You should do vertical and horizontal line detection separately so that you can make them each more specific.
Go through all your lines and compile a list of intersections between horizontal and vertical line combinations
Now you have a list of 2d points that if you draw them should pretty much be on the corners of the boxes. The final step is to collect those points into meaningful sets.
To get those sets, I would start with the point nearest origin (just for the sake of starting somewhere). I would look through all the other points for the closest other point that has a greater x but is withing +-5 (or some configurable range) y of the starting point. Then do the same but in the y direction. You now have the bottom corner of the box. Which you could just complete and start your ocr on, but to be more robust, find the final corner as well.
Once all 4 corners are found, remove all of those points from your intersection array and add however you want to denote box locations into a new array. Rinse and repeat as now a different point will be nearest origin. Without actually testing this, I think it will choke (or need some conditional improvement for missing walls) on the K box but should be pretty generic to variable box shapes and sizes.
Edit 1: In testing, I am finding that it will probably be difficult to separate the close corners of two adjacent boxes. I think a more generic and robust solution would be to after you get collisions, do a point clustering operation at about 1/3 box min side length. This will average corners together with their nearest neighbor. So this will slightly change the strategy as you will need to use every corner twice (box to left and box to right) except for end points.
Wrote up some test code and it is functional, here are the outputs:
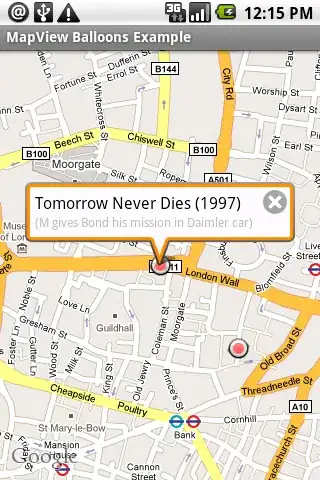
Code, sorry for c++ and not at all optimized, happy friday :)
//CPP libaries
#include <stdio.h>
#include <mutex>
#include <thread>
//Included libraries
//Note: these headers have to be before any opencv due to a namespace collision (could probably be fixed)
#include <opencv2/opencv.hpp>
#include "opencv2/imgproc/imgproc.hpp"
#include "opencv2/highgui/highgui.hpp"
using namespace cv;
// Finds the intersection of two lines, or returns false.
// The lines are defined by (o1, p1) and (o2, p2).
//https://stackoverflow.com/questions/7446126/opencv-2d-line-intersection-helper-function
bool intersection(Point2f o1, Point2f p1, Point2f o2, Point2f p2,
Point2f &r)
{
Point2f x = o2 - o1;
Point2f d1 = p1 - o1;
Point2f d2 = p2 - o2;
float cross = d1.x*d2.y - d1.y*d2.x;
if (abs(cross) < /*EPS*/1e-8)
return false;
double t1 = (x.x * d2.y - x.y * d2.x) / cross;
r = o1 + d1 * t1;
return true;
}
std::vector<Point2f> clusterPts(std::vector<Point2f> inputPts, double clusterRadius_Squared)
{
std::vector<Point2f> outputPts = std::vector<Point2f>();
while(inputPts.size()>0)
{
Point2f clusterCenter = inputPts[0];
while (true)
{
Point2f newClustCenter = Point2f(0, 0);
int averagingCount = 0;
std::vector<int> clusterIndicies = std::vector<int>();
for (int i = 0; i < inputPts.size(); i++)
{
if (clusterRadius_Squared >= pow(inputPts[i].x - clusterCenter.x, 2) + pow(inputPts[i].y - clusterCenter.y, 2))
{
newClustCenter.x += inputPts[i].x;
newClustCenter.y += inputPts[i].y;
