The right way to do it is to simply set the alpha
value to 0.0
. In the OpenCV documentation: alpha=0
means that the rectified images are zoomed and shifted so that only valid pixels are visible (no black areas after rectification).
Alternatively, you can implement your own algorithm for this, something like:
Imagine that I have these two images, after rectifying (the green lines are just to visually check that the rectification is correct):
R1, R2, P1, P2, Q, roi1, roi2 = \
cv2.stereoRectify(cameraMatrix1=k_1,
distCoeffs1=dist_coeff,
cameraMatrix2=k_2,
distCoeffs2=dist_coeff,
imageSize=(width, height),
R=r_stereo,
T=t_stereo,
flags=cv2.CALIB_ZERO_DISPARITY,
alpha=1.0
)
map1x, map1y = cv2.initUndistortRectifyMap(
cameraMatrix=k_1,
distCoeffs=dist_coeff,
R=R1,
newCameraMatrix=P1,
size=(width, height),
m1type=cv2.CV_32FC1)
map2x, map2y = cv2.initUndistortRectifyMap(
cameraMatrix=k_2,
distCoeffs=dist_coeff,
R=R2,
newCameraMatrix=P2,
size=(width, height),
m1type=cv2.CV_32FC1)
im_1_rect = cv2.remap(im_1, map1x, map1y, cv2.INTER_LINEAR)
im_2_rect = cv2.remap(im_2, map2x, map2y, cv2.INTER_LINEAR)
result = np.hstack((im_1_rect, im_2_rect))
for tmp_col in range(20, height, 30):
result = cv2.line(result, (0, tmp_col), (int(2.0 * width), tmp_col), (0, 255, 0), 1)
cv2.imshow("rectified image", result)
cv2.waitKey(0)
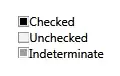
The trick is to project the points in the border of the image to the rectified image and then check the projected u
and v
coordinates. For example, here I will show you how we can project the corners of the original image to the rectified images (painted in red):
pts = np.array([[[0, 0]], [[width - 1, 0]], [[0, height - 1]], [[width - 1, height - 1]]], dtype=np.float64)
pts_transformed_l = cv2.undistortPoints(pts, k_1, dist_coeff, R=R1, P=P1)
pts_transformed_r = cv2.undistortPoints(pts, k_2, dist_coeff, R=R2, P=P2)
for pt in pts_transformed_l:
u, v = pt[0]
result = cv2.circle(result, (int(round(u)), int(round(v))), 3, (0, 0, 255), -1)
for pt in pts_transformed_r:
u, v = pt[0]
u += 640
result = cv2.circle(result, (int(round(u)), int(round(v))), 3, (0, 0, 255), -1)
cv2.imshow("rectified image with corners", result)
cv2.waitKey(0)
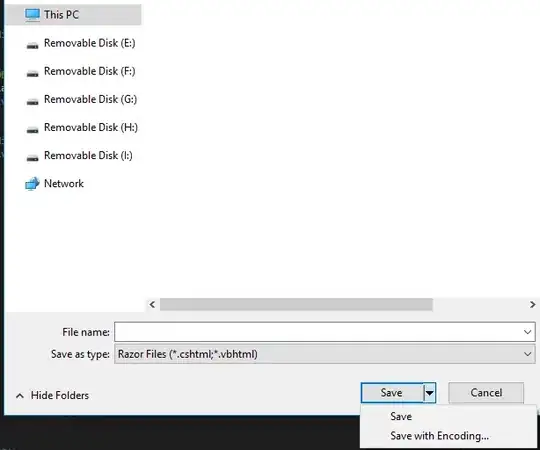
Let's start with the cropping the height. To do that, we warp the top and bottom points to each of the rectified images. We want the largest v
for the top part and the smallest v
for the bottom part. Note that now we need to consider all the points of the top (0, 0), (0, 1), ... (width-1, 0)
since the rectified images have generally curved borders. In other words, we basically want to find the largest v
in the red points and the smallest v
in the points in blue in this image:

""" crop in the v direction """
pts_top_list = []
pts_bot_list = []
for i in range(width):
pt_tmp = [[i, 0]]
