Naive version
As I understand your question: you have an input image, you transform its pixel positions, and want to put the result into a larger array that can accommodate it. Here's how I'd do that:
import numpy as np
import matplotlib.pyplot as plt # for plotting the result
from scipy.misc import face # for dummy data
img = face() # dummy RGB data
# transform pixels by 45 degrees
i,j = np.mgrid[:img.shape[0], :img.shape[1]] # 2d arrays each
T = np.array([[1, -1],[1, 1]])/np.sqrt(2)
inew,jnew = T @ [i.ravel(), j.ravel()] # 1d arrays each
# new coordinates now range into negatives, shift back into positives
# and the non-integer pixel indices will be normalized with floor
inew = np.floor(inew - inew.min()).astype(int)
jnew = np.floor(jnew - jnew.min()).astype(int)
# now the new coordinates are all non-negative, this defines the size of the output
out = np.zeros((inew.max() + 1, jnew.max() + 1, 3), dtype=img.dtype)
# fill the necessary indices of out with pixels from img
# reshape the indices to 2d for matching broadcast
inew = inew.reshape(img.shape[:-1])
jnew = jnew.reshape(img.shape[:-1])
out[inew, jnew, :] = img
# OR, alternative with 1d index arrays:
#out[inew, jnew, :] = img.reshape(-1, 3)
# check what we've done
plt.imshow(out)
plt.show()
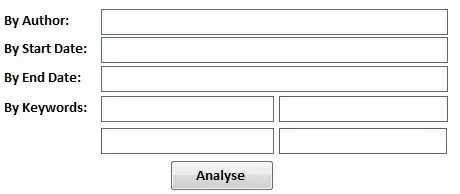
The gist of the code is that the rotated pixel coordinates are shifted back into the positives (this corresponds to your [i+a, j+b]
shift), a new zero array is allocated that will fit all the new indices, and indexing is applied only on the right-hand-side! This is what doesn't match your code, but I believe this is what you really want to do: for each pixel in the original (unindexed) image we set its RGB value in the new position of the resulting array.
As you can see, there are a lot of black pixels in the image due to the fact that the non-integer transformed coordinates were rounded with floor
. This is not nice, so if we pursue this path we should perform 2d interpolation in order to get rid of these artifacts. Note that this needs quite a bit of memory and CPU time:
import numpy as np
import scipy.interpolate as interp
import matplotlib.pyplot as plt # for plotting the result
from scipy.misc import face # for dummy data
img = face() # dummy RGB data
# transform pixels by 45 degrees
i,j = np.mgrid[:img.shape[0], :img.shape[1]] # 2d arrays each
T = np.array([[1, -1],[1, 1]])/np.sqrt(2)
inew,jnew = T @ [i.ravel(), j.ravel()] # 1d arrays each
# new coordinates now range into negatives, shift back into positives
# keep them non-integer for interpolation later
inew -= inew.min()
jnew -= jnew.min()
# (inew, jnew, img) contain the data from which the output should be interpolated
# now the new coordinates are all non-negative, this defines the size of the output
out = np.zeros((int(round(inew.max())) + 1, int(round(jnew.max())) + 1, 3), dtype=img.dtype)
i_interp,j_interp = np.mgrid[:out.shape[0], :out.shape[1]]
# interpolate for each channel
for channel in range(3):
out[..., channel] = interp.griddata(np.array([inew.ravel(), jnew.ravel()]).T, img[..., channel].ravel(), (i_interp, j_interp), fill_value=0)
# check what we've done
plt.imshow(out)
plt.show()
At least the result looks much better:

scipy.ndimage: map_coordinates
An approach that is directly along what you had in mind can make use of scipy.ndimage.map_coordinates
to perform interpolation using the inverse transformation. This should have better performance than the previous attempt with griddata
, since map_coordinates
can make use of the fact that the input data is defined on a grid. It turns out that it indeed uses both less memory and much less CPU:
import numpy as np
import scipy.ndimage as ndi
import matplotlib.pyplot as plt # for plotting the result
from scipy.misc import face # for dummy data
img = face() # dummy RGB data
n,m = img.shape[:-1]
# transform pixels by 45 degrees
T = np.array([[1, -1],[1, 1]])/np.sqrt(2)
# find out the extent of the transformed pixels from the four corners
inew_tmp,jnew_tmp = T @ [[0, 0, n-1, n-1], [0, m-1, 0, m-1]] # 1d arrays each
imin,imax,jmin,jmax = inew_tmp.min(),inew_tmp.max(),jnew_tmp.min(),jnew_tmp.max()
