The best way to do this probably is via the Stroke Width Transform. This isn't in OpenCV, though it is in a few other libraries and you can find some implementations floating around the internet. The stroke width transform finds the minimum width between the nearest edges for each pixel in the image. See the following figure from the paper:
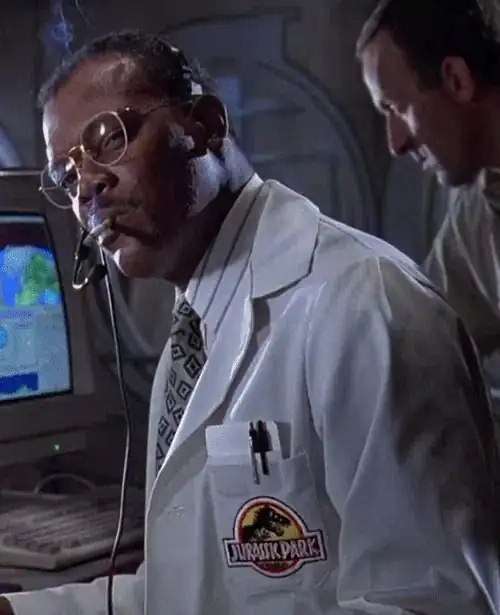
Thresholding this image then tells you where there are edges separated by some small distance. E.g., all the pixels with values < 40, say, are between two edges that are separated by less than 40 pixels.
So, as is probably clear, this is pretty close to the answer that you want. There would be some additional noise here, like you'd also get values that are between the square ridges on the edge of your shapes...which you'd have to filter out or smooth out (contour approximation would be a simple way to clean them up as a preprocessing step, for example).
However, while I do have a prototype SWT programmed, it's not a very good implementation, and I haven't really tested it (and actually forgot about it for a few months.......maybe a year) so I'm not going to put it out right now. But, I do have another idea that is a little simpler and doesn't necessitate reading a research paper.
You have multiple blobs in your input image. Imagine if you had each one separately in its own image, and you grew each blob by however much distance you're willing to put between them. If you grew each blob by say 10 pixels, and they overlap, then they'd be within 20 pixels of each other. However this doesn't give us the full overlap region, just a portion of where the two expanded blobs overlapped. A different, but similar way to measure this is if the blobs grew by 10 pixels, and overlapped, and furthermore overlapped the original blobs before they were expanded, then the two blobs are within 10 pixels of each other. We're going to use this second definition to find nearby blobs.
def find_connection_paths(binimg, distance):
h, w = binimg.shape[:2]
overlap = np.zeros((h, w), dtype=np.int32)
overlap_mask = np.zeros((h, w), dtype=np.uint8)
kernel = cv2.getStructuringElement(cv2.MORPH_ELLIPSE, (distance, distance))
# grows the blobs by `distance` and sums to get overlaps
nlabels, labeled = cv2.connectedComponents(binimg, connectivity=8)
for label in range(1, nlabels):
mask = 255 * np.uint8(labeled == label)
overlap += cv2.dilate(mask, kernel, iterations=1) // 255
overlap = np.uint8(overlap > 1)
# for each overlap, does the overlap touch the original blob?
noverlaps, overlap_components = cv2.connectedComponents(overlap, connectivity=8)
for label in range(1, noverlaps):
mask = 255 * np.uint8(overlap_components == label)
if np.any(cv2.bitwise_and(binimg, mask)):
overlap_mask = cv2.bitwise_or(overlap_mask, mask)
return overlap_mask
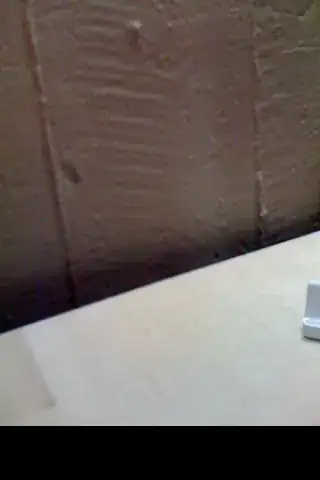
Now the output isn't perfect---when I expanded the blobs, I expanded them outwardly with a circle (the dilation kernel), so the connection areas aren't exactly super clear. However, this was the best way to ensure it'll work on things of any orientation. You could potentially filter this out/clip it down. An easy way to do this would be to get each connecting piece (shown in blue), and repeatedly erode it down a pixel until it doesn't overlap the original blob. Actually OK let's add that:
def find_connection_paths(binimg, distance):
h, w = binimg.shape[:2]
overlap = np.zeros((h, w), dtype=np.int32)
overlap_mask = np.zeros((h, w), dtype=np.uint8)
overlap_min_mask = np.zeros((h, w), dtype=np.uint8)
kernel_dilate = cv2.getStructuringElement(cv2.MORPH_ELLIPSE, (distance, distance))
# grows the blobs by `distance` and sums to get overlaps
nlabels, labeled = cv2.connectedComponents(binimg)
for label in range(1, nlabels):
mask = 255 * np.uint8(labeled == label)
overlap += cv2.dilate(mask, kernel_dilate, iterations=1) // 255
overlap = np.uint8(overlap > 1)
# for each overlap, does the overlap touch the original blob?
noverlaps, overlap_components = cv2.connectedComponents(overlap)
for label in range(1, noverlaps):
mask = 255 * np.uint8(overlap_components == label)
if np.any(cv2.bitwise_and(binimg, mask)):
overlap_mask = cv2.bitwise_or(overlap_mask, mask)
# for each overlap, shrink until it doesn't touch the original blob
kernel_erode = cv2.getStructuringElement(cv2.MORPH_RECT, (3, 3))
noverlaps, overlap_components = cv2.connectedComponents(overlap_mask)
for label in range(1, noverlaps):
mask = 255 * np.uint8(overlap_components == label)
while np.any(cv2.bitwise_and(binimg, mask)):
mask = cv2.erode(mask, kernel_erode, iterations=1)
overlap_min_mask = cv2.bitwise_or(overlap_min_mask, mask)
return overlap_min_mask
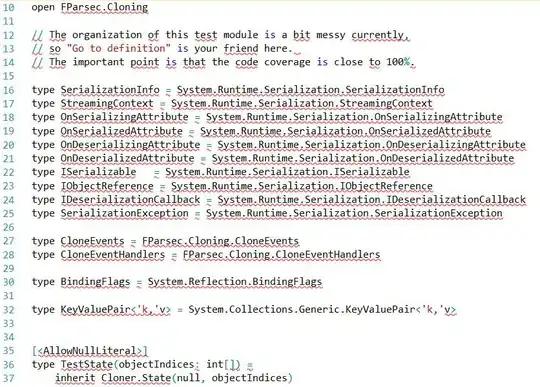
Of course, if you still wanted them to be a little bigger or smaller you could do whatever you like with them, but this looks pretty close to your requested output so I'll leave it there. Also, if you're wondering, I have no idea where the blob on the top right went. I can take another pass at this last piece later. Note that the last two steps could be combined; check if there's overlap, if it is, cool---shrink it down and store it in the mask.