For a more general solution that works for models besides Logistic Regression (like Decision Trees or Random Forest which lack a model summary) you can get the ROC curve using BinaryClassificationMetrics from Spark MLlib.
Note that the PySpark version doesn't implement all of the methods that the Scala version does, so you'll need to use the .call(name)
function from JavaModelWrapper. It also seems that py4j doesn't support parsing scala.Tuple2
classes, so they have to be manually processed.
Example:
from pyspark.mllib.evaluation import BinaryClassificationMetrics
# Scala version implements .roc() and .pr()
# Python: https://spark.apache.org/docs/latest/api/python/_modules/pyspark/mllib/common.html
# Scala: https://spark.apache.org/docs/latest/api/java/org/apache/spark/mllib/evaluation/BinaryClassificationMetrics.html
class CurveMetrics(BinaryClassificationMetrics):
def __init__(self, *args):
super(CurveMetrics, self).__init__(*args)
def _to_list(self, rdd):
points = []
# Note this collect could be inefficient for large datasets
# considering there may be one probability per datapoint (at most)
# The Scala version takes a numBins parameter,
# but it doesn't seem possible to pass this from Python to Java
for row in rdd.collect():
# Results are returned as type scala.Tuple2,
# which doesn't appear to have a py4j mapping
points += [(float(row._1()), float(row._2()))]
return points
def get_curve(self, method):
rdd = getattr(self._java_model, method)().toJavaRDD()
return self._to_list(rdd)
Usage:
import matplotlib.pyplot as plt
# Create a Pipeline estimator and fit on train DF, predict on test DF
model = estimator.fit(train)
predictions = model.transform(test)
# Returns as a list (false positive rate, true positive rate)
preds = predictions.select('label','probability').rdd.map(lambda row: (float(row['probability'][1]), float(row['label'])))
points = CurveMetrics(preds).get_curve('roc')
plt.figure()
x_val = [x[0] for x in points]
y_val = [x[1] for x in points]
plt.title(title)
plt.xlabel(xlabel)
plt.ylabel(ylabel)
plt.plot(x_val, y_val)
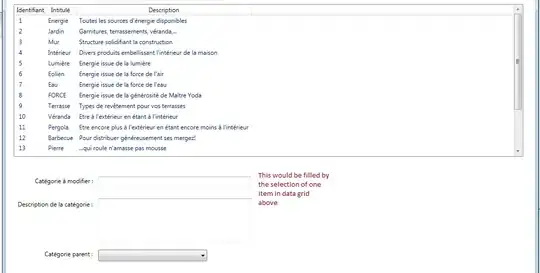
BinaryClassificationMetrics in Scala implements several other useful methods as well:
metrics = CurveMetrics(preds)
metrics.get_curve('fMeasureByThreshold')
metrics.get_curve('precisionByThreshold')
metrics.get_curve('recallByThreshold')