imin,imax,jmin,jmax = (int(round(val)) for val in (imin,imax,jmin,jmax))
# so the pixels of the original map inside [imin, imax] x [jmin, jmax]
# we need an image of size (imax - imin + 1, jmax - jmin + 1) to house this
out = np.zeros((imax - imin + 1, jmax - jmin + 1, 3), dtype=img.dtype)
# indices have to be shifted by [imin, imax]
# compute the corresponding (non-integer) coordinates on the domain for interpolation
inew,jnew = np.mgrid[:out.shape[0], :out.shape[1]]
i_back,j_back = np.linalg.inv(T) @ [inew.ravel() + imin, jnew.ravel() + jmin]
# perform 2d interpolation for each colour channel separately
for channel in range(3):
out[inew, jnew, channel] = ndi.map_coordinates(img[..., channel], [i_back, j_back]).reshape(inew.shape)
# check what we've done
plt.imshow(out)
plt.show()
The result is still nice:

scipy.ndimage: geometric_transform
Finally, I realized that we can go one level higher and use scipy.ndimage.geometric_transform
directly. For the rotated raccoon case this seems to be slower than the manual version using map_coordinates
, but leads to cleaner code:
import numpy as np
import scipy.ndimage as ndi
import matplotlib.pyplot as plt # for plotting the result
from scipy.misc import face # for dummy data
img = face() # dummy RGB data
n,m = img.shape[:-1]
# transform pixels by 45 degrees
T = np.array([[1, -1],[1, 1]])/np.sqrt(2)
Tinv = np.linalg.inv(T)
# find out the extent of the transformed pixels from the four corners
inew_tmp,jnew_tmp = T @ [[0, 0, n-1, n-1], [0, m-1, 0, m-1]] # 1d arrays each
imin,imax,jmin,jmax = inew_tmp.min(),inew_tmp.max(),jnew_tmp.min(),jnew_tmp.max()
imin,imax,jmin,jmax = (int(round(val)) for val in (imin,imax,jmin,jmax))
# so the pixels of the original map inside [imin, imax] x [jmin, jmax]
# we need an image of size (imax - imin + 1, jmax - jmin + 1) to house this
def transform_func(output_coords):
"""Inverse transform output coordinates back into input coordinates"""
inew,jnew,channel = output_coords
i,j = Tinv @ [inew + imin, jnew + jmin]
return i,j,channel
out = ndi.geometric_transform(img, transform_func, output_shape = (imax - imin + 1, jmax - jmin + 1, 3))
# check what we've done
plt.imshow(out)
plt.show()
Result:
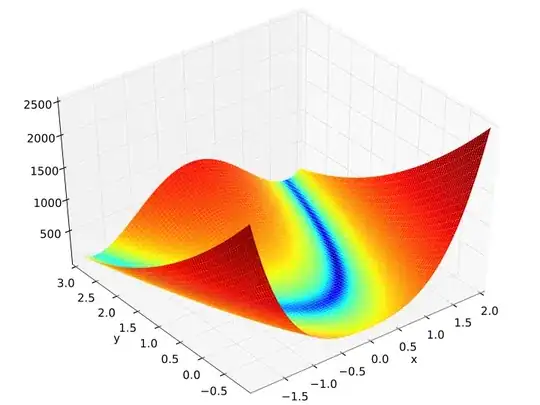
Final fix: only numpy
I was primarily concerned with image quality, so all of the above solutions use interpolation in one way or the other. As you explained in comments, this is not of primary concern to you. If this is the case we can modify the version using map_coordinates
and calculate approximate (rounded integer) indices ourselves and perform the vectorized assignment:
import numpy as np
import matplotlib.pyplot as plt # for plotting the result
from scipy.misc import face # for dummy data
img = face() # dummy RGB data
n,m = img.shape[:-1]
# transform pixels by 45 degrees
T = np.array([[1, -1],[1, 1]])/np.sqrt(2)
# find out the extent of the transformed pixels from the four corners
inew_tmp,jnew_tmp = T @ [[0, 0, n-1, n-1], [0, m-1, 0, m-1]] # 1d arrays each
imin,imax,jmin,jmax = inew_tmp.min(),inew_tmp.max(),jnew_tmp.min(),jnew_tmp.max()
imin,imax,jmin,jmax = (int(round(val)) for val in (imin,imax,jmin,jmax))
# so the pixels of the original map inside [imin, imax] x [jmin, jmax]
# we need an image of size (imax - imin + 1, jmax - jmin + 1) to house this
out = np.zeros((imax - imin + 1, jmax - jmin + 1, 3), dtype=img.dtype)
# compute the corresponding coordinates on the domain for matching
inew,jnew = np.mgrid[:out.shape[0], :out.shape[1]]
inew = inew.ravel() # 1d array, indices of output array
jnew = jnew.ravel() # 1d array, indices of output array
i_back,j_back = np.linalg.inv(T) @ [inew + imin, jnew + jmin]
# create a mask to grab only those rounded (i_back,j_back) indices which make sense
i_back = i_back.round().astype(int)
j_back = j_back.round().astype(int)
inds = (0 <= i_back) & (i_back < n) & (0 <= j_back) & (j_back < m)
# (i_back[inds], j_back[inds]) maps to (inew[inds], jnew[inds])
# the rest stays black
out[inew[inds], jnew[inds], :] = img[i_back[inds], j_back[inds], :]
# check what we've done
plt.imshow(out)
plt.show()
The result, while full of single-pixel inaccuracies, looks good enough:
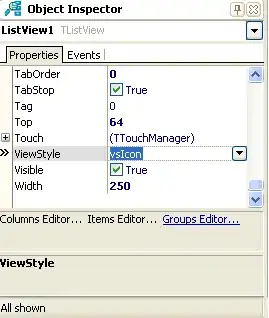