Of course K-Means can be used for color quantization. It's very handy for that.
Let's see an example in Mathematica:
We start with a greyscale (150x150) image:
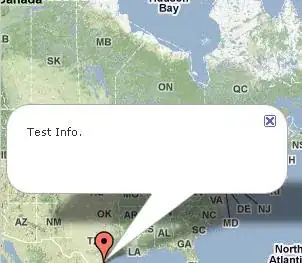
Let's see how many grey levels are there when representing the image in 8 bits:
ac = ImageData[ImageTake[i, All, All], "Byte"];
First@Dimensions@Tally@Flatten@ac
-> 234
Ok. Let's reduce those 234 levels. Our first try will be to let the algorithm alone to determine how many clusters are there with the default configuration:
ic = ClusteringComponents[Image@ac];
First@Dimensions@Tally@Flatten@ic
-> 3
It selects 3 clusters, and the corresponding image is:

Now, if that is ok, or you need more clusters, is up to you.
Let's suppose you decide that a more fine-grained color separation is needed. Let's ask for 6 clusters instead of 3:
ic2 = ClusteringComponents[Image@ac, 6];
Image@ic2 // ImageAdjust
Result:
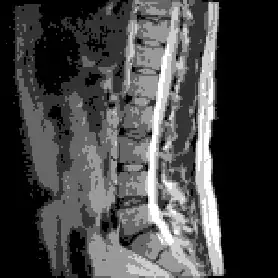
and here are the pixel ranges used in each bin:
Table[{Min@#, Max@#} &@(Take[orig, {#[[1]]}, {#[[2]]}] & /@
Position[clus, n]), {n, 1, 6}]
-> {{0, 11}, {12, 30}, {31, 52}, {53, 85}, {86, 134}, {135, 241}}
and the number of pixels in each bin:
Table[Count[Flatten@clus, i], {i, 6}]
-> {8906, 4400, 4261, 2850, 1363, 720}
So, the answer is YES, and it is straightforward.
Edit
Perhaps this will help you understand what you are doing wrong in your new example.
If I clusterize your color image, and use the cluster number to represent brightness, I get:
That's because the clusters are not being numbered in an ascending brightness order.
But if I calculate the mean brightness value for each cluster, and use it to represent the cluster value, I get:
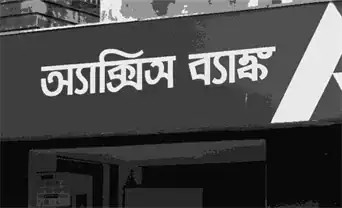
In my previous example, that was not needed, but that was just luck :D (i.e. clusters were found in ascending brightness order)