Here's an idea. We break this problem up into several steps:
Determine average rectangular contour area. We threshold then find contours and filter using the bounding rectangle area of the contour. The reason we do this is because of the observation that any typical character will only be so big whereas large noise will span a larger rectangular area. We then determine the average area.
Remove large outlier contours. We iterate through contours again and remove the large contours if they are 5x
larger than the average contour area by filling in the contour. Instead of using a fixed threshold area, we use this dynamic threshold for more robustness.
Dilate with a vertical kernel to connect characters. The idea is take advantage of the observation that characters are aligned in columns. By dilating with a vertical kernel we connect the text together so noise will not be included in this combined contour.
Remove small noise. Now that the text to keep is connected, we find contours and remove any contours smaller than 4x
the average contour area.
Bitwise-and to reconstruct image. Since we only have desired contours to keep on our mask, we bitwise-and to preserve the text and get our result.
Here's a visualization of the process:
We Otsu's threshold to obtain a binary image then find contours to determine the average rectangular contour area. From here we remove the large outlier contours highlighted in green by filling contours
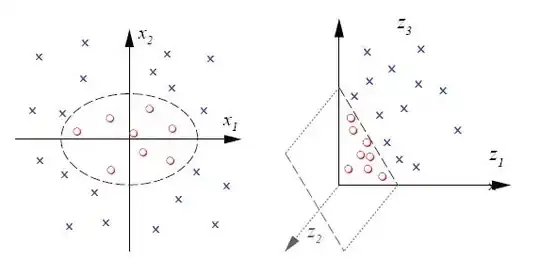
Next we construct a vertical kernel and dilate to connect the characters. This step connects all the desired text to keep and isolates the noise into individual blobs.
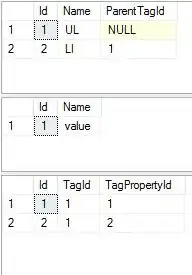
Now we find contours and filter using contour area to remove the small noise
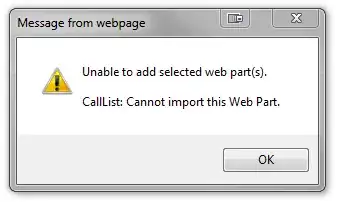
Here are all the removed noise particles highlighted in green
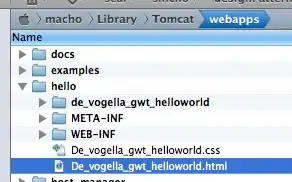
Result
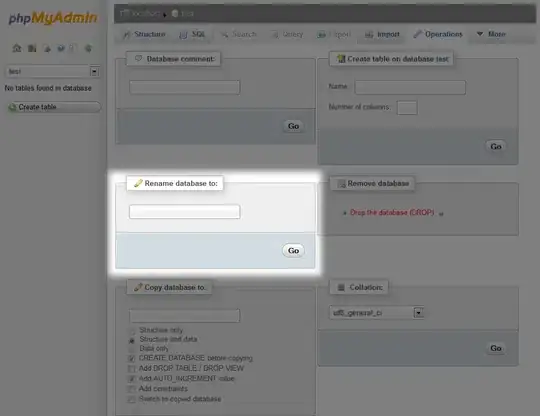
Code
import cv2
# Load image, grayscale, and Otsu's threshold
image = cv2.imread('1.png')
gray = cv2.cvtColor(image, cv2.COLOR_BGR2GRAY)
thresh = cv2.threshold(gray, 0, 255, cv2.THRESH_BINARY_INV + cv2.THRESH_OTSU)[1]
# Determine average contour area
average_area = []
cnts = cv2.findContours(thresh, cv2.RETR_EXTERNAL, cv2.CHAIN_APPROX_SIMPLE)
cnts = cnts[0] if len(cnts) == 2 else cnts[1]
for c in cnts:
x,y,w,h = cv2.boundingRect(c)
area = w * h
average_area.append(area)
average = sum(average_area) / len(average_area)
# Remove large lines if contour area is 5x bigger then average contour area
cnts = cv2.findContours(thresh, cv2.RETR_EXTERNAL, cv2.CHAIN_APPROX_SIMPLE)
cnts = cnts[0] if len(cnts) == 2 else cnts[1]
for c in cnts:
x,y,w,h = cv2.boundingRect(c)
area = w * h
if area > average * 5:
cv2.drawContours(thresh, [c], -1, (0,0,0), -1)
# Dilate with vertical kernel to connect characters
kernel = cv2.getStructuringElement(cv2.MORPH_RECT, (2,5))
dilate = cv2.dilate(thresh, kernel, iterations=3)
# Remove small noise if contour area is smaller than 4x average
cnts = cv2.findContours(dilate, cv2.RETR_EXTERNAL, cv2.CHAIN_APPROX_SIMPLE)
cnts = cnts[0] if len(cnts) == 2 else cnts[1]
for c in cnts:
area = cv2.contourArea(c)
if area < average * 4:
cv2.drawContours(dilate, [c], -1, (0,0,0), -1)
# Bitwise mask with input image
result = cv2.bitwise_and(image, image, mask=dilate)
result[dilate==0] = (255,255,255)
cv2.imshow('result', result)
cv2.imshow('dilate', dilate)
cv2.imshow('thresh', thresh)
cv2.waitKey()
Note: Traditional image processing is limited to thresholding, morphological operations, and contour filtering (contour approximation, area, aspect ratio, or blob detection). Since input images can vary based on character text size, finding a singular solution is quite difficult. You may want to look into training your own classifier with machine/deep learning for a dynamic solution.