I just found a chunk of code on github that does this
class LogitScale(mscale.ScaleBase):
"""
Scales data in range 0,1 to -infty, +infty
"""
# The scale class must have a member ``name`` that defines the
# string used to select the scale.
name = 'logit'
def __init__(self, axis, **kwargs):
"""
Any keyword arguments passed to ``set_xscale`` and
``set_yscale`` will be passed along to the scale's
constructor.
thresh: The degree above which to crop the data.
"""
mscale.ScaleBase.__init__(self)
p_min = kwargs.pop("p_min", 1e-5)
if not (0 <p_min < 0.5):
raise ValueError("p_min must be between 0 and 0.5 excluded")
p_max = kwargs.pop("p_max", 1-p_min)
if not (0.5 < p_max < 1):
raise ValueError("p_max must be between 0.5 and 1 excluded")
self.p_min = p_min
self.p_max = p_max
def get_transform(self):
"""
Override this method to return a new instance that does the
actual transformation of the data.
"""
return self.LogitTransform(self.p_min, self.p_max)
def set_default_locators_and_formatters(self, axis):
expon_major = np.arange(1, 18)
# ..., 0.01, 0.1, 0.5, 0.9, 0.99, ...
axis.set_major_locator(FixedLocator(
list(1/10.**(expon_major)) + \
[0.5] + \
list(1-1/10.**(expon_major))
))
minor_ticks = [0.2,0.3,0.4,0.6,0.7, 0.8]
for i in range(2,17):
minor_ticks.extend(1/10.**i * np.arange(2,10))
minor_ticks.extend(1-1/10.**i * np.arange(2,10))
axis.set_minor_locator(FixedLocator(minor_ticks))
axis.set_major_formatter(ProbaFormatter())
axis.set_minor_formatter(ProbaFormatter())
def limit_range_for_scale(self, vmin, vmax, minpos):
return max(vmin, self.p_min), min(vmax, self.p_max)
class LogitTransform(mtransforms.Transform):
input_dims = 1
output_dims = 1
is_separable = True
def __init__(self, p_min, p_max):
mtransforms.Transform.__init__(self)
self.p_min = p_min
self.p_max = p_max
def transform_non_affine(self, a):
"""logit transform (base 10)"""
p_over = a > self.p_max
p_under = a < self.p_min
# saturate extreme values:
a_sat = np.where(p_over, self.p_max, a)
a_sat = np.where(p_under, self.p_min, a_sat)
return np.log10(a_sat / (1-a_sat))
def inverted(self):
return LogitScale.InvertedLogitTransform(self.p_min, self.p_max)
class InvertedLogitTransform(mtransforms.Transform):
input_dims = 1
output_dims = 1
is_separable = True
def __init__(self, p_min, p_max):
mtransforms.Transform.__init__(self)
self.p_min = p_min
self.p_max = p_max
def transform_non_affine(self, a):
"""sigmoid transform (base 10)"""
return 1/(1+10**(-a))
def inverted(self):
return LogitScale.LogitTransform(self.p_min, self.p_max)
mscale.register_scale(LogitScale)
if __name__ == '__main__':
import matplotlib.pyplot as plt
x = np.array([.5, .8, .9, .999, .9999, .99999])
y = np.array([1, 1, 1, 1, .1, .01])
plt.plot(x, y)
plt.xticks(x)
plt.gca().set_xscale('logit')
plt.grid(True)
plt.show()
And my result looks good enough:
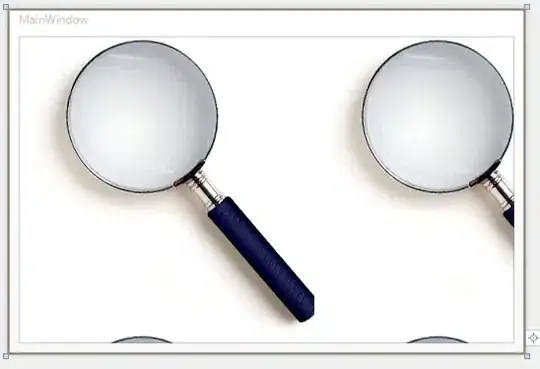