You can read the lovely, small JPEG/PNG/JP2 images into memory as a bunch of bytes and hold them there compressed with the same size as they have on disk and then uncompress them from memory when you need them.
First, let's look at the memory required by a 1280x1024 image of RGB888 in memory - its a whopping 3.9MB:
# Decompressed image in memory takes 3.9MB memory
im = np.zeros([1280,1024,3], dtype=np.uint8)
# print(im.nbytes) => 3932160
Now let's look at a JPEG the same size:
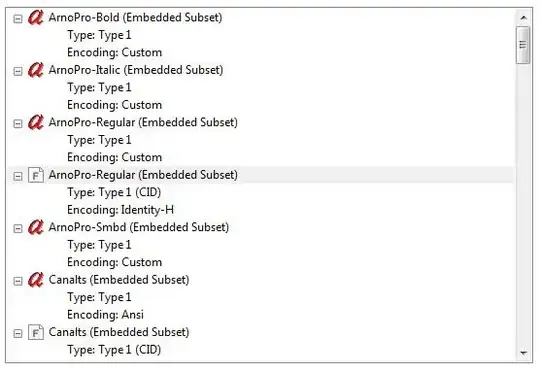
Here it is on disk, with ls -l
:
-rw-r--r--@ 1 mark staff 47276 2 Apr 17:13 image.jpg
And here it is still compressed in memory, also at 47kB or just 1.2% of the size:
# Same image as JPEG takes 47kB of memory
with open('image.jpg','rb') as f:
jpg = f.read()
# print(len(jpg)) => 47276
Now when you want an image, decompress it from memory rather than from disk
# Read with 'imageio'
from io import BytesIO
import imageio
numpyArray = imageio.imread(BytesIO(jpg))
# print(numpyArray.shape) =>(1024, 1280, 3)
# Or, alternatively, and probably faster, read with OpenCV
import cv2
numpyArray = cv2.imdecode(np.frombuffer(jpg,dtype=np.uint8), -1)
# print(numpyArray.shape) =>(1024, 1280, 3)
Another, totally different option that will decode miles faster, but will only reduce the memory footprint by a factor of 3 is to palettise the images. You reduce the number of colours down to less than 256 unique colours, and store a palette with 256 colours. At each pixel location, you then store a single byte which is the index into the palette, rather than 3 bytes of RGB. This will reduce your memory usage from 3.9MB/image to 1.3MB/image. It will not require any decoding. but may result in slight loss of colour fidelity and/or banding - which may or may not be an issue depending on the quality of your camera/images.
That looks something like this:
from PIL import Image
import numpy as np
im = Image.open('image.jpg')
# Make into Numpy array - size is 3.9MB
a = np.array(im)
# Now make a 256 colour palletised version
p = im.convert('P',palette=Image.ADAPTIVE)
# Make into Numpy array - size is now only 1.3MB
a = np.array(p)