Here's a potential solution:
Obtain binary image. We load the image, convert to grayscale, apply a Gaussian blur, and then Otsu's threshold
Detect horizontal lines. We create a horizontal kernel and draw detected horizontal lines onto a mask
Detect vertical lines. We create a vertical kernel and draw detected vertical lines onto a mask
Perform morphological opening. We create a rectangular kernel and perform morph opening to smooth out noise and separate any connected contours
Find contours, draw rectangle, and extract ROI. We find contours and draw the bounding rectangle onto the image
Here's a visualization of each step:
Binary image
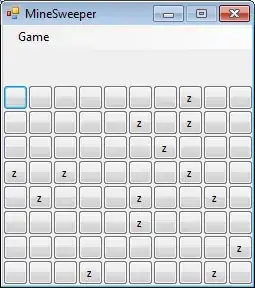
Detected horizontal and vertical lines drawn onto a mask
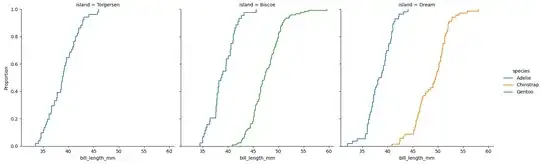
Morphological opening
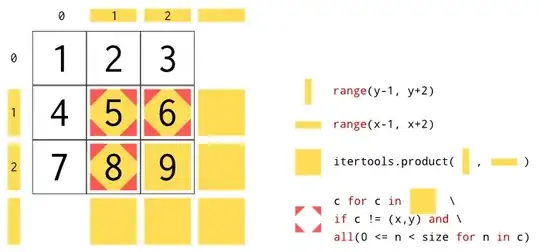
Result
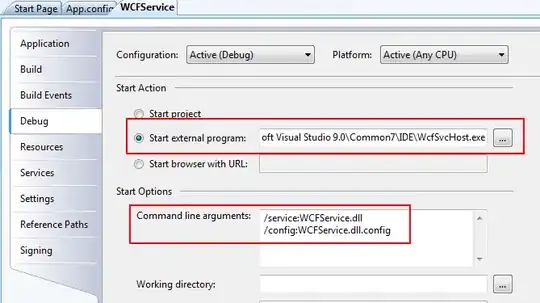
Individual extracted saved ROI
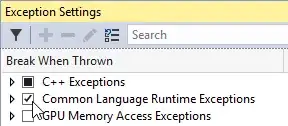
Note: To extract only the hand written numbers/letters out of each ROI, take a look at a previous answer in Remove borders from image but keep text written on borders (preprocessing before OCR)
Code
import cv2
import numpy as np
# Load image, grayscale, blur, Otsu's threshold
image = cv2.imread('1.png')
original = image.copy()
mask = np.zeros(image.shape, dtype=np.uint8)
gray = cv2.cvtColor(image, cv2.COLOR_BGR2GRAY)
blur = cv2.GaussianBlur(gray, (5,5), 0)
thresh = cv2.threshold(blur, 0, 255, cv2.THRESH_BINARY_INV + cv2.THRESH_OTSU)[1]
# Find horizontal lines
horizontal_kernel = cv2.getStructuringElement(cv2.MORPH_RECT, (50,1))
detect_horizontal = cv2.morphologyEx(thresh, cv2.MORPH_OPEN, horizontal_kernel, iterations=2)
cnts = cv2.findContours(detect_horizontal, cv2.RETR_EXTERNAL, cv2.CHAIN_APPROX_SIMPLE)
cnts = cnts[0] if len(cnts) == 2 else cnts[1]
for c in cnts:
cv2.drawContours(mask, [c], -1, (255,255,255), 3)
# Find vertical lines
vertical_kernel = cv2.getStructuringElement(cv2.MORPH_RECT, (1,50))
detect_vertical = cv2.morphologyEx(thresh, cv2.MORPH_OPEN, vertical_kernel, iterations=2)
cnts = cv2.findContours(detect_vertical, cv2.RETR_EXTERNAL, cv2.CHAIN_APPROX_SIMPLE)
cnts = cnts[0] if len(cnts) == 2 else cnts[1]
for c in cnts:
cv2.drawContours(mask, [c], -1, (255,255,255), 3)
# Morph open
mask = cv2.cvtColor(mask, cv2.COLOR_BGR2GRAY)
kernel = cv2.getStructuringElement(cv2.MORPH_RECT, (7,7))
opening = cv2.morphologyEx(mask, cv2.MORPH_OPEN, kernel, iterations=1)
# Draw rectangle and save each ROI
number = 0
cnts = cv2.findContours(opening, cv2.RETR_EXTERNAL, cv2.CHAIN_APPROX_SIMPLE)
cnts = cnts[0] if len(cnts) == 2 else cnts[1]
for c in cnts:
x,y,w,h = cv2.boundingRect(c)
cv2.rectangle(image, (x, y), (x + w, y + h), (36,255,12), 2)
ROI = original[y:y+h, x:x+w]
cv2.imwrite('ROI_{}.png'.format(number), ROI)
number += 1
cv2.imshow('thresh', thresh)
cv2.imshow('mask', mask)
cv2.imshow('opening', opening)
cv2.imshow('image', image)
cv2.waitKey()