You may find bounding rectangle of the largest contour that is not white.
I suggest using the following stages:
- Convert image from BGR to Gray.
- Convert from gray to to binary image.
Use automatic threshold (use cv2.THRESH_OTSU
flag) and invert polarity.
The result is white color where original image is dark, and black where image is bright.
- Find contours using
cv2.findContours()
(as Mark Setchell commented).
Finding the outer contour is simpler solution than detecting the edges.
- Find the bounding rectangle of the contour with the maximum area.
- Crop the bounding rectangle from the input image.
I used NumPy array slicing instead of using pillow.
Here is the code:
import cv2
# Read input image
img = cv2.imread('img.jpg')
# Convert from BGR to Gray.
gray = cv2.cvtColor(img, cv2.COLOR_BGR2GRAY)
# Convert to binary image using automatic threshold and invert polarity
_, thresh = cv2.threshold(gray, 0, 255, cv2.THRESH_BINARY_INV + cv2.THRESH_OTSU)
# Find contours on thresh
cnts = cv2.findContours(thresh, cv2.RETR_EXTERNAL, cv2.CHAIN_APPROX_NONE)[-2] # Use index [-2] to be compatible to OpenCV 3 and 4
# Get contour with maximum area
c = max(cnts, key=cv2.contourArea)
x, y, w, h = cv2.boundingRect(c)
# Crop the bounding rectangle (use .copy to get a copy instead of slice).
crop = img[y:y+h, x:x+w, :].copy()
# Draw red rectangle for testing
cv2.rectangle(img, (x, y), (x+w, y+h), (0, 0, 255), thickness = 2)
# Show result
cv2.imshow('img', img)
cv2.imshow('crop', crop)
cv2.waitKey(0)
cv2.destroyAllWindows()
Result:
crop
:
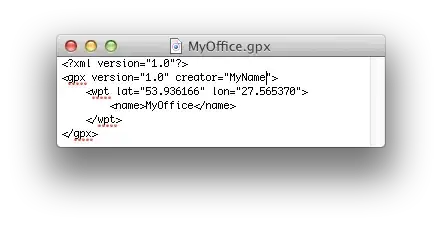
img
:
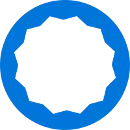
thresh
:
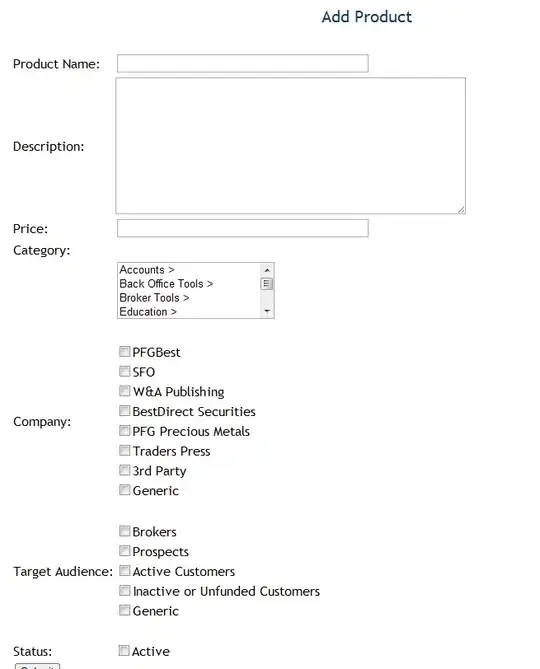