python threads will not really help you to process in parallel since they are executed on the same one "real CPU thread", python threads are helpful when you deal with asynchronous HTTP calls
AboutProcessPoolExecutor
from the docs:
concurrent.futures.ProcessPoolExecutor()
The ProcessPoolExecutor
class is an Executor subclass that uses a pool of processes to execute
calls asynchronously. ProcessPoolExecutor uses the multiprocessing
module, which allows it to side-step the Global Interpreter Lock but
also means that only picklable objects can be executed and returned.
it can help you if you need high CPU processing, you can use:
import concurrent
def manipulate_values(k_v):
k, v = k_v
return_values = []
for i in v :
new_value = i ** 2 - 13
return_values.append(new_value)
return k, return_values
with concurrent.futures.ProcessPoolExecutor() as executor:
example_dict = dict(executor.map(manipulate_values, example_dict1.items()))
here is a simple benchmark, using a simple for
loop to process your data versus using ProcessPoolExecutor
, my scenario assume that for each item to be processed you need ~50ms CPU time:
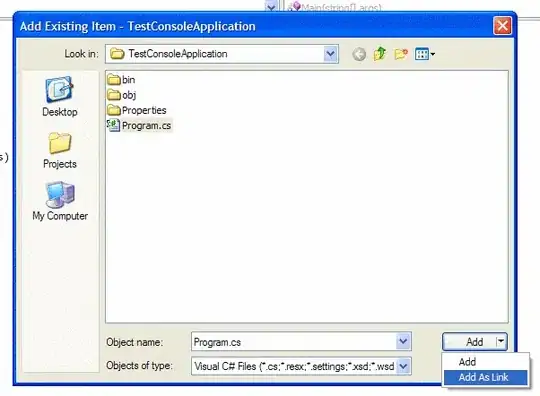
you can see the real benefit from ProcessPoolExecutor
if the CPU time per item to be processed is high
from simple_benchmark import BenchmarkBuilder
import time
import concurrent
b = BenchmarkBuilder()
def manipulate_values1(k_v):
k, v = k_v
time.sleep(0.05)
return k, v
def manipulate_values2(v):
time.sleep(0.05)
return v
@b.add_function()
def test_with_process_pool_executor(d):
with concurrent.futures.ProcessPoolExecutor() as executor:
return dict(executor.map(manipulate_values1, d.items()))
@b.add_function()
def test_simple_for_loop(d):
for key, value in d.items():
d[key] = manipulate_values2((key, value))
@b.add_arguments('Number of keys in dict')
def argument_provider():
for exp in range(2, 10):
size = 2**exp
yield size, {i: [i] * 10_000 for i in range(size)}
r = b.run()
r.plot()
if you do not set the number of workers for ProcessPoolExecutor the default number of workers will be equal with the number of processors on your machine (for the benchmark I used a pc with 8 CPU).
but in your case, with the data provided in your question, to process 1 item will take ~3 µs:
%timeit manipulate_values([367, 30, 847, 482, 887, 654, 347, 504, 413, 821])
2.32 µs ± 25.8 ns per loop (mean ± std. dev. of 7 runs, 100000 loops each)
in which case the benchmark will look:
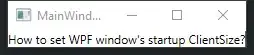
So it is better to use a simple for loop if the CPU time for one item to be processed is low.
a good point raised by @user3666197 is the case when you have huge items/lists, I benchmarked both approaches using 1_000_000_000
random numbers in a list:
as you can see in this case is more suitable to use ProcessPoolExecutor
from simple_benchmark import BenchmarkBuilder
import time
import concurrent
from random import choice
b = BenchmarkBuilder()
def manipulate_values1(k_v):
k, v = k_v
return_values = []
for i in v:
new_value = i ** 2 - 13
return_values.append(new_value)
return k, return_values
def manipulate_values2(v):
return_values = []
for i in v:
new_value = i ** 2 - 13
return_values.append(new_value)
return return_values
@b.add_function()
def test_with_process_pool_executor(d):
with concurrent.futures.ProcessPoolExecutor() as executor:
return dict(executor.map(manipulate_values1, d.items()))
@b.add_function()
def test_simple_for_loop(d):
for key, value in d.items():
d[key] = manipulate_values2(value)
@b.add_arguments('Number of keys in dict')
def argument_provider():
for exp in range(2, 5):
size = 2**exp
yield size, {i: [choice(range(1000)) for _ in range(1_000_000)] for i in range(size)}
r = b.run()
r.plot()
expected since to process one item it takes ~209ms:
l = [367] * 1_000_000
%timeit manipulate_values2(l)
# 209 ms ± 1.45 ms per loop (mean ± std. dev. of 7 runs, 1 loop each)
still, the fastest option will be to use numpy.arrays with the for
loop solution:
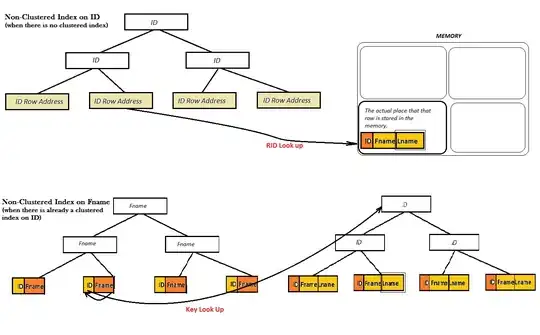
from simple_benchmark import BenchmarkBuilder
import time
import concurrent
import numpy as np
b = BenchmarkBuilder()
def manipulate_values1(k_v):
k, v = k_v
return k, v ** 2 - 13
def manipulate_values2(v):
return v ** 2 - 13
@b.add_function()
def test_with_process_pool_executor(d):
with concurrent.futures.ProcessPoolExecutor() as executor:
return dict(executor.map(manipulate_values1, d.items()))
@b.add_function()
def test_simple_for_loop(d):
for key, value in d.items():
d[key] = manipulate_values2(value)
@b.add_arguments('Number of keys in dict')
def argument_provider():
for exp in range(2, 7):
size = 2**exp
yield size, {i: np.random.randint(0, 1000, size=1_000_000) for i in range(size)}
r = b.run()
r.plot()
it is expected that the simple for
loop to be faster since to process one numpy.array takes < 1ms:
def manipulate_value2( input_list ):
return input_list ** 2 - 13
l = np.random.randint(0, 1000, size=1_000_000)
%timeit manipulate_values2(l)
# 951 µs ± 5.7 µs per loop (mean ± std. dev. of 7 runs, 1000 loops each)