You are making two very basic mistakes:
- Your ultra-simple model (a single-layer network with a single unit) hardly qualifies as a neural network at all, let alone a "deep learning" one (as your question is tagged)
- Similarly, your dataset (just 20 samples) is also ultra-small
It is certainly understood that neural networks need to be of some complexity if they are to solve problems even as "simple" as x*x
; and where they really shine is when fed with large training datasets.
The methodology when trying to solve such function approximations is not to just list the (few possible) inputs and then fed to the model, along with the desired outputs; remember, NNs learn through examples, and not through symbolic reasoning. And the more examples the better. What we usually do in similar cases is to generate a large number of examples, which we subsequently feed to the model for training.
Having said that, here is a rather simple demonstration of a 3-layer neural network in Keras for approximating the function x*x
, using as input 10,000 random numbers generated in [-50, 50]
:
import numpy as np
import keras
from keras.models import Sequential
from keras.layers import Dense
from keras.optimizers import Adam
from keras import regularizers
import matplotlib.pyplot as plt
model = Sequential()
model.add(Dense(8, activation='relu', kernel_regularizer=regularizers.l2(0.001), input_shape = (1,)))
model.add(Dense(8, activation='relu', kernel_regularizer=regularizers.l2(0.001)))
model.add(Dense(1))
model.compile(optimizer=Adam(),loss='mse')
# generate 10,000 random numbers in [-50, 50], along with their squares
x = np.random.random((10000,1))*100-50
y = x**2
# fit the model, keeping 2,000 samples as validation set
hist = model.fit(x,y,validation_split=0.2,
epochs= 15000,
batch_size=256)
# check some predictions:
print(model.predict([4, -4, 11, 20, 8, -5]))
# result:
[[ 16.633354]
[ 15.031291]
[121.26833 ]
[397.78638 ]
[ 65.70035 ]
[ 27.040245]]
Well, not that bad! Remember that NNs are function approximators: we should expect them neither to exactly reproduce the functional relationship nor to "know" that the results for 4
and -4
should be identical.
Let's generate some new random data in [-50,50]
(remember, for all practical purposes, these are unseen data for the model) and plot them, along with the original ones, to get a more general picture:
plt.figure(figsize=(14,5))
plt.subplot(1,2,1)
p = np.random.random((1000,1))*100-50 # new random data in [-50, 50]
plt.plot(p,model.predict(p), '.')
plt.xlabel('x')
plt.ylabel('prediction')
plt.title('Predictions on NEW data in [-50,50]')
plt.subplot(1,2,2)
plt.xlabel('x')
plt.ylabel('y')
plt.plot(x,y,'.')
plt.title('Original data')
Result:
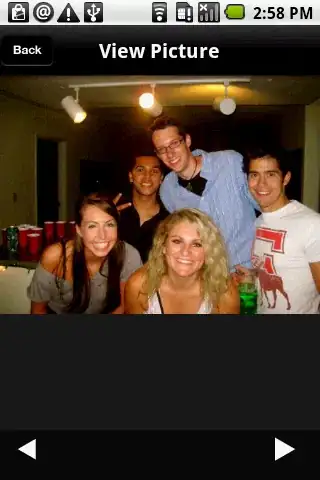
Well, it arguably does look like a good approximation indeed...
You could also take a look at this thread for a sine approximation.
The last thing to keep in mind is that, although we did get a decent approximation even with our relatively simple model, what we should not expect is extrapolation, i.e. good performance outside [-50, 50]
; for details, see my answer in Is deep learning bad at fitting simple non linear functions outside training scope?