Nothing that you can do will make it easy to see 2839 nodes with 9379 links.
There just isn't that much space on the screen. Nevertheless, I have some suggestions
that may provide more insight than just passing the graph into plot.
First, a quick glance at your plot reveals that this graph is not composed of a single
connected component.
COMP = components(PRIN_graph)
table(COMP$membership)
1 2 3 4 5 6 7 8 9 10 11 12 13 14
2696 42 2 4 18 13 2 7 7 2 3 2 2 2
15 16 17 18 19 20 21 22 23 24 25 26 27
2 6 14 3 1 1 1 2 1 3 1 1 1
So 2696 of the nodes are in a single large component and the remaining 143
are in 26 small components. The 2696 nodes in the big component overwhelm
the smaller components and the 26 small components acts as visual clutter
for the big component. Let's separate the 26 small components.
SC = which(COMP$membership != 1)
SmallComps = induced_subgraph(PRIN_graph, SC)
Now it is easy to see the community structure on all of these small components.
SC.gn.comm <- cluster_edge_betweenness(SmallComps)
colors <- rainbow(max(membership(SC.gn.comm)))
plot(SC.gn.comm, SmallComps, vertex.size = 6,
vertex.color=colors[membership(SC.gn.comm)],
vertex.label = NA, edge.width = 1)
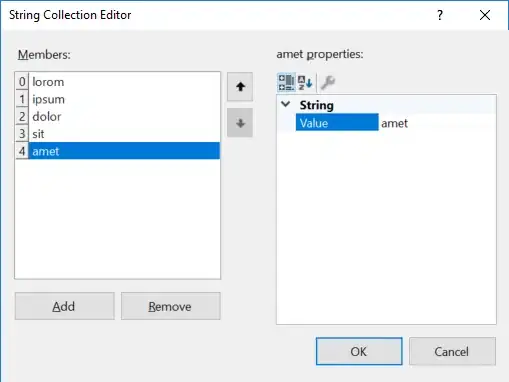
Mostly, small components comprised of a single community,
although there are a few with some structure.
That was the easy part, now let's look at the big component.
LC = which(COMP$membership == 1)
LargeComp = induced_subgraph(PRIN_graph, LC)
Girvan-Newman produces 43 communities within this large component
LC.gn.comm <- cluster_edge_betweenness(LargeComp)
max(LC.gn.comm$membership)
[1] 43
But simply plotting that still leaves a mess.
par(mar=c(0,0,0,0))
colors <- rainbow(max(membership(LC.gn.comm)))
set.seed(1234)
plot(LC.gn.comm, LargeComp, vertex.size = 6,
vertex.color=colors[membership(LC.gn.comm)],
vertex.label = NA, edge.width = 1)
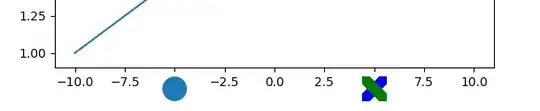
I will suggest two ways to improve the appearance of this graph:
separating the communities and contracting the communities.
Separating Communities
Based on this previous answer,
we can position vertices in the same community group together and make different
communities stay further apart.
LC_Grouped = LargeComp
E(LC_Grouped)$weight = 1
for(i in unique(membership(LC.gn.comm))) {
GroupV = which(membership(LC.gn.comm) == i)
LC_Grouped = add_edges(LC_Grouped, combn(GroupV, 2), attr=list(weight=6))
}
set.seed(1234)
LO = layout_with_fr(LC_Grouped)
colors <- rainbow(max(membership(LC.gn.comm)))
par(mar=c(0,0,0,0))
plot(LC.gn.comm, LargeComp, layout=LO,
vertex.size = 6,
vertex.color=colors[membership(LC.gn.comm)],
vertex.label = NA, edge.width = 1)
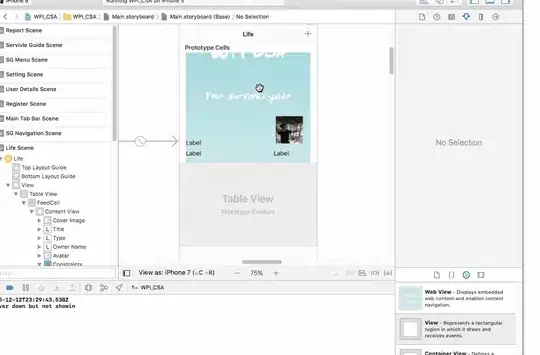
This makes the communities stand out better, but it is still pretty hard
to see the relationships. So another option is
Contract the Communities
Just plot a single node for each community. Here, I make the area of each
community vertex proportional to the number of members of that community
and I colored the vertices using a coarse grouping based on their degrees.
GN.Comm = simplify(contract(LargeComp, membership(LC.gn.comm)))
D = unname(degree(GN.Comm))
set.seed(1234)
par(mar=c(0,0,0,0))
plot(GN.Comm, vertex.size=sqrt(sizes(LC.gn.comm)),
vertex.label=1:43, vertex.cex = 0.8,
vertex.color=round(log(D))+1)

You can see that some communities barely connect to any others and some are
very well connected. None of these visualizations are perfect, but I hope that they might provide some insight into the structure and relationships.