Here's a possible pipeline to solve your problem. The main idea is to identify the text, create a super blob of it with some morphology, locate the 4 corners of this super blob and feed the points to a perspective "unwarper" (or rectifier, or whatever you wish to call that perspective correction method).
Start by converting your image to grayscale and apply adaptive thresholding to it. Try the Gaussian or Mean methods with parameters that better fit your tests. This is the result I obtain after fiddling with the values for a bit:
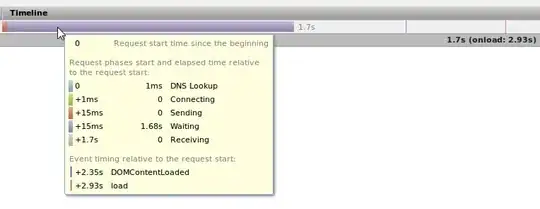
Now, the idea is to isolate just the text. The solution I applied is: obtain the biggest blobs and subtract them from the original image. You're going to need a method to calculate the area of each binary blob. Check this previous post for suggestions on how to implement one.
These are the biggest blobs from the image:

Subtract the largest blobs from the original image. This is the result:
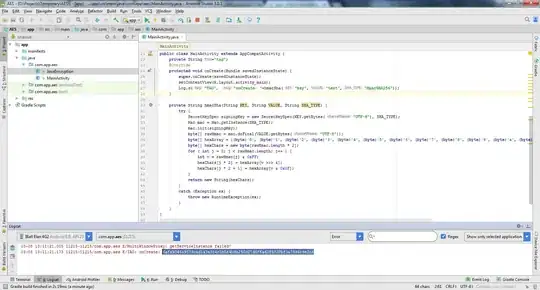
As you can see, the text is almost isolated. Let me clean up the little bits of pixels by applying, again, an area filter. This time to eliminate the small blobs. This is the result:
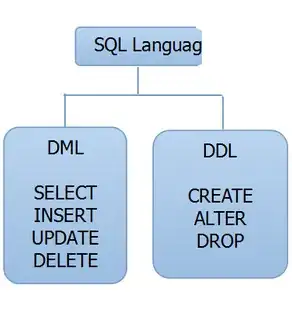
Very good, some characters are lost during the operation, but that’s ok. We need a nice continuous block of text, because we are gonna dilate the hell of it. I tried applying a rectangular structuring element of size 5 and 5 Op iterations. Erode the output with 5 more iterations afterward, so you end up with this nice - isolated - super blob were the text used to be:
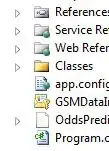
Check it out. The 3 markers you see are the centroids of the biggest blobs that I detected on the image. We need to find the 4 corners of the super blob. The biggest blob in the image is what we are after. I decided to re-use the area filter and look for the blob with the biggest area. This is the isolated super blob:
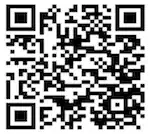
From here, the operations are pretty straightforward. Again, the goal is to get the four corners of this blob. You can fit a rectangle or apply an edge detector followed by Hough transform, to get the straight lines that follow the edges of the super blob.
I decided to apply a Canny Edge detector followed by Hough transform. Of course, I tuned the transform to filter only the possible lines I’m interested in – straight lines above a certain length. This is the result of the line detection:
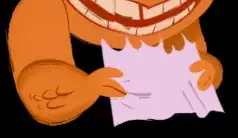
There's some extra info plotted on the image. The markers you see (red and yellow) are the start/endpoints of the lines. My idea here was to find a bunch of these lines and compute the mean of these points. The idea is that we have a cluster of points that are separated in "quadrants". If we compute the mean of the start and endpoints of each line per quadrant, we will end up with 4 means – and these are the approximate values of the super blob’s corners!
I applied K-means to the start and endpoints of the lines, but you very well prefer other methods of processing. That's ok. My approximate corners are identified by the big red O markers in the above image.
As I suggested, try giving a fixed output position for these corners. I defined the red rectangle for the corners to be mapped on. For this test, I pretty much adjusted the rectangle manually. The perspective correction yields this result:
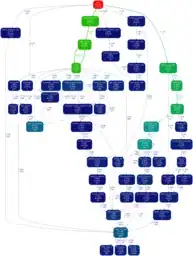
Some suggestions:
Depending on the resolution of the input image, you could downsize it
for a faster and better result, as your input seems big enough for
that.
Tune Hough Line Detection to yield larger lines. My current
configuration detects some smaller lines and that can hinder the
corner approximation.
I choose a somewhat robust method for calculating the 4 corners of
the super blob that I’ve personally used before (Edge detection +
Hough Line Transform + K-means) but whatever processing chain you
chose to obtain the data is entirely up to you!