averagingCount += 1;
clusterIndicies.push_back(i);
}
}
newClustCenter = newClustCenter / (double)averagingCount;
if (newClustCenter == clusterCenter)
{
//remove all points inside cluster from inputPts, stash cluster center, and break inner while loop
std::vector<Point2f> remainingPts = std::vector<Point2f>();
for (int i = 0; i < inputPts.size(); i++)
{
if (std::find(clusterIndicies.begin(), clusterIndicies.end(), i) == clusterIndicies.end())
{
remainingPts.push_back(inputPts[i]);
}
}
inputPts = remainingPts;
outputPts.push_back(clusterCenter);
break;
}
else
{
clusterCenter = newClustCenter;
}
}
}
return outputPts;
}
std::vector<Rect> findBoxes(std::vector<Point2f> corners, bool shrinkBoxes = false, int boxSideLength_Guess = 50)
{
std::vector<Rect> outBoxes = std::vector<Rect>();
int approxBoxSize = 1000 * boxSideLength_Guess;
while (corners.size()>4)
{
//find point above or below (these points will be removed from array after used)
int secondPtIndex = -1;
for (int i = 1; i < corners.size(); i++)
{
if (abs(corners[i].x - corners[0].x) < boxSideLength_Guess / 2.0)
{
secondPtIndex = i;
break;
}
}
if (secondPtIndex == -1)
{
std::cout << "bad box point tossed" << std::endl;
corners.erase(corners.begin() + 0);
continue;
}
//now search for closest same level point on either side
int thirdIndexRight = -1;
int thirdIndexLeft = -1;
double minDistRight = approxBoxSize;
double minDistLeft = -approxBoxSize;
for (int i = 2; i < corners.size(); i++)
{
if (abs(corners[i].y - corners[secondPtIndex].y) < boxSideLength_Guess / 2.0)
{
double dist = corners[i].x - corners[secondPtIndex].x;
if (dist < 0 && dist > minDistLeft) //check left
{
minDistLeft = dist;
thirdIndexLeft = i;
}
else if(dist > 0 && dist < minDistRight) //check right
{
minDistRight = dist;
thirdIndexRight = i;
}
}
}
if (thirdIndexLeft != -1) { approxBoxSize = 1.5 * abs(minDistLeft); }
if (thirdIndexRight != -1) { approxBoxSize = 1.5 * minDistRight; }
int fourthIndexRight = -1;
int fourthIndexLeft = -1;
for (int i = 1; i < corners.size(); i++)
{
if (i == thirdIndexLeft || i == thirdIndexRight) { continue; }
if (thirdIndexLeft != -1 && abs(corners[i].x - corners[thirdIndexLeft].x) < boxSideLength_Guess / 2.0)
{ fourthIndexLeft = i; }
if (thirdIndexRight != -1 && abs(corners[i].x - corners[thirdIndexRight].x) < boxSideLength_Guess / 2.0)
{ fourthIndexRight = i; }
}
if (!shrinkBoxes)
{
if (fourthIndexRight != -1)
{
outBoxes.push_back(Rect(corners[0], corners[thirdIndexRight]));
}
if (fourthIndexLeft != -1)
{
outBoxes.push_back(Rect(corners[0], corners[thirdIndexLeft]));
}
}
else
{
if (fourthIndexRight != -1)
{
outBoxes.push_back(Rect(corners[0] * 0.90 + corners[thirdIndexRight] *0.10, corners[0] * 0.10 + corners[thirdIndexRight] * 0.90));
}
if (fourthIndexLeft != -1)
{
outBoxes.push_back(Rect(corners[0] * 0.90 + corners[thirdIndexLeft] * 0.10, corners[0] * 0.10 + corners[thirdIndexLeft] * 0.90));
}
}
corners.erase(corners.begin() + secondPtIndex);
corners.erase(corners.begin() + 0);
}
std::cout << approxBoxSize << std::endl;
return outBoxes;
}
int main(int argc, char** argv)
{
Mat image = imread("../../resources/images/boxPic.png", CV_LOAD_IMAGE_GRAYSCALE);
imshow("source", image);
//namedWindow("Display window", WINDOW_AUTOSIZE);// Create a window for display.
//imshow("Display window", image); // Show our image inside it.
