I am trying to find accurate locations for the corners on ink blotches as seen below:
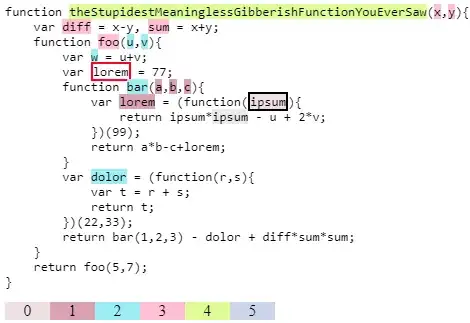
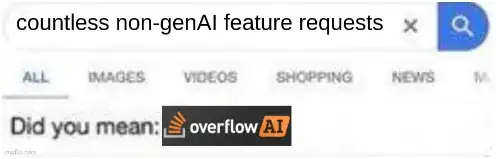
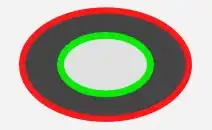
My idea is to fit lines to the edges and then find where they intersect. As of now, I've tried using cv2.approxPolyDP()
with various values of epsilon to approximate the edges, however this doesn't look like the way to go. My cv2.approxPolyDP
code gives the following result:
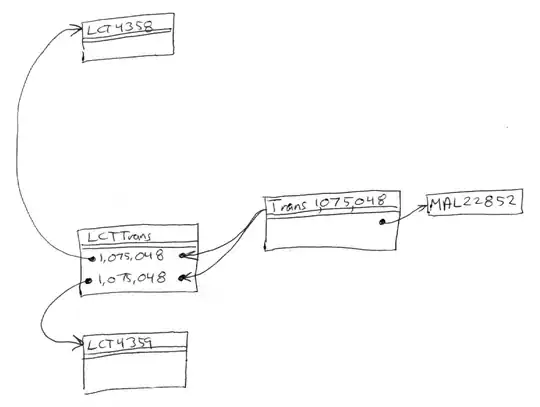
Ideally, this is what I want to produce (drawn on paint):

Are there CV functions in place for this sort of problem? I've considered using Gaussian blurring before the threshold step although that method does not seem like it would be very accurate for corner finding. Additionally, I would like this to be robust to rotated images, so filtering for vertical and horizontal lines won't necessarily work without other considerations.
Code:*
import numpy as np
from PIL import ImageGrab
import cv2
def process_image4(original_image): # Douglas-peucker approximation
# Convert to black and white threshold map
gray = cv2.cvtColor(original_image, cv2.COLOR_BGR2GRAY)
gray = cv2.GaussianBlur(gray, (5, 5), 0)
(thresh, bw) = cv2.threshold(gray, 128, 255, cv2.THRESH_BINARY + cv2.THRESH_OTSU)
# Convert bw image back to colored so that red, green and blue contour lines are visible, draw contours
modified_image = cv2.cvtColor(bw, cv2.COLOR_GRAY2BGR)
contours, hierarchy = cv2.findContours(bw, cv2.RETR_TREE, cv2.CHAIN_APPROX_SIMPLE)
cv2.drawContours(modified_image, contours, -1, (255, 0, 0), 3)
# Contour approximation
try: # Just to be sure it doesn't crash while testing!
for cnt in contours:
epsilon = 0.005 * cv2.arcLength(cnt, True)
approx = cv2.approxPolyDP(cnt, epsilon, True)
# cv2.drawContours(modified_image, [approx], -1, (0, 0, 255), 3)
except:
pass
return modified_image
def screen_record():
while(True):
screen = np.array(ImageGrab.grab(bbox=(100, 240, 750, 600)))
image = process_image4(screen)
cv2.imshow('window', image)
if cv2.waitKey(25) & 0xFF == ord('q'):
cv2.destroyAllWindows()
break
screen_record()
- A note about my code: I'm using screen capture so that I can process these images live. I have a digital microscope that can display live feed on a screen, so the constant screen recording will allow me to sample from the video feed and locate the corners live on the other half of my screen.