I got an OK fit to a sigmoidal type equation "y = a / (1.0 + exp(-(x-b)/c))" with parameters a = 9.25160014, b = 2.70654566, and c = 0.80626597 yielding RMSE = 0.2661 and R-squared = 0.9924. Here is the Python graphical fitter I used with the scipy differential_evolution genetic algorithm to find initial parameter estimates. The scipy implementation of that module uses the Latin Hypercube algorithm to ensure a thorough search of parameter space, requiring parameter bounds within which to search - in this example those bounds are taken from the data maximum and minimum values.
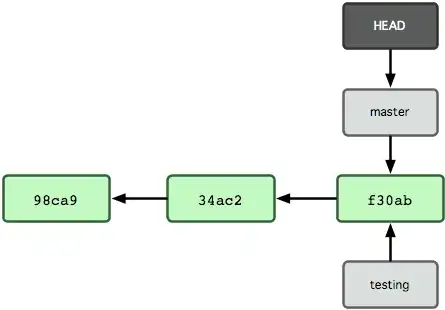
import numpy, scipy, matplotlib
import matplotlib.pyplot as plt
from scipy.optimize import curve_fit
from scipy.optimize import differential_evolution
import warnings
data = [(1, 1), (2, 3), (3, 5), (4, 8), (5, 9), (6, 9), (7, 9)]
# data to float arrays
xData = []
yData = []
for d in data:
xData.append(float(d[0]))
yData.append(float(d[1]))
def func(x, a, b, c): #sigmoidal curve fitting function
return a / (1.0 + numpy.exp(-1.0 * (x - b) / c))
# function for genetic algorithm to minimize (sum of squared error)
def sumOfSquaredError(parameterTuple):
warnings.filterwarnings("ignore") # do not print warnings by genetic algorithm
val = func(xData, *parameterTuple)
return numpy.sum((yData - val) ** 2.0)
def generate_Initial_Parameters():
# min and max used for bounds
maxX = max(xData)
minX = min(xData)
maxY = max(yData)
minY = min(yData)
minXY = min(minX, minY)
maxXY = min(maxX, maxY)
parameterBounds = []
parameterBounds.append([minXY, maxXY]) # search bounds for a
parameterBounds.append([minXY, maxXY]) # search bounds for b
parameterBounds.append([minXY, maxXY]) # search bounds for c
# "seed" the numpy random number generator for repeatable results
result = differential_evolution(sumOfSquaredError, parameterBounds, seed=3)
return result.x
# by default, differential_evolution completes by calling curve_fit() using parameter bounds
geneticParameters = generate_Initial_Parameters()
# now call curve_fit without passing bounds from the genetic algorithm,
# just in case the best fit parameters are aoutside those bounds
fittedParameters, pcov = curve_fit(func, xData, yData, geneticParameters)
print('Fitted parameters:', fittedParameters)
print()
modelPredictions = func(xData, *fittedParameters)
absError = modelPredictions - yData
SE = numpy.square(absError) # squared errors
MSE = numpy.mean(SE) # mean squared errors
RMSE = numpy.sqrt(MSE) # Root Mean Squared Error, RMSE
Rsquared = 1.0 - (numpy.var(absError) / numpy.var(yData))
print()
print('RMSE:', RMSE)
print('R-squared:', Rsquared)
print()
##########################################################
# graphics output section
def ModelAndScatterPlot(graphWidth, graphHeight):
f = plt.figure(figsize=(graphWidth/100.0, graphHeight/100.0), dpi=100)
axes = f.add_subplot(111)
# first the raw data as a scatter plot
axes.plot(xData, yData, 'D')
# create data for the fitted equation plot
xModel = numpy.linspace(min(xData), max(xData))
yModel = func(xModel, *fittedParameters)
# now the model as a line plot
axes.plot(xModel, yModel)
axes.set_xlabel('X Data') # X axis data label
axes.set_ylabel('Y Data') # Y axis data label
plt.show()
plt.close('all') # clean up after using pyplot
graphWidth = 800
graphHeight = 600
ModelAndScatterPlot(graphWidth, graphHeight)