How to define overlapping rectangles?
We need a way to define when two rectangles overlap. We can use the &
intersection operator to find the intersection of the two rectangles, and check that it's not empty:
bool overlap(const cv::Rect& lhs, const cv::Rect& rhs) {
return (lhs & rhs).area() > 0;
}
If we want to ignore small intersections, we can use a threshold over the intersection area:
bool overlap(const cv::Rect& lhs, const cv::Rect& rhs, int th) {
return (lhs & rhs).area() > th;
}
But now the threshold depends on the dimensions of the rectangles. We can use the "Intersection over Union" metric (IoU) which is in the range [0, 1], and apply a threshold in that interval.
bool overlap(const cv::Rect& lhs, const cv::Rect& rhs, double th) {
double i = static_cast<double>((lhs & rhs).area());
double u = static_cast<double>((lhs | rhs).area());
double iou = i / u;
return iou > th;
}
This works well in general, but may show unexpected results if the two rectangles have a very different size. Another approach could be to check if the first rectangle intersects with the second one for most of its area, and vice versa:
bool overlap(const cv::Rect& lhs, const cv::Rect& rhs, double th) {
double i = static_cast<double>((lhs & rhs).area());
double ratio_intersection_over_lhs_area = i / static_cast<double>(lhs.area());
double ratio_intersection_over_rhs_area = i / static_cast<double>(rhs.area());
return (ratio_intersection_over_lhs_area > th) || (ratio_intersection_over_rhs_area > th);
}
Ok, now we have a few ways to define when two rectangles overlap. Pick one.
How to find overlapping rectangles?
We can cluster the rectangles with cv::partition
with a predicate that puts overlapping rectangles in the same cluster. This will put in the same cluster even two rectangles that do not directly overlap each other, but are linked by one or more overlapping rectangles. The output of this function is a vector of cluster, where each cluster consists in a vector of rectangles:
std::vector<std::vector<cv::Rect>> cluster_rects(const std::vector<cv::Rect>& rects, const double th)
{
std::vector<int> labels;
int n_labels = cv::partition(rects, labels, [th](const cv::Rect& lhs, const cv::Rect& rhs) {
double i = static_cast<double>((lhs & rhs).area());
double ratio_intersection_over_lhs_area = i / static_cast<double>(lhs.area());
double ratio_intersection_over_rhs_area = i / static_cast<double>(rhs.area());
return (ratio_intersection_over_lhs_area > th) || (ratio_intersection_over_rhs_area > th);
});
std::vector<std::vector<cv::Rect>> clusters(n_labels);
for (size_t i = 0; i < rects.size(); ++i) {
clusters[labels[i]].push_back(rects[i]);
}
return clusters;
}
For example, from the rectangles in this image:
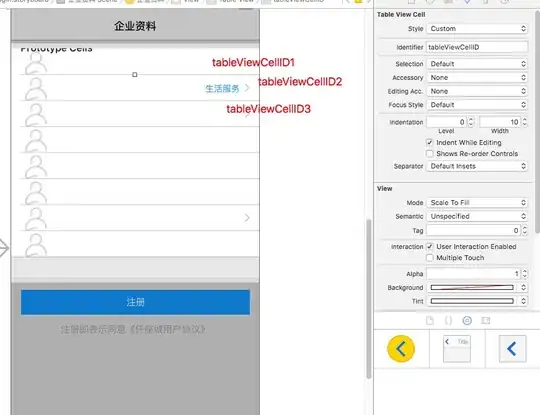
we obtain these clusters (with a threshold of 0.2
). Note that:
- in the top left cluster the three rectangles do not overlap with each other
- the rectangle on the top right is on its own cluster, because it doesn't intersect enough with the other rectangles.
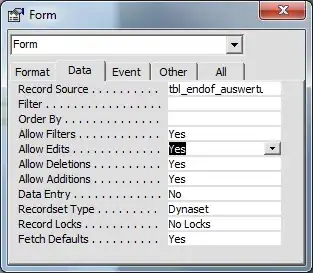
How to find a rectangle that represents a cluster?
Well, that's really application dependent. It can be the union of all rectangles:
cv::Rect union_of_rects(const std::vector<cv::Rect>& cluster)
{
cv::Rect one;
if (!cluster.empty())
{
one = cluster[0];
for (const auto& r : cluster) { one |= r; }
}
return one;
}
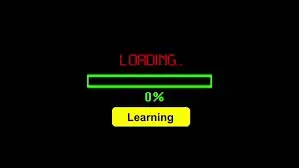
Or it can be the maximum inscribed rectangle (code below):
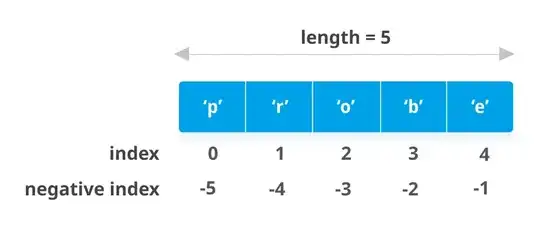
Or something else. For example, if you have a score associated with each rectangle (e.g. it's a detection with a confidence) you can sort each cluster by score and take only the first one. This is a an example of non-maxima-suppression (NMA) and you keep only the highest score rectangle for each cluster (Not showed in this answer).
