyIntInt {
int n1, n2; bool received1, received2;
std::vector<std::function<void(int)>> out;
void set1(int n) { n1 = n; received1 = true; eval(); }
void set2(int n) { n2 = n; received2 = true; eval(); }
void link(std::function<void(int)> in)
{
out.push_back(in); eval();
}
void eval()
{
if (received1 && received2) {
int prod = n1 * n2;
for (std::function<void(int)> &f : out) f(prod);
}
}
};
struct Print {
const char *text;
explicit Print(const char *text): text(text) { }
void set(int n)
{
std::cout << text << n << '\n';
}
};
int main()
{
// setup data flow
Print print("Result: ");
MultiplyIntInt mul;
ConstInt const3(3), const4(4);
// link nodes
const3.link([&mul](int n) { mul.set1(n); });
const4.link([&mul](int n) { mul.set2(n); });
mul.link([&print](int n) { print.set(n); });
// done
return 0;
}
With the dataflow graph image (provided by koman900 – the OP) in mind, the out
vectors represent outputs of nodes, where the methods set()
/set1()
/set2()
represent inputs.
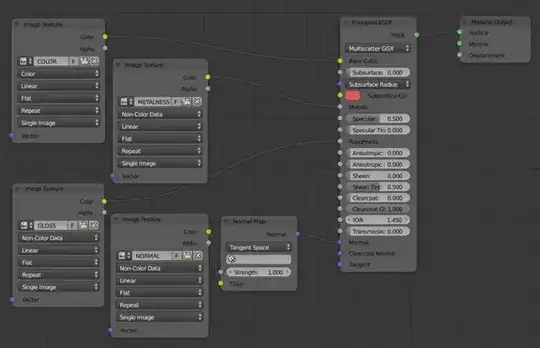
Output:
Result: 12
Live Demo on coliru
After connection of graph, the source nodes (const3
and const4
) may push new results to their output which may or may not cause following operations to recompute.
For a graphical presentation, the operator classes should provide additionally some kind of infrastructure (e.g. to retrieve a name/type and the available inputs and outputs, and, may be, signals for notification about state changes).
Surely, it is possible to combine both approaches (data-driven and demand-driven execution). (A node in the middle may change its state and requests new input to push new output afterwards.)