I run the tutorial, the Python code which you use:
python -c 'import base64, json; img = base64.b64encode(open("image.jpg").read()); print json.dumps({"image_bytes": {"b64": img}})' image.jpg &> request.json
Generates a file with the following contents:
{"image_bytes": {"b64": "Base64Text..."}}
Train your model using export saved_model_dir
option.
$ python retrain.py --image_dir ~/flower_photos --
saved_model_dir=/tmp/saved_models/$(date +%s)
Use SavedModel CLI to show the signatures of your SavedModel. Enter the following command to show the signature of inputs/outputs of a TensorFlow SavedModel:
$ saved_model_cli show --dir /tmp/saved_models/1575937119 --all
MetaGraphDef with tag-set: 'serve' contains the following SignatureDefs:
signature_def['serving_default']:
The given SavedModel SignatureDef contains the following input(s):
inputs['image'] tensor_info:
dtype: DT_FLOAT
shape: (-1, 299, 299, 3)
name: Placeholder:0
The given SavedModel SignatureDef contains the following output(s):
outputs['prediction'] tensor_info:
dtype: DT_FLOAT
shape: (-1, 5)
name: final_result:0
Method name is: tensorflow/serving/predict
This means that the model is expecting an input using Tensors, not a b64 encoded image.
You can start a TensorFlow serving to test locally:
tensorflow_model_server --model_base_path=/tmp/saved_models/ --rest_api_port
=9001
URL=http://localhost:9001/v1/models/default:predict
curl -X POST -d @out.json $URL
Where out.json
is a file in JSON format you will get the expected result.
With TF Serving you can use the following code to generate the file:
import numpy as np
import json
from PIL import Image
INPUT_FILE = 'image.jpg'
OUTPUT_FILE = '/tmp/out.json'
def convert_to_json(image_file):
"""Open image, convert it to numpy and create JSON request"""
img = Image.open(image_file).resize((224, 224))
img_array = np.array(img)
predict_request = {"instances": [img_array.tolist()]}
with open(OUTPUT_FILE, 'w') as output_file:
json.dump(predict_request, output_file)
return predict_request
prediction_data = convert_to_json(INPUT_FILE)
You will get:
{
"predictions": [[0.0, 0.0, 1.0, 0.0, 0.0]]
}
If you use AI Platform, you can just send the request using gcloud ai-platform predict
or as an example the UI for testing:
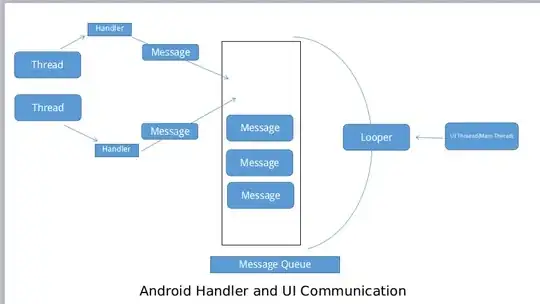
Check: How convert a jpeg image into json file in Google machine learning
for details.
As mentioned by @Puneith, you need to change the Serving function to handle b64
.
This question is similar to GCP ML Engine Prediction failed: Error processing input: Expected float32 got base64