You can use the cv_results_
attribute of GridSearchCV
and get the results for each combination of hyperparameters. Validation Curve
is meant to depict the impact of single parameter in training and cross validation scores.
Since fine tuning is done for multiple parameters in GridSearchCV
, multiple plots are required to vizualise the impact of each parameter. Point to note is that the other parameter's impact has to be averaged out. This can be achieved by doing groupby
on each parameter separately.
For mean train and test - the mean of means would work out, but for standard deviation we have to use pooled variance because std deviations for each combination in Cross validation is almost constant.
from sklearn.datasets import make_classification
from sklearn.model_selection import GridSearchCV, train_test_split
from sklearn.ensemble import RandomForestClassifier
import matplotlib.pyplot as plt
import numpy as np
X, y = make_classification(n_samples=1000,
n_features=100, n_informative=2,
class_sep=0.1,random_state=7)
X_train, X_test, y_train, y_test = train_test_split(X, y, test_size=0.3)
grid_params = {
'n_estimators': [10, 20],
'max_features': ['sqrt', 'log2'],
'criterion': ['gini', 'entropy'],
'max_depth': [2, 5,]
}
cv = 5
gs = GridSearchCV(
RandomForestClassifier(random_state=42),
grid_params,
cv=cv,
verbose=1,
n_jobs=-1,
return_train_score=True # set this for train score
)
gs.fit(X_train, y_train)
import pandas as pd
df = pd.DataFrame(gs.cv_results_)
results = ['mean_test_score',
'mean_train_score',
'std_test_score',
'std_train_score']
# https://en.wikipedia.org/wiki/Pooled_variance#Pooled_standard_deviation
def pooled_var(stds):
n = cv # size of each group
return np.sqrt(sum((n-1)*(stds**2))/ len(stds)*(n-1))
fig, axes = plt.subplots(1, len(grid_params),
figsize = (5*len(grid_params), 7),
sharey='row')
axes[0].set_ylabel("Score", fontsize=25)
lw = 2
for idx, (param_name, param_range) in enumerate(grid_params.items()):
grouped_df = df.groupby(f'param_{param_name}')[results]\
.agg({'mean_train_score': 'mean',
'mean_test_score': 'mean',
'std_train_score': pooled_var,
'std_test_score': pooled_var})
previous_group = df.groupby(f'param_{param_name}')[results]
axes[idx].set_xlabel(param_name, fontsize=30)
axes[idx].set_ylim(0.0, 1.1)
axes[idx].plot(param_range,
grouped_df['mean_train_score'],
label="Training score",
color="darkorange",
lw=lw)
axes[idx].fill_between(param_range,
grouped_df['mean_train_score'] - grouped_df['std_train_score'],
grouped_df['mean_train_score'] + grouped_df['std_train_score'],
alpha=0.2,
color="darkorange",
lw=lw)
axes[idx].plot(param_range,
grouped_df['mean_test_score'],
label="Cross-validation score",
color="navy",
lw=lw)
axes[idx].fill_between(param_range,
grouped_df['mean_test_score'] - grouped_df['std_test_score'],
grouped_df['mean_test_score'] + grouped_df['std_test_score'],
alpha=0.2,
color="navy",
lw=lw)
handles, labels = axes[0].get_legend_handles_labels()
fig.suptitle('Validation curves', fontsize=40)
fig.legend(handles, labels, loc=8, ncol=2, fontsize=20)
fig.subplots_adjust(bottom=0.25, top=0.85)
plt.show()
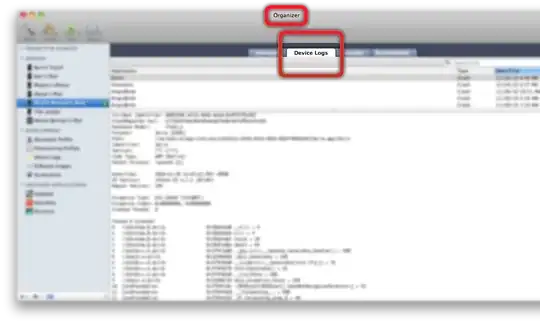
Note: line plots are not the right one for parameters with string values like criterion
, you could modify it to be bar plots with error bars.