Adding to the previous solution. Using reduceByKey is more efficient if your data is really big and you care about parallelism.
If your data is big and want to reduce shuffling effect, as groupBy
can cause shuffling, here is another solution using RDD API
and reduceByKey
that will operate within a partition level:
val mockedRdd = sc.parallelize(Seq(("key1", "a"), ("key1", "b"), ("key2", "a"), ("key2", "a"), ("key2", "a")))
// Converting to PairRDD
val pairRDD = new PairRDDFunctions[String, String](mockedRdd)
// Map and then Reduce
val reducedRDD = pairRDD.mapValues(v => (Set(v), 1)).
reduceByKey((v1, v2) => (v1._1 ++ v2._1, v1._2 + v1._2))
scala> val result = reducedRDD.collect()
`res0: Array[(String, (scala.collection.immutable.Set[String], Int))] = Array((key1,(Set(a, b),2)), (key2,(Set(a),4)))`
Now the final result has the following format (key, set(labels), count)
:
Array((key1,(Set(a, b),2)), (key2,(Set(a),4)))
Now after you collect the results in your driver, you can simply accept counts from Sets that contain only one label:
// Filter our sets with more than one label
scala> result.filter(elm => elm._2._1.size == 1)
res15: Array[(String, (scala.collection.immutable.Set[String], Int))]
= Array((key2,(Set(a),4)))
Analysis using Spark 2.3.2
1) Analysing the (DataFrame API) groupBy Solution
I am not really a Spark Expert, but I will throw my 5 cents here :)
Yes, DataFrame
and SQL Query
go through Catalyst Optimizer, which can possibly optimize a groupBy
.
groupBy
approach proposed using DataFrame API generates the following Physical Plan by running df.explain(true)
== Physical Plan ==
*(3) HashAggregate(keys=[key#14], functions=[count(val#15), count(distinct val#15)], output=[key#14, count#94L])
+- Exchange hashpartitioning(key#14, 200)
+- *(2) HashAggregate(keys=[key#14], functions=[merge_count(val#15), partial_count(distinct val#15)], output=[key#14, count#105L, count#108L])
+- *(2) HashAggregate(keys=[key#14, val#15], functions=[merge_count(val#15)], output=[key#14, val#15, count#105L])
+- Exchange hashpartitioning(key#14, val#15, 200)
+- *(1) HashAggregate(keys=[key#14, val#15], functions=[partial_count(val#15)], output=[key#14, val#15, count#105L])
+- *(1) Project [_1#11 AS key#14, _2#12 AS val#15]
+- *(1) SerializeFromObject [staticinvoke(class org.apache.spark.unsafe.types.UTF8String, StringType, fromString, assertnotnull(input[0, scala.Tuple2, true])._1, true, false) AS _1#11, staticinvoke(class org.apache.spark.unsafe.types.UTF8String, StringType, fromString, assertnotnull(input[0, scala.Tuple2, true])._2, true, false) AS _2#12]
+- Scan ExternalRDDScan[obj#10]
Note that the job has been split into three stages, and has two exchange phases. And it is worth to mention that the second hashpartitioning exchange
used a different set of keys (key, label), which IMO will cause shuffle in this case as partitions hashed with (key, val) will not necessary co-exist with partitions hashed with (key) only.
Here is the plan visualized by Spark UI:
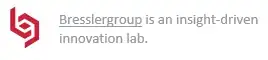
2) Analysing the RDD API Solution
By running reducedRDD.toDebugString
, we will get the following plan:
scala> reducedRDD.toDebugString
res81: String =
(8) ShuffledRDD[44] at reduceByKey at <console>:30 []
+-(8) MapPartitionsRDD[43] at mapValues at <console>:29 []
| ParallelCollectionRDD[42] at parallelize at <console>:30 []
Here is the plan visualized by Spark UI:
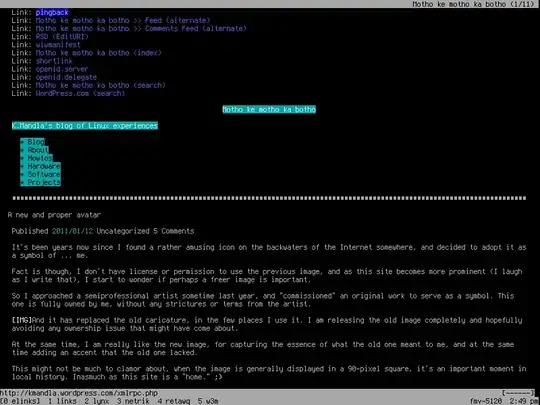
You can clearly see that the RDD approach generated less number of stages and tasks, and also doesn't cause any shuffle until we process the dataset and collect it from the driver side of course. This alone tells us that this approach consumes less resources and time.
Conclusion at the end of the day, how much optimization you want to apply does really depend on your business requirement, and the size of the Data you are dealing with. If you don't have big data, then going by the groupBy approach will be a straight forward option; otherwise, considering (Parallelism, Speed, Shuffling,
& Memory Management) will be important, and most of the time you can accomplish that by analyzing Query Plans and examining your jobs through Spark UI.