I'm not sure if this answers all of your question, but should be a good start. Clarify in a comment or in your question should you need a different type of output.
It removes all points that are not inside one of the 'zone' polygons, counts them by zone and plots the zones colored by the number of points that fall within.
library(raster)
library(tidyverse)
library(sf)
#> Linking to GEOS 3.6.2, GDAL 2.2.3, PROJ 4.9.3
library(spatstat)
library(maptools)
#> Checking rgeos availability: TRUE
load(url("https://www.dropbox.com/s/iv1s5butsx2v01r/example.RData?dl=1"))
# alternatively, links to gists for each object
# https://gist.github.com/ericpgreen/d80665d22dfa1c05607e75b8d2163b84
# https://gist.github.com/ericpgreen/7f4d3cee3eb5efed5486f7f713306e96
p1 <- ggplot() +
geom_sf(data = zones) +
geom_sf(data = points) +
theme_minimal()
#Remove points outside of zones
points_inside <- st_intersection(points, zones)
#> although coordinates are longitude/latitude, st_intersection assumes that they are planar
#> Warning: attribute variables are assumed to be spatially constant throughout all
#> geometries
nrow(points)
#> [1] 308
nrow(points_inside)
#> [1] 201
p2 <- ggplot() +
geom_sf(data = zones) +
geom_sf(data = points_inside)
points_per_zone <- st_join(zones, points_inside) %>%
count(LocationID.x)
#> although coordinates are longitude/latitude, st_intersects assumes that they are planar
p3 <- ggplot() +
geom_sf(data = points_per_zone,
aes(fill = n)) +
scale_fill_viridis_c(option = 'C')
points_per_zone
#> Simple feature collection with 4 features and 2 fields
#> geometry type: POLYGON
#> dimension: XY
#> bbox: xmin: 34.0401 ymin: -1.076718 xmax: 34.17818 ymax: -0.9755066
#> epsg (SRID): 4326
#> proj4string: +proj=longlat +ellps=WGS84 +no_defs
#> # A tibble: 4 x 3
#> LocationID.x n geometry
#> * <dbl> <int> <POLYGON [°]>
#> 1 10 129 ((34.08018 -0.9755066, 34.0803 -0.9757393, 34.08046 -0.975…
#> 2 20 19 ((34.05622 -0.9959458, 34.05642 -0.9960835, 34.05665 -0.99…
#> 3 30 29 ((34.12994 -1.026372, 34.12994 -1.026512, 34.12988 -1.0266…
#> 4 40 24 ((34.11962 -1.001829, 34.11956 -1.002018, 34.11966 -1.0020…
cowplot::plot_grid(p1, p2, p3, nrow = 2, ncol = 2)

It seems I underestimated the difficulty of your problem. Is something like the plot below (& underlying data) what you're looking for?
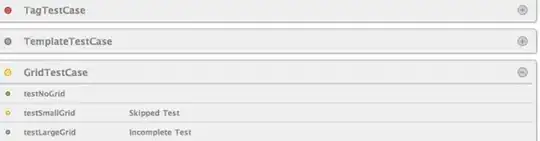
It uses raster with ~50x50 grid, raster::focal with a window of 9x9 using the mean to interpolate the data.