This example code uses an equation that has two shape parameters, a and b, and an offset term (that does not affect curvature). The equation is "y = 1.0 / (1.0 + exp(-a(x-b))) + Offset" with parameter values a = 2.1540318329369712E-01, b = -6.6744890642157646E+00, and Offset = -3.5241299859669645E-01 which gives an R-squared of 0.988 and an RMSE of 0.0085.
The example contains your posted data with Python code for fitting and graphing, with automatic initial parameter estimation using the scipy.optimize.differential_evolution genetic algorithm. The scipy implementation of Differential Evolution uses the Latin Hypercube algorithm to ensure a thorough search of parameter space, and this requires bounds within which to search - in this example code, these bounds are based on the maximum and minimum data values.
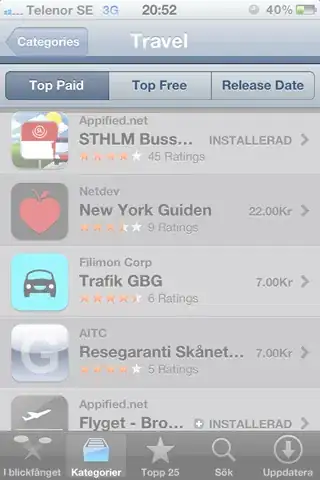
import numpy, scipy, matplotlib
import matplotlib.pyplot as plt
from scipy.optimize import curve_fit
from scipy.optimize import differential_evolution
import warnings
xData = numpy.array([19.1647, 18.0189, 16.9550, 15.7683, 14.7044, 13.6269, 12.6040, 11.4309, 10.2987, 9.23465, 8.18440, 7.89789, 7.62498, 7.36571, 7.01106, 6.71094, 6.46548, 6.27436, 6.16543, 6.05569, 5.91904, 5.78247, 5.53661, 4.85425, 4.29468, 3.74888, 3.16206, 2.58882, 1.93371, 1.52426, 1.14211, 0.719035, 0.377708, 0.0226971, -0.223181, -0.537231, -0.878491, -1.27484, -1.45266, -1.57583, -1.61717])
yData = numpy.array([0.644557, 0.641059, 0.637555, 0.634059, 0.634135, 0.631825, 0.631899, 0.627209, 0.622516, 0.617818, 0.616103, 0.613736, 0.610175, 0.606613, 0.605445, 0.603676, 0.604887, 0.600127, 0.604909, 0.588207, 0.581056, 0.576292, 0.566761, 0.555472, 0.545367, 0.538842, 0.529336, 0.518635, 0.506747, 0.499018, 0.491885, 0.484754, 0.475230, 0.464514, 0.454387, 0.444861, 0.437128, 0.415076, 0.401363, 0.390034, 0.378698])
def func(x, a, b, Offset): # Sigmoid A With Offset from zunzun.com
return 1.0 / (1.0 + numpy.exp(-a * (x-b))) + Offset
# function for genetic algorithm to minimize (sum of squared error)
def sumOfSquaredError(parameterTuple):
warnings.filterwarnings("ignore") # do not print warnings by genetic algorithm
val = func(xData, *parameterTuple)
return numpy.sum((yData - val) ** 2.0)
def generate_Initial_Parameters():
# min and max used for bounds
maxX = max(xData)
minX = min(xData)
maxY = max(yData)
minY = min(yData)
parameterBounds = []
parameterBounds.append([minX, maxX]) # search bounds for a
parameterBounds.append([minX, maxX]) # search bounds for b
parameterBounds.append([0.0, maxY]) # search bounds for Offset
# "seed" the numpy random number generator for repeatable results
result = differential_evolution(sumOfSquaredError, parameterBounds, seed=3)
return result.x
# generate initial parameter values
geneticParameters = generate_Initial_Parameters()
# curve fit the test data
fittedParameters, pcov = curve_fit(func, xData, yData, geneticParameters)
print('Parameters', fittedParameters)
modelPredictions = func(xData, *fittedParameters)
absError = modelPredictions - yData
SE = numpy.square(absError) # squared errors
MSE = numpy.mean(SE) # mean squared errors
RMSE = numpy.sqrt(MSE) # Root Mean Squared Error, RMSE
Rsquared = 1.0 - (numpy.var(absError) / numpy.var(yData))
print('RMSE:', RMSE)
print('R-squared:', Rsquared)
##########################################################
# graphics output section
def ModelAndScatterPlot(graphWidth, graphHeight):
f = plt.figure(figsize=(graphWidth/100.0, graphHeight/100.0), dpi=100)
axes = f.add_subplot(111)
# first the raw data as a scatter plot
axes.plot(xData, yData, 'D')
# create data for the fitted equation plot
xModel = numpy.linspace(min(xData), max(xData))
yModel = func(xModel, *fittedParameters)
# now the model as a line plot
axes.plot(xModel, yModel)
axes.set_xlabel('X Data') # X axis data label
axes.set_ylabel('Y Data') # Y axis data label
plt.show()
plt.close('all') # clean up after using pyplot
graphWidth = 800
graphHeight = 600
ModelAndScatterPlot(graphWidth, graphHeight)