One way to remove gradients is to use cv2.medianBlur()
to smooth out the image by taking the median of all pixels under a kernel. Then to extract the letters, you can perform cv2.adaptiveThreshold()
.
The blur removes most of the gradient noise. You can change the kernel size to remove more but it will also remove the details of the letters
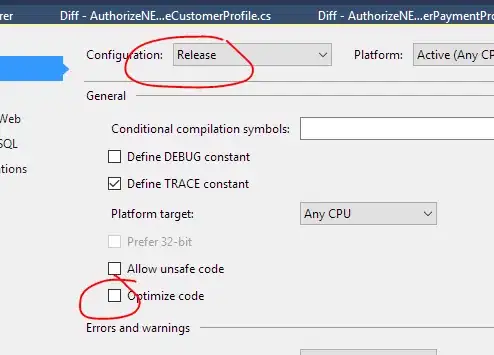
Adaptive threshold the image to extract characters. From your original image, it seems like gradient noise was added onto the the letters c
, x
, and z
to make it blend into the background.
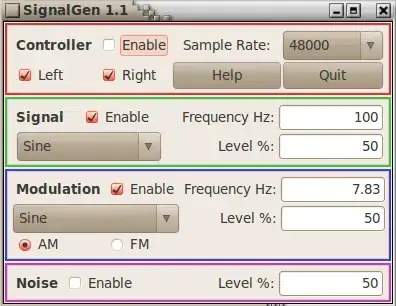
Next we can perform cv2.Canny()
to detect edges and obtain this
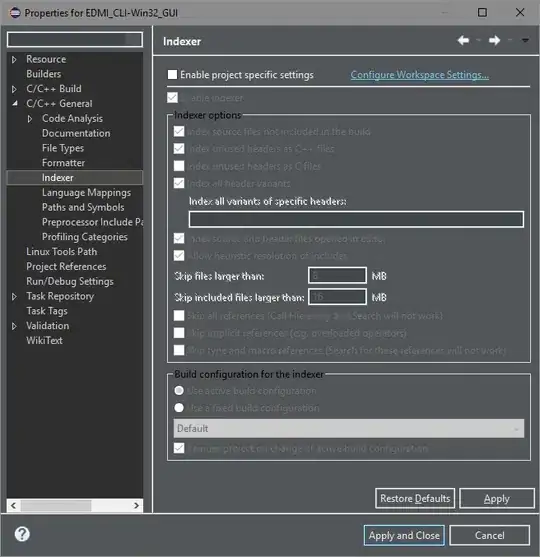
Then we can do morphological opening using cv2.morphologyEx()
to clean up the small noise and enhance details
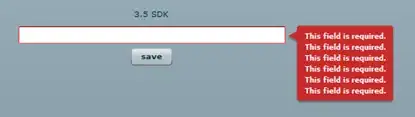
Now we dilate using cv2.dilate()
to obtain a single contour
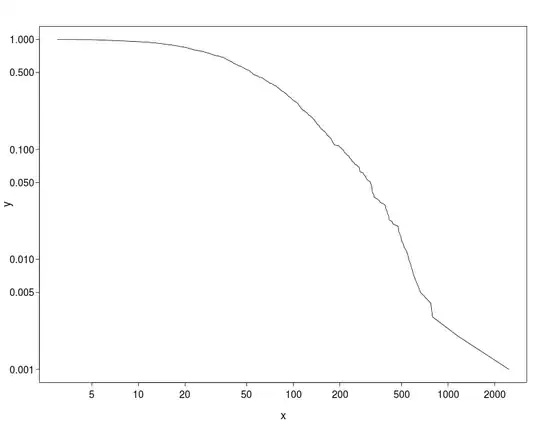
From here, we find contours using cv2.findContours()
. We iterate through each contour and filter using cv2.contourArea()
with a minimum and maximum area to obtain bounding boxes. Depending on your image, you may have to adjust the min/max area filter. Here's the result
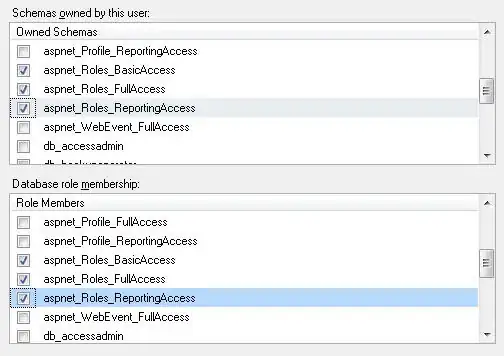
import cv2
import numpy as np
image = cv2.imread('1.png')
blur = cv2.medianBlur(image, 7)
gray = cv2.cvtColor(blur, cv2.COLOR_BGR2GRAY)
thresh = cv2.adaptiveThreshold(gray,255,cv2.ADAPTIVE_THRESH_GAUSSIAN_C, cv2.THRESH_BINARY,11,3)
canny = cv2.Canny(thresh, 120, 255, 1)
kernel = cv2.getStructuringElement(cv2.MORPH_RECT, (5,5))
opening = cv2.morphologyEx(canny, cv2.MORPH_CLOSE, kernel)
dilate = cv2.dilate(opening, kernel, iterations=2)
cnts = cv2.findContours(dilate, cv2.RETR_TREE, cv2.CHAIN_APPROX_SIMPLE)
cnts = cnts[0] if len(cnts) == 2 else cnts[1]
min_area = 500
max_area = 7000
for c in cnts:
area = cv2.contourArea(c)
if area > min_area and area < max_area:
x,y,w,h = cv2.boundingRect(c)
cv2.rectangle(image, (x, y), (x + w, y + h), (36,255,12), 2)
cv2.imshow('blur', blur)
cv2.imshow('thresh', thresh)
cv2.imshow('canny', canny)
cv2.imshow('opening', opening)
cv2.imshow('dilate', dilate)
cv2.imshow('image', image)
cv2.waitKey(0)