You've probably figured out a solution by now but, for the sake of others who might be looking for it, here's some code that I've used to mimic the parallel analysis from the psych library:
import pandas as pd
from factor_analyzer import FactorAnalyzer
import numpy as np
import matplotlib.pyplot as plt
def _HornParallelAnalysis(data, K=10, printEigenvalues=False):
################
# Create a random matrix to match the dataset
################
n, m = data.shape
# Set the factor analysis parameters
fa = FactorAnalyzer(n_factors=1, method='minres', rotation=None, use_smc=True)
# Create arrays to store the values
sumComponentEigens = np.empty(m)
sumFactorEigens = np.empty(m)
# Run the fit 'K' times over a random matrix
for runNum in range(0, K):
fa.fit(np.random.normal(size=(n, m)))
sumComponentEigens = sumComponentEigens + fa.get_eigenvalues()[0]
sumFactorEigens = sumFactorEigens + fa.get_eigenvalues()[1]
# Average over the number of runs
avgComponentEigens = sumComponentEigens / K
avgFactorEigens = sumFactorEigens / K
################
# Get the eigenvalues for the fit on supplied data
################
fa.fit(data)
dataEv = fa.get_eigenvalues()
# Set up a scree plot
plt.figure(figsize=(8, 6))
################
### Print results
################
if printEigenvalues:
print('Principal component eigenvalues for random matrix:\n', avgComponentEigens)
print('Factor eigenvalues for random matrix:\n', avgFactorEigens)
print('Principal component eigenvalues for data:\n', dataEv[0])
print('Factor eigenvalues for data:\n', dataEv[1])
# Find the suggested stopping points
suggestedFactors = sum((dataEv[1] - avgFactorEigens) > 0)
suggestedComponents = sum((dataEv[0] - avgComponentEigens) > 0)
print('Parallel analysis suggests that the number of factors = ', suggestedFactors , ' and the number of components = ', suggestedComponents)
################
### Plot the eigenvalues against the number of variables
################
# Line for eigenvalue 1
plt.plot([0, m+1], [1, 1], 'k--', alpha=0.3)
# For the random data - Components
plt.plot(range(1, m+1), avgComponentEigens, 'b', label='PC - random', alpha=0.4)
# For the Data - Components
plt.scatter(range(1, m+1), dataEv[0], c='b', marker='o')
plt.plot(range(1, m+1), dataEv[0], 'b', label='PC - data')
# For the random data - Factors
plt.plot(range(1, m+1), avgFactorEigens, 'g', label='FA - random', alpha=0.4)
# For the Data - Factors
plt.scatter(range(1, m+1), dataEv[1], c='g', marker='o')
plt.plot(range(1, m+1), dataEv[1], 'g', label='FA - data')
plt.title('Parallel Analysis Scree Plots', {'fontsize': 20})
plt.xlabel('Factors/Components', {'fontsize': 15})
plt.xticks(ticks=range(1, m+1), labels=range(1, m+1))
plt.ylabel('Eigenvalue', {'fontsize': 15})
plt.legend()
plt.show();
If you call the above like this:
_HornParallelAnalysis(myDataSet)
You should get something like the following:
Example output for parallel analysis:
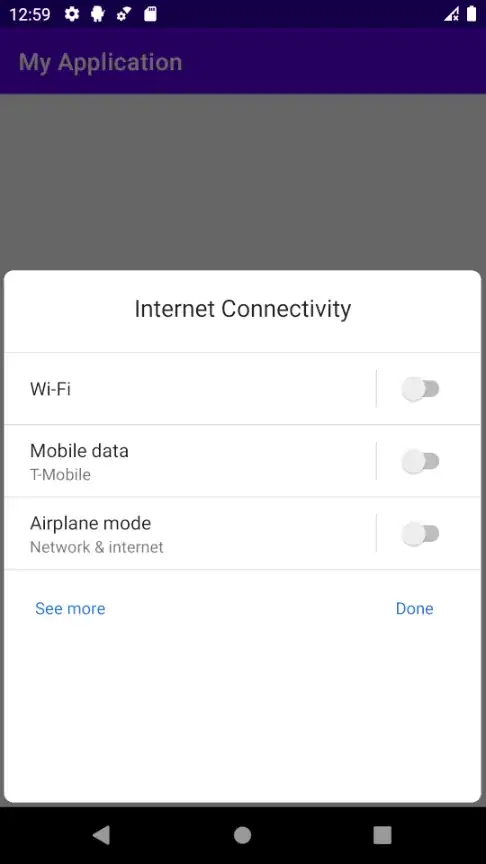