Update: as you've now updated your question to make clearer what you're after, let me demonstrate three different ways to plot such data, which all have lots of pros and cons.
The general gist (at least for me!) is that matplotlib
is bad in 3D, especially when it comes to creating publishable figures (again, my personal opinion, your mileage may vary.)
What I did: I've used the original data behind the second image you've posted. In all cases, I used zorder
and added polygon data (in 2D: fill_between()
, in 3D: PolyCollection
) to enhance the "3D effect", i.e. to enable "plotting in front of each other". The code below shows:
plot_2D_a()
uses color to indicate angle, hence keeping the original y-axis; though this technically can now only be used to read out the foremost line plot, it still gives the reader a "feeling" for the y scale.
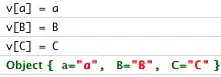
plot_2D_b()
removes unnecessary spines/ticks and rather adds the angle as text labels; this comes closest to the second image you've posted

plot_3D()
uses mplot3d
to make a "3D" plot; while this can now be rotated to analyze the data, it breaks (at least for me) when trying to zoom, yielding cut-off data and/or hidden axes.
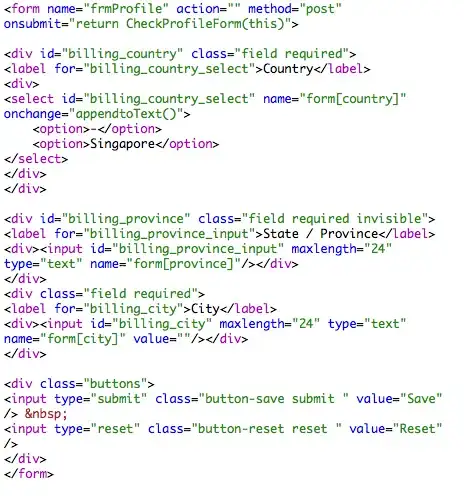
In the end there are many ways to achieve a waterfall plot in matplotlib
, and you have to decide yourself what you're after. Personally, I'd probably us plot_2D_a()
most of the time, since it allows for easy rescaling in more or less "all 3 dimensions" while also keeping proper axes (+colorbar) that allow the reader to get all relevant information once you publish it somewhere as a static image.
Code:
import pandas as pd
import matplotlib as mpl
import matplotlib.pyplot as plt
from mpl_toolkits.mplot3d import Axes3D
from matplotlib.collections import PolyCollection
import numpy as np
def offset(myFig,myAx,n=1,yOff=60):
dx, dy = 0., yOff/myFig.dpi
return myAx.transData + mpl.transforms.ScaledTranslation(dx,n*dy,myFig.dpi_scale_trans)
## taken from
## http://www.gnuplotting.org/data/head_related_impulse_responses.txt
df=pd.read_csv('head_related_impulse_responses.txt',delimiter="\t",skiprows=range(2),header=None)
df=df.transpose()
def plot_2D_a():
""" a 2D plot which uses color to indicate the angle"""
fig,ax=plt.subplots(figsize=(5,6))
sampling=2
thetas=range(0,360)[::sampling]
cmap = mpl.cm.get_cmap('viridis')
norm = mpl.colors.Normalize(vmin=0,vmax=360)
for idx,i in enumerate(thetas):
z_ind=360-idx ## to ensure each plot is "behind" the previous plot
trans=offset(fig,ax,idx,yOff=sampling)
xs=df.loc[0]
ys=df.loc[i+1]
## note that I am using both .plot() and .fill_between(.. edgecolor="None" ..)
# in order to circumvent showing the "edges" of the fill_between
ax.plot(xs,ys,color=cmap(norm(i)),linewidth=1, transform=trans,zorder=z_ind)
## try alpha=0.05 below for some "light shading"
ax.fill_between(xs,ys,-0.5,facecolor="w",alpha=1, edgecolor="None",transform=trans,zorder=z_ind)
cbax = fig.add_axes([0.9, 0.15, 0.02, 0.7]) # x-position, y-position, x-width, y-height
cb1 = mpl.colorbar.ColorbarBase(cbax, cmap=cmap, norm=norm, orientation='vertical')
cb1.set_label('Angle')
## use some sensible viewing limits
ax.set_xlim(-0.2,2.2)
ax.set_ylim(-0.5,5)
ax.set_xlabel('time [ms]')
def plot_2D_b():
""" a 2D plot which removes the y-axis and replaces it with text labels to indicate angles """
fig,ax=plt.subplots(figsize=(5,6))
sampling=2
thetas=range(0,360)[::sampling]
for idx,i in enumerate(thetas):
z_ind=360-idx ## to ensure each plot is "behind" the previous plot
trans=offset(fig,ax,idx,yOff=sampling)
xs=df.loc[0]
ys=df.loc[i+1]
## note that I am using both .plot() and .fill_between(.. edgecolor="None" ..)
# in order to circumvent showing the "edges" of the fill_between
ax.plot(xs,ys,color="k",linewidth=0.5, transform=trans,zorder=z_ind)
ax.fill_between(xs,ys,-0.5,facecolor="w", edgecolor="None",transform=trans,zorder=z_ind)
## for every 10th line plot, add a text denoting the angle.
# There is probably a better way to do this.
if idx%10==0:
textTrans=mpl.transforms.blended_transform_factory(ax.transAxes, trans)
ax.text(-0.05,0,u'{0}º'.format(i),ha="center",va="center",transform=textTrans,clip_on=False)
## use some sensible viewing limits
ax.set_xlim(df.loc[0].min(),df.loc[0].max())
ax.set_ylim(-0.5,5)
## turn off the spines
for side in ["top","right","left"]:
ax.spines[side].set_visible(False)
## and turn off the y axis
ax.set_yticks([])
ax.set_xlabel('time [ms]')
#--------------------------------------------------------------------------------
def plot_3D():
""" a 3D plot of the data, with differently scaled axes"""
fig=plt.figure(figsize=(5,6))
ax= fig.gca(projection='3d')
"""
adjust the axes3d scaling, taken from https://stackoverflow.com/a/30419243/565489
"""
# OUR ONE LINER ADDED HERE: to scale the x, y, z axes
ax.get_proj = lambda: np.dot(Axes3D.get_proj(ax), np.diag([1, 2, 1, 1]))
sampling=2
thetas=range(0,360)[::sampling]
verts = []
count = len(thetas)
for idx,i in enumerate(thetas):
z_ind=360-idx
xs=df.loc[0].values
ys=df.loc[i+1].values
## To have the polygons stretch to the bottom,
# you either have to change the outermost ydata here,
# or append one "x" pixel on each side and then run this.
ys[0] = -0.5
ys[-1]= -0.5
verts.append(list(zip(xs, ys)))
zs=thetas
poly = PolyCollection(verts, facecolors = "w", edgecolors="k",linewidth=0.5 )
ax.add_collection3d(poly, zs=zs, zdir='y')
ax.set_ylim(0,360)
ax.set_xlim(df.loc[0].min(),df.loc[0].max())
ax.set_zlim(-0.5,1)
ax.set_xlabel('time [ms]')
# plot_2D_a()
# plot_2D_b()
plot_3D()
plt.show()