You can try using geom_histogram
or geom_density
, however it's a little bit complicated as you have to rotate axis for them (while keeping original orientation for geom_line
). I would use geom_violin
(which is a density plot, but mirrored). If you want to get only one sided violin plot you can use custom geom_flat_violin
geom. It was first posted by @David Robinson on his gists.
I used this geom in different answer, however I don't think that it's a duplicate as you need to put it at the end of the plot and combine with different geom.
Final code is:
library(ggplot2)
ggplot(data.frame(x = seq(100), y = rnorm(100))) +
geom_flat_violin(aes(100, y), color = "red", fill = "red", alpha = 0.5, width = 10) +
geom_line(aes(x, y))
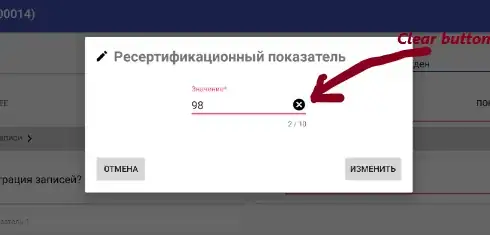
geom_flat_violin
code:
library(dplyr)
"%||%" <- function(a, b) {
if (!is.null(a)) a else b
}
geom_flat_violin <- function(mapping = NULL, data = NULL, stat = "ydensity",
position = "dodge", trim = TRUE, scale = "area",
show.legend = NA, inherit.aes = TRUE, ...) {
layer(
data = data,
mapping = mapping,
stat = stat,
geom = GeomFlatViolin,
position = position,
show.legend = show.legend,
inherit.aes = inherit.aes,
params = list(
trim = trim,
scale = scale,
...
)
)
}
GeomFlatViolin <-
ggproto(
"GeomFlatViolin",
Geom,
setup_data = function(data, params) {
data$width <- data$width %||%
params$width %||% (resolution(data$x, FALSE) * 0.9)
# ymin, ymax, xmin, and xmax define the bounding rectangle for each group
data %>%
dplyr::group_by(.data = ., group) %>%
dplyr::mutate(
.data = .,
ymin = min(y),
ymax = max(y),
xmin = x,
xmax = x + width / 2
)
},
draw_group = function(data, panel_scales, coord)
{
# Find the points for the line to go all the way around
data <- base::transform(data,
xminv = x,
xmaxv = x + violinwidth * (xmax - x))
# Make sure it's sorted properly to draw the outline
newdata <-
base::rbind(
dplyr::arrange(.data = base::transform(data, x = xminv), y),
dplyr::arrange(.data = base::transform(data, x = xmaxv), -y)
)
# Close the polygon: set first and last point the same
# Needed for coord_polar and such
newdata <- rbind(newdata, newdata[1,])
ggplot2:::ggname("geom_flat_violin",
GeomPolygon$draw_panel(newdata, panel_scales, coord))
},
draw_key = draw_key_polygon,
default_aes = ggplot2::aes(
weight = 1,
colour = "grey20",
fill = "white",
size = 0.5,
alpha = NA,
linetype = "solid"
),
required_aes = c("x", "y")
)