my solution is constrained to solely this image, where the card is held up close, and horizontally.
!wget https://i.stack.imgur.com/46VsT.jpg
read in image.
import matplotlib.pyplot as plt
import numpy as np
import imageio
# rgb to gray https://stackoverflow.com/a/51571053/868736
im = imageio.imread('46VsT.jpg')
gray = lambda rgb : np.dot(rgb[... , :3] , [0.299 , 0.587, 0.114])
gray = gray(im)
image = np.array(gray)
plt.imshow(image,cmap='gray')
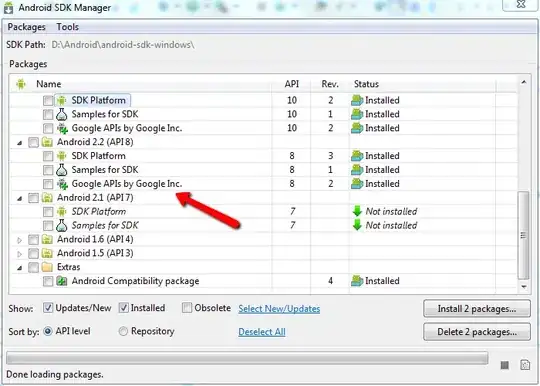
import numpy as np
import skimage
from skimage import feature
from skimage.transform import probabilistic_hough_line
import matplotlib.pyplot as plt
from matplotlib import cm
find horizontal edges with some constraints.
edges = np.abs(skimage.filters.sobel_h(image))
edges = feature.canny(edges,1,100,200)
plt.imshow(edges,cmap='gray')
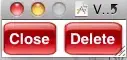
find horizontal lines with more constraints.
# https://scikit-image.org/docs/dev/auto_examples/edges/plot_line_hough_transform.html
lines = probabilistic_hough_line(edges, threshold=1, line_length=200,line_gap=100)
plt.imshow(edges * 0,cmap='gray')
for line in lines:
p0, p1 = line
plt.plot((p0[0], p1[0]), (p0[1], p1[1]),color='red')
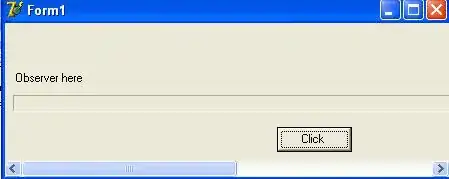
use detected lines to obtain region of interest.
# https://scikit-image.org/docs/dev/auto_examples/edges/plot_convex_hull.html
from skimage.morphology import convex_hull_image
canvas = edges*0
for line in lines:
p0, p1 = line
canvas[p0[1],p0[0]]=1
canvas[p1[1],p1[0]]=1
chull = convex_hull_image(canvas)
plt.imshow(chull,cmap='gray')
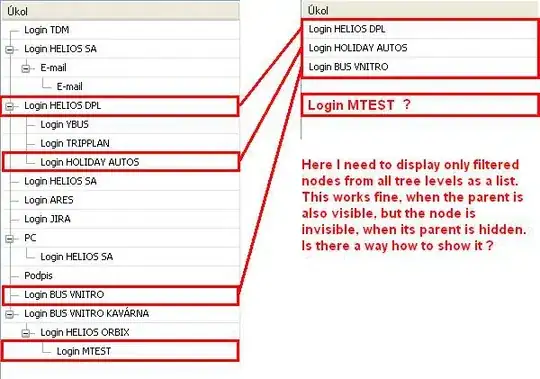
... but why? ;)
I doubt the above solution would actually work "in production"... if you have the resource, I would go for an modified YOLO model, and spend the resource on building a good dataset for training (emphasis on "GOOD" dataset, but you got to define what good is first...), see this video for some inspiration, https://www.youtube.com/watch?v=pnntrewH0xg