First option is to make a big file out of small parquet files at source level is to merge them together as multiple files > 128 mb sized files or what ever size you wanted
how to merge multiple parquet files to single parquet file using linux or hdfs command?
Second option i.e. Using spark : read small parquet files and then before actual data business processing logic using spark and write them in to relatively big sized files as expected by you (by taking performance factors in to consideration)
Second option :
Even though what is your spark job configuration I am not aware... But in general coalesce
should work.... try like below example (master -> local but change it to yarn for your app) which worked for me.
here in this example I took small files "./userdata*.parquet" (5 small files all around 110 KB) under src/main/resources
and merged in to final 2 files with coalesce
...
Approach : Read each parquet file as a dataframe and then union to make single dataframe and then coalesce
it.
package com.examples
import org.apache.hadoop.conf._
import org.apache.hadoop.fs._
import org.apache.log4j.{Level, Logger}
import org.apache.spark.internal.Logging
import org.apache.spark.sql.{DataFrame, SaveMode, SparkSession}
import scala.collection.mutable
/** *
* take small pegs and make a large peg
* and coalesce it
*
* @author : Ram Ghadiyaram
*/
object ParquetPlay extends Logging {
Logger.getLogger("org").setLevel(Level.OFF)
//public FileStatus[] globStatus(Path pathPattern) throws IOException
def main(args: Array[String]): Unit = {
val appName = if (args.length >0) args(0) else this.getClass.getName
val spark: SparkSession = SparkSession.builder
.config("spark.master", "local")
.appName(appName)
.getOrCreate()
val fs = FileSystem.get(new Configuration())
val files = fs.globStatus(new Path("./userdata*.parquet")).map(_.getPath.toString)
val dfSeq = mutable.MutableList[DataFrame]()
println(dfSeq)
println(files.length)
files.foreach(x => println(x))
val newDFs = files.map(dir => {
dfSeq += spark.read.parquet(dir).toDF()
})
println(dfSeq.length)
val finalDF = dfSeq.reduce(_ union _)
.toDF
finalDF.show(false)
println(System.getProperty("java.io.tmpdir"))
println(System.getProperties.toString)
finalDF.coalesce(2)
.write
.mode(SaveMode.Overwrite)
.parquet(s"${System.getProperty("java.io.tmpdir")}/final.parquet")
println("done")
}
}
Result : Nearly equal sized 2 files like below... here in the example again it generated small files but in your case since you have
500KB size and around 600 files you can see the size of the file and
you can decide coalesce
(number of partitions of your expectation)
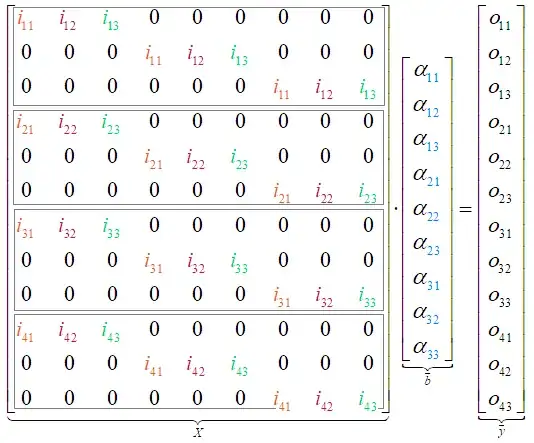
Third Option : As Minh (original poster) mentioned in the comment ... there might be big files which are highly compressed which become small after compression might be causing this.