Pick one.
Below the working code I used for creating these images. Please play with it :)
#include <opencv2/opencv.hpp>
std::vector<cv::Rect> create_some_rects()
{
std::vector<cv::Rect> rects
{
{20, 20, 20, 40},
{30, 40, 40, 40},
{50, 46, 30, 40},
{100, 120, 30, 40},
{110, 130, 36, 20},
{104, 124, 50, 30},
{200, 80, 40, 50},
{220, 90, 50, 30},
{240, 84, 30, 70},
{260, 60, 20, 30},
};
return rects;
}
void draw_rects(cv::Mat3b& img, const std::vector<cv::Rect>& rects)
{
for (const auto& r : rects) {
cv::Scalar random_color(rand() & 255, rand() & 255, rand() & 255);
cv::rectangle(img, r, random_color);
}
}
void draw_rects(cv::Mat3b& img, const std::vector<cv::Rect>& rects, const cv::Scalar& color)
{
for (const auto& r : rects) {
cv::rectangle(img, r, color);
}
}
void draw_clusters(cv::Mat3b& img, const std::vector<std::vector<cv::Rect>>& clusters)
{
for (const auto& cluster : clusters) {
cv::Scalar random_color(rand() & 255, rand() & 255, rand() & 255);
draw_rects(img, cluster, random_color);
}
}
std::vector<std::vector<cv::Rect>> cluster_rects(const std::vector<cv::Rect>& rects, const double th)
{
std::vector<int> labels;
int n_labels = cv::partition(rects, labels, [th](const cv::Rect& lhs, const cv::Rect& rhs) {
double i = static_cast<double>((lhs & rhs).area());
double ratio_intersection_over_lhs_area = i / static_cast<double>(lhs.area());
double ratio_intersection_over_rhs_area = i / static_cast<double>(rhs.area());
return (ratio_intersection_over_lhs_area > th) || (ratio_intersection_over_rhs_area > th);
});
std::vector<std::vector<cv::Rect>> clusters(n_labels);
for (size_t i = 0; i < rects.size(); ++i) {
clusters[labels[i]].push_back(rects[i]);
}
return clusters;
}
cv::Rect union_of_rects(const std::vector<cv::Rect>& cluster)
{
cv::Rect one;
if (!cluster.empty())
{
one = cluster[0];
for (const auto& r : cluster) { one |= r; }
}
return one;
}
// https://stackoverflow.com/a/30418912/5008845
// https://stackoverflow.com/a/34905215/5008845
cv::Rect findMaxRect(const cv::Mat1b& src)
{
cv::Mat1f W(src.rows, src.cols, float(0));
cv::Mat1f H(src.rows, src.cols, float(0));
cv::Rect maxRect(0, 0, 0, 0);
float maxArea = 0.f;
for (int r = 0; r < src.rows; ++r)
{
for (int c = 0; c < src.cols; ++c)
{
if (src(r, c) == 0)
{
H(r, c) = 1.f + ((r > 0) ? H(r - 1, c) : 0);
W(r, c) = 1.f + ((c > 0) ? W(r, c - 1) : 0);
}
float minw = W(r, c);
for (int h = 0; h < H(r, c); ++h)
{
minw = std::min(minw, W(r - h, c));
float area = (h + 1) * minw;
if (area > maxArea)
{
maxArea = area;
maxRect = cv::Rect(cv::Point(c - minw + 1, r - h), cv::Point(c + 1, r + 1));
}
}
}
}
return maxRect;
}
cv::Rect largest_inscribed_of_rects(const std::vector<cv::Rect>& cluster)
{
cv::Rect roi = union_of_rects(cluster);
cv::Mat1b mask(roi.height, roi.width, uchar(255));
for (const auto& r : cluster) {
cv::rectangle(mask, r - roi.tl(), cv::Scalar(0), cv::FILLED);
}
cv::Rect largest_rect = findMaxRect(mask);
largest_rect += roi.tl();
return largest_rect;
}
std::vector<cv::Rect> find_one_for_cluster(const std::vector<std::vector<cv::Rect>>& clusters)
{
std::vector<cv::Rect> one_for_cluster;
for (const auto& cluster : clusters) {
//cv::Rect one = union_of_rects(cluster);
cv::Rect one = largest_inscribed_of_rects(cluster);
one_for_cluster.push_back(one);
}
return one_for_cluster;
}
int main()
{
cv::Mat3b img(200, 300, cv::Vec3b(0, 0, 0));
std::vector<cv::Rect> rects = create_some_rects();
cv::Mat3b initial_rects_img = img.clone();
draw_rects(initial_rects_img, rects, cv::Scalar(127, 127, 127));
std::vector<std::vector<cv::Rect>> clusters = cluster_rects(rects, 0.2);
cv::Mat3b clustered_rects_img = initial_rects_img.clone();
draw_clusters(clustered_rects_img, clusters);
std::vector<cv::Rect> single_rects = find_one_for_cluster(clusters);
cv::Mat3b single_rects_img = initial_rects_img.clone();
draw_rects(single_rects_img, single_rects);
return 0;
}