Mat edges, lineOverlay, cornerOverlay, finalBoxes;
Canny(image, edges, 50, 200, 3);
//edges = image;
//cvtColor(image, edges, COLOR_GRAY2BGR);
cvtColor(image, lineOverlay, COLOR_GRAY2BGR);
cvtColor(image, cornerOverlay, COLOR_GRAY2BGR);
cvtColor(image, finalBoxes, COLOR_GRAY2BGR);
std::cout << image.cols << " , "<<image.rows << std::endl;
std::vector<Vec2f> linesHorizontal;
std::vector<Point> ptsLH;
HoughLines(edges, linesHorizontal, 5, CV_PI / 180, 2 * edges.cols * 0.6, 0.0,0.0, CV_PI / 4, 3 * CV_PI / 4);
std::vector<Vec2f> linesVertical;
std::vector<Point> ptsLV;
HoughLines(edges, linesVertical, 5, CV_PI / 180, 2 * edges.rows * 0.6,0,0,-CV_PI/32,CV_PI/32);
for (size_t i = 0; i < linesHorizontal.size(); i++)
{
float rho = linesHorizontal[i][0], theta = linesHorizontal[i][1];
Point pt1, pt2;
double a = cos(theta), b = sin(theta);
double x0 = a * rho, y0 = b * rho;
pt1.x = cvRound(x0 + 1000 * (-b));
pt1.y = cvRound(y0 + 1000 * (a));
pt2.x = cvRound(x0 - 1000 * (-b));
pt2.y = cvRound(y0 - 1000 * (a));
ptsLH.push_back(pt1);
ptsLH.push_back(pt2);
line(lineOverlay, pt1, pt2, Scalar(0, 0, 255), 1, LINE_AA);
}
for (size_t i = 0; i < linesVertical.size(); i++)
{
float rho = linesVertical[i][0], theta = linesVertical[i][1];
Point pt1, pt2;
double a = cos(theta), b = sin(theta);
double x0 = a * rho, y0 = b * rho;
pt1.x = cvRound(x0 + 1000 * (-b));
pt1.y = cvRound(y0 + 1000 * (a));
pt2.x = cvRound(x0 - 1000 * (-b));
pt2.y = cvRound(y0 - 1000 * (a));
ptsLV.push_back(pt1);
ptsLV.push_back(pt2);
line(lineOverlay, pt1, pt2, Scalar(0, 255, 0), 1, LINE_AA);
}
imshow("edged", edges);
imshow("detected lines", lineOverlay);
//look for collisions
std::vector<Point2f> xPts;
for (size_t i = 0; i < linesHorizontal.size(); i++)
{
for (size_t ii = 0; ii < linesVertical.size(); ii++)
{
Point2f xPt;
bool intersectionExists = intersection(ptsLH[2 * i], ptsLH[2 * i + 1], ptsLV[2 * ii], ptsLV[2 * ii + 1], xPt);
if (intersectionExists)
{
xPts.push_back(xPt);
}
}
}
waitKey(1000);
std::vector<Point2f> boxCorners = clusterPts(xPts, 25*25);
for (int i = 0; i < boxCorners.size(); i++)
{
circle(cornerOverlay, boxCorners[i], 5, Scalar(0, 255, 0), 2);
}
imshow("detected corners", cornerOverlay);
//group make boxes for groups of points
std::vector<Rect> ocrBoxes = findBoxes(boxCorners,true);
for (int i = 0; i < ocrBoxes.size(); i++)
{
if (i % 3 == 0) { rectangle(finalBoxes, ocrBoxes[i], Scalar(255, 0, 0), 2); }
else if(i % 3 == 1) { rectangle(finalBoxes, ocrBoxes[i], Scalar(0, 255, 0), 2); }
else if (i % 3 == 2) { rectangle(finalBoxes, ocrBoxes[i], Scalar(0, 0, 255), 2); }
}
imshow("detected boxes", finalBoxes);
waitKey(0); // Wait for a keystroke in the window
return 0;
}