Update: the LayerNormalization implementation I was using was inter-layer, not recurrent as in the original paper; results with latter may prove superior.
BatchNormalization
can work with LSTMs - the linked SO gives false advice; in fact, in my application of EEG classification, it dominated LayerNormalization
. Now to your case:
- "Can I add it before
Conv1D
"? Don't - instead, standardize your data beforehand, else you're employing an inferior variant to do the same thing
- Try both:
BatchNormalization
before an activation, and after - apply to both Conv1D
and LSTM
- If your model is exactly as you show it,
BN
after LSTM
may be counterproductive per ability to introduce noise, which can confuse the classifier layer - but this is about being one layer before output, not LSTM
- If you aren't using stacked
LSTM
with return_sequences=True
preceding return_sequences=False
, you can place Dropout
anywhere - before LSTM
, after, or both
- Spatial Dropout: drop units / channels instead of random activations (see bottom); was shown more effective at reducing coadaptation in CNNs in paper by LeCun, et al, w/ ideas applicable to RNNs. Can considerably increase convergence time, but also improve performance
recurrent_dropout
is still preferable to Dropout
for LSTM
- however, you can do both; just do not use with with activation='relu'
, for which LSTM
is unstable per a bug
- For data of your dimensionality, any sort of
Pooling
is redundant and may harm performance; scarce data is better transformed via a non-linearity than simple averaging ops
- I strongly recommend a
SqueezeExcite
block after your Conv; it's a form of self-attention - see paper; my implementation for 1D below
- I also recommend trying
activation='selu'
with AlphaDropout
and 'lecun_normal'
initialization, per paper Self Normalizing Neural Networks
- Disclaimer: above advice may not apply to NLP and embed-like tasks
Below is an example template you can use as a starting point; I also recommend the following SO's for further reading: Regularizing RNNs, and Visualizing RNN gradients
from keras.layers import Input, Dense, LSTM, Conv1D, Activation
from keras.layers import AlphaDropout, BatchNormalization
from keras.layers import GlobalAveragePooling1D, Reshape, multiply
from keras.models import Model
import keras.backend as K
import numpy as np
def make_model(batch_shape):
ipt = Input(batch_shape=batch_shape)
x = ConvBlock(ipt)
x = LSTM(16, return_sequences=False, recurrent_dropout=0.2)(x)
# x = BatchNormalization()(x) # may or may not work well
out = Dense(1, activation='relu')
model = Model(ipt, out)
model.compile('nadam', 'mse')
return model
def make_data(batch_shape): # toy data
return (np.random.randn(*batch_shape),
np.random.uniform(0, 2, (batch_shape[0], 1)))
batch_shape = (32, 21, 20)
model = make_model(batch_shape)
x, y = make_data(batch_shape)
model.train_on_batch(x, y)
Functions used:
def ConvBlock(_input): # cleaner code
x = Conv1D(filters=10, kernel_size=3, padding='causal', use_bias=False,
kernel_initializer='lecun_normal')(_input)
x = BatchNormalization(scale=False)(x)
x = Activation('selu')(x)
x = AlphaDropout(0.1)(x)
out = SqueezeExcite(x)
return out
def SqueezeExcite(_input, r=4): # r == "reduction factor"; see paper
filters = K.int_shape(_input)[-1]
se = GlobalAveragePooling1D()(_input)
se = Reshape((1, filters))(se)
se = Dense(filters//r, activation='relu', use_bias=False,
kernel_initializer='he_normal')(se)
se = Dense(filters, activation='sigmoid', use_bias=False,
kernel_initializer='he_normal')(se)
return multiply([_input, se])
Spatial Dropout: pass noise_shape = (batch_size, 1, channels)
to Dropout
- has the effect below; see Git gist for code:
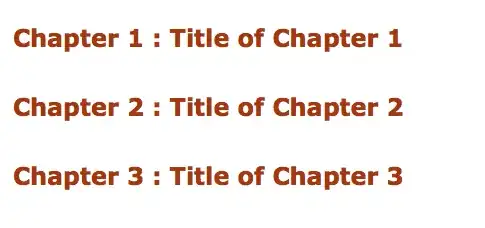