Here's a simple approach using OpenCV Python
- Convert image to grayscale
- Perform canny edge detection
- Perform morphological transformations
- Find contours and sort by largest contour area
- Extract ROI
Canny edge detection (left) then perform morphological transformations to smooth image (right)
canny = cv2.Canny(gray, 5, 150, 1)
kernel = cv2.getStructuringElement(cv2.MORPH_RECT, (5,5))
close = cv2.morphologyEx(canny, cv2.MORPH_CLOSE, kernel, iterations=2)
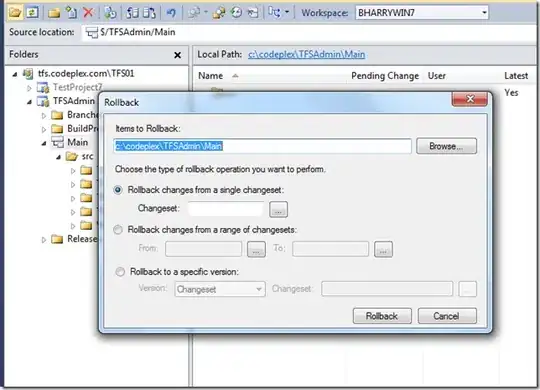
Now we find contours and sort by the largest contour area. The idea is that the largest contour will be the main image. Even if the image is not centered, it should be the largest area. An additional filtering step could be to add in aspect ratio to ensure that the contour is a square/rectangle.
cnts = cv2.findContours(close, cv2.RETR_EXTERNAL, cv2.CHAIN_APPROX_SIMPLE)
cnts = cnts[0] if len(cnts) == 2 else cnts[1]
cnts = sorted(cnts, key = cv2.contourArea, reverse = True)[:10]
for c in cnts:
x,y,w,h = cv2.boundingRect(c)
cv2.rectangle(image, (x, y), (x + w, y + h), (36,255,12), 2)
ROI = original[y:y+h, x:x+w]
break
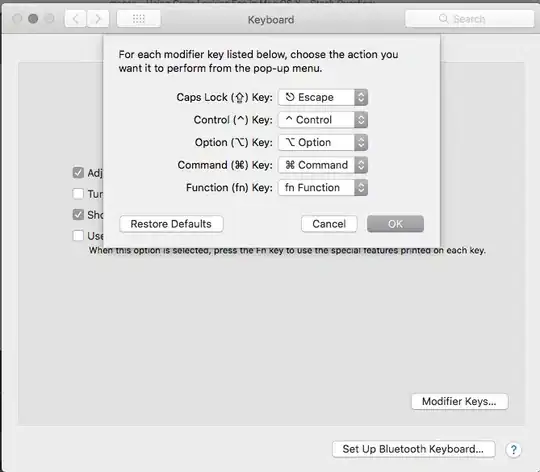
Finally to extract the ROI, we can use Numpy slicing
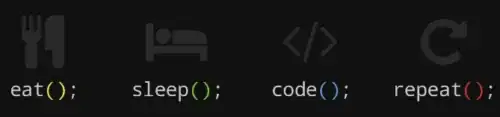
Code
import cv2
image = cv2.imread('2.jpg')
original = image.copy()
gray = cv2.cvtColor(image, cv2.COLOR_BGR2GRAY)
canny = cv2.Canny(gray, 5, 150, 1)
kernel = cv2.getStructuringElement(cv2.MORPH_RECT, (5,5))
close = cv2.morphologyEx(canny, cv2.MORPH_CLOSE, kernel, iterations=2)
cnts = cv2.findContours(close, cv2.RETR_EXTERNAL, cv2.CHAIN_APPROX_SIMPLE)
cnts = cnts[0] if len(cnts) == 2 else cnts[1]
cnts = sorted(cnts, key = cv2.contourArea, reverse = True)[:10]
for c in cnts:
x,y,w,h = cv2.boundingRect(c)
cv2.rectangle(image, (x, y), (x + w, y + h), (36,255,12), 2)
ROI = original[y:y+h, x:x+w]
break
cv2.imshow('canny', canny)
cv2.imshow('close', close)
cv2.imshow('image', image)
cv2.imshow('ROI', ROI)
cv2.imwrite('canny.png', canny)
cv2.imwrite('close.png', close)
cv2.imwrite('ROI.png', ROI)
cv2.imwrite('image.png', image)
cv2.waitKey(0)