Benchmarking
We will just benchmark across various datasets and draw conclusions from them.
Timings
Using benchit
package (few benchmarking tools packaged together; disclaimer: I am its author) to benchmark proposed solutions.
Benchmarking code :
import numpy as np
import benchit
def numpy_concatenate(a, b):
return np.concatenate((a,b),axis=1)
def numpy_hstack(a, b):
return np.hstack((a,b))
def preallocate(a, b):
m,n = a.shape[1], b.shape[1]
out = np.empty((a.shape[0],m+n), dtype=np.result_type((a.dtype, b.dtype)))
out[:,:m] = a
out[:,m:] = b
return out
funcs = [numpy_concatenate, numpy_hstack, preallocate]
R = np.random.rand
inputs = {n: (R(1000,1000), R(1000,n)) for n in [100, 200, 500, 1000, 200, 5000]}
t = benchit.timings(funcs, inputs, multivar=True, input_name='Col length of b')
t.plot(logy=False, logx=True, savepath='plot_1000rows.png')
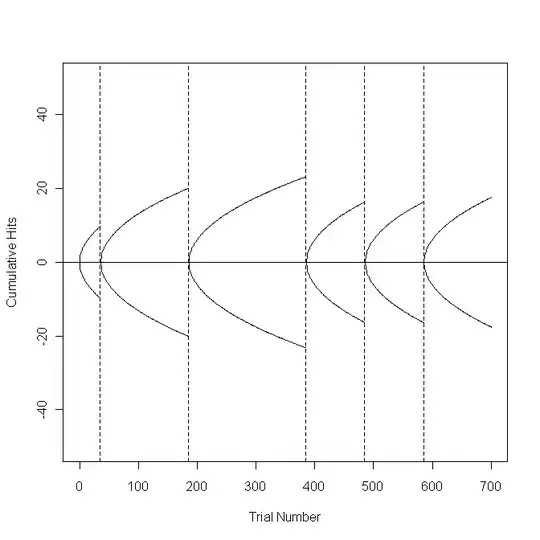
Conclusion : They are comparable on timings.
Memory profiling
On memory side, np.hstack
should be similar to np.concatenate
. So, we will use one of them.
Let's setup an input dataset with large 2D arrays. We will do some memory benchmarking.
Setup code :
# Filename : memprof_npconcat_preallocate.py
import numpy as np
from memory_profiler import profile
@profile(precision=10)
def numpy_concatenate(a, b):
return np.concatenate((a,b),axis=1)
@profile(precision=10)
def preallocate(a, b):
m,n = a.shape[1], b.shape[1]
out = np.empty((a.shape[0],m+n), dtype=np.result_type((a.dtype, b.dtype)))
out[:,:m] = a
out[:,m:] = b
return out
R = np.random.rand
a,b = R(1000,1000), R(1000,1000)
if __name__ == '__main__':
numpy_concatenate(a, b)
if __name__ == '__main__':
preallocate(a, b)
So, a
is 1000x1000 and same for b
.
Run :
$ python3 -m memory_profiler memprof_npconcat_preallocate.py
Filename: memprof_npconcat_preallocate.py
Line # Mem usage Increment Line Contents
================================================
9 69.3281250000 MiB 69.3281250000 MiB @profile(precision=10)
10 def numpy_concatenate(a, b):
11 84.5546875000 MiB 15.2265625000 MiB return np.concatenate((a,b),axis=1)
Filename: memprof_npconcat_preallocate.py
Line # Mem usage Increment Line Contents
================================================
13 69.3554687500 MiB 69.3554687500 MiB @profile(precision=10)
14 def preallocate(a, b):
15 69.3554687500 MiB 0.0000000000 MiB m,n = a.shape[1], b.shape[1]
16 69.3554687500 MiB 0.0000000000 MiB out = np.empty((a.shape[0],m+n), dtype=np.result_type((a.dtype, b.dtype)))
17 83.6484375000 MiB 14.2929687500 MiB out[:,:m] = a
18 84.4218750000 MiB 0.7734375000 MiB out[:,m:] = b
19 84.4218750000 MiB 0.0000000000 MiB return out
Thus, for preallocate
method, the total mem consumption is 14.2929687500
+ 0.7734375000
, which is slightly lesser than 15.2265625000
.
Changing the sizes for input arrays to 5000x5000 for both a
and b
-
$ python3 -m memory_profiler memprof_npconcat_preallocate.py
Filename: memprof_npconcat_preallocate.py
Line # Mem usage Increment Line Contents
================================================
9 435.4101562500 MiB 435.4101562500 MiB @profile(precision=10)
10 def numpy_concatenate(a, b):
11 816.8515625000 MiB 381.4414062500 MiB return np.concatenate((a,b),axis=1)
Filename: memprof_npconcat_preallocate.py
Line # Mem usage Increment Line Contents
================================================
13 435.5351562500 MiB 435.5351562500 MiB @profile(precision=10)
14 def preallocate(a, b):
15 435.5351562500 MiB 0.0000000000 MiB m,n = a.shape[1], b.shape[1]
16 435.5351562500 MiB 0.0000000000 MiB out = np.empty((a.shape[0],m+n), dtype=np.result_type((a.dtype, b.dtype)))
17 780.3203125000 MiB 344.7851562500 MiB out[:,:m] = a
18 816.9296875000 MiB 36.6093750000 MiB out[:,m:] = b
19 816.9296875000 MiB 0.0000000000 MiB return out
Again, the total from preallocation is lesser.
Conclusion : Preallocation method has slightly better memory benefits, which in a way makes sense. With concatenate, we have three arrays involved src1 + src2 -> dst, whereas with preallocation, there's just src and dst with lesser memory congestion though in two steps.