This solution is simple. This algorithm replicates the way that humans would solve the problem. Here are the steps:
- Identify the key
- Mask out a keyhole
- Guess what should fit in the keyhole (using inpaint)
- Compare the key+keyhole to the inpaint image
The best fit will occur where the difference between the inpaint guess and the key+keyhole image are the lowest. This .gif explains
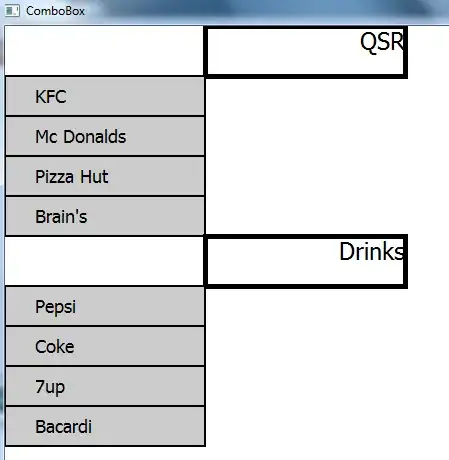
This is the code that I used:
import cv2
import numpy as np
# Read image
img = cv2.imread('/home/stephen/Desktop/capcha.png')
# Get key and mask of key
key = img[567:700, 145:234]
lower, upper = np.array([0,0,0]),np.array([101,255,255])
hsv = cv2.cvtColor(key, cv2.COLOR_BGR2HSV)
key_mask = cv2.inRange(hsv, lower, upper)
key = cv2.bitwise_and(key, key, mask = key_mask)
kernel = np.ones((20,20), np.uint8)
# Create a dilated mask so the key will surely fill keyhole
dilated_key_mask = key_mask.copy()
cv2.morphologyEx(dilated_key_mask, cv2.MORPH_DILATE, kernel)
# https://stackoverflow.com/questions/189943/how-can-i-quantify-difference-between-two-images
from scipy.misc import imread
from scipy.linalg import norm
from scipy import sum, average
def compare_images(img1, img2):
# normalize to compensate for exposure difference, this may be unnecessary
# consider disabling it
img1 = normalize(img1)
img2 = normalize(img2)
# calculate the difference and its norms
diff = img1 - img2 # elementwise for scipy arrays
m_norm = sum(abs(diff)) # Manhattan norm
z_norm = norm(diff.ravel(), 0) # Zero norm
return (m_norm, z_norm)
def to_grayscale(arr):
"If arr is a color image (3D array), convert it to grayscale (2D array)."
if len(arr.shape) == 3:
return average(arr, -1) # average over the last axis (color channels)
else:
return arr
def normalize(arr):
rng = arr.max()-arr.min()
amin = arr.min()
return (arr-amin)*255/rng
# Scan through the image
h, w, _ = img.shape
dh, dw, _ = key.shape
close_diff = h*w
graph = np.zeros((300,600,3), np.uint8)
for row in range(h-dh):
for col in range(w-dw):
# Create a mask of the image with the key missing
img_temp = img.copy()
img_mask = np.zeros((h,w), np.uint8)
img_mask[row:row+dh, col:col+dw] = dilated_key_mask
img_temp = cv2.bitwise_and(img_temp, img_temp, mask = 255-img_mask)
# Inpaint to guess what should be there
inpaint = cv2.inpaint(img_temp,img_mask,3,cv2.INPAINT_TELEA)
# Mask the key of the image
actual = img_temp.copy()
actual[row:row+dh, col:col+dw] += key
# Compare the images
img1 = to_grayscale(inpaint)
img2 = to_grayscale(actual)
_, difference = compare_images(img1, img2)
cv2.imshow('inpaint', inpaint)
cv2.imshow('actual', actual)
cv2.waitKey(1)
if difference < close_diff:
cv2.waitKey()
close_diff = difference
best_fit = row, col
cv2.destroyAllWindows()