pts_top_list.append(pt_tmp)
pt_tmp = [[i, height]]
pts_bot_list.append(pt_tmp)
pts_top = np.asarray(pts_top_list, dtype=np.float64)
pts_bot = np.asarray(pts_bot_list, dtype=np.float64)
# top part - larger v
v_top = 0
## rectified image 1
pts_transformed_l = cv2.undistortPoints(pts_top, k_1, dist_coeff, R=R1, P=P1)
for pt in pts_transformed_l:
_, v = pt[0]
if math.ceil(v) > v_top:
v_top = math.ceil(v)
## rectified image 2
pts_transformed_r = cv2.undistortPoints(pts_top, k_2, dist_coeff, R=R2, P=P2)
for pt in pts_transformed_r:
_, v = pt[0]
if math.ceil(v) > v_top:
v_top = math.ceil(v)
# bottom part - smaller v
v_bot = height
## rectified image 1
pts_transformed_l = cv2.undistortPoints(pts_bot, k_1, dist_coeff, R=R1, P=P1)
for pt in pts_transformed_l:
_, v = pt[0]
if int(v) < v_bot:
v_bot = int(v)
## rectified image 2
pts_transformed_r = cv2.undistortPoints(pts_bot, k_2, dist_coeff, R=R2, P=P2)
for pt in pts_transformed_r:
_, v = pt[0]
if int(v) < v_bot:
v_bot = int(v)
result_cropped_v = result[v_top:v_bot, :]
cv2.imshow("rectified cropped v", result_cropped_v)
cv2.waitKey(0)

You can apply the same in the u
direction. Just be careful that if you crop in u
and you are estimating disparity, you will need to take that into consideration before estimating depth!
""" crop in the u direction (for both images) """
pts_left_list = []
pts_rght_list = []
for i in range(width):
pt_tmp = [[0, i]]
pts_left_list.append(pt_tmp)
pt_tmp = [[width, i]]
pts_rght_list.append(pt_tmp)
pts_left = np.asarray(pts_left_list, dtype=np.float64)
pts_rght = np.asarray(pts_rght_list, dtype=np.float64)
# rectified image 1
## left part - larger u
u_left_1 = 0
pts_transformed_l = cv2.undistortPoints(pts_left, k_1, dist_coeff, R=R1, P=P1)
for pt in pts_transformed_l:
u, _ = pt[0]
if math.ceil(u) > u_left_1:
u_left_1 = math.ceil(u)
## right part - smaller u
u_right_1 = width
pts_transformed_r = cv2.undistortPoints(pts_rght, k_1, dist_coeff, R=R1, P=P1)
for pt in pts_transformed_r:
u, _ = pt[0]
if int(u) < u_right_1:
u_right_1 = int(u)
# rectified image 2
## left part - larger u
u_left_2 = 0
pts_transformed_l = cv2.undistortPoints(pts_left, k_2, dist_coeff, R=R2, P=P2)
for pt in pts_transformed_l:
u, _ = pt[0]
if math.ceil(u) > u_left_2:
u_left_2 = math.ceil(u)
## right part - smaller u
u_right_2 = width
pts_transformed_r = cv2.undistortPoints(pts_rght, k_2, dist_coeff, R=R2, P=P2)
for pt in pts_transformed_r:
u, _ = pt[0]
if int(u) < u_right_2:
u_right_2 = int(u)
im_1_rect_cropped = im_1_rect[v_top:v_bot, u_left_1:u_right_1]
im_2_rect_cropped = im_2_rect[v_top:v_bot, u_left_2:u_right_2]
result_cropped = np.hstack((im_1_rect_cropped, im_2_rect_cropped))
for tmp_col in range(20, height, 30):
result = cv2.line(result_cropped, (0, tmp_col), (int(2.0 * width), tmp_col), (0, 255, 0), 1)
cv2.imshow("rectified image cropped", result_cropped)
cv2.waitKey(0)
