A good deep learning library that I have used in the past for my work is called Mask R-CNN, or Mask Regions-Convolutional Neural-Network. Although I have only used this algorithm on images and not on videos, the same principles apply, and it's very easy to make the transition to detection objects in a video. The algorithm uses Tensorflow and Keras, where you can split your input data, i.e images of people, into two sets, training, and validation.
For training, use a third party software like via, to annotate the people in the images. After the annotations have been drawn, you will export a JSON file with all annotations drawn, which will be used for the training process. Do the same thing for the validation phase, BUT make sure the images in the validation have not been seen before by the algorithm.
Once you have annotated both groups and generated JSON files, you then can start training the algorithm. Mask R-CNN makes it very easy to train, with all you need to do is pass one line full of commands to start it. If you want to train data on your GPU instead of your CPU, then install Nvidia's CUDA, which works very well with supported GPUs, and requires no coding after the installation.
During the training stage, you will be generating weights files, which are stored in the .h5 format. Depending on the number of epochs you choose, there will be a weights file generated per epoch. Once the training has finished, you then will just have to reference that weights file anytime you want to detect relevant objects, i.e. in your video feed.
Some important info:
- Mask R-CNN is somewhat of an older algorithm, but it still works flawlessly today. Although some people have updated the algorithm to Tenserflow 2.0+, to get the best use out of it, use the following.
- Tensorflow-gpu 1.13.2+
- Keras 2.0.0+
- CUDA 9.0 to 10.0
Honestly, the hardest part for me in the past was not using the algorithm, but finding the right versions of Tensorflow, Keras, and CUDA, that all play well with each other, and don't error out. Although the above-mentioned versions will work, try and see if you can upgrade or downgrade certain libraries to see if you can get better results.
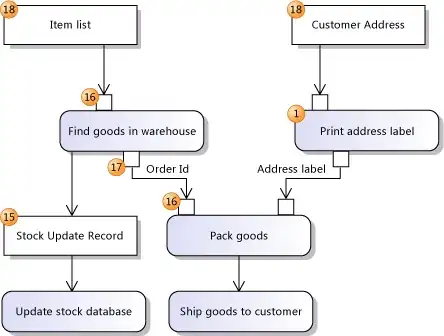
Article about Mask R-CNN with video, I find it to be very useful and resourceful.
https://www.pyimagesearch.com/2018/11/19/mask-r-cnn-with-opencv/
The GitHub repo can be found below.
https://github.com/matterport/Mask_RCNN
EDIT
You can use this method across multiple cameras, just set up multiple video captures within a computer vision library like OpenCV. I assume this would be done with Python, which both Mask R-CNN and OpenCV are primarly based in.