The skip =
and n_max =
arguments in readr::read_tsv()
can be used to control how much data is read from a tab separated file into a data frame.
To read the first 10 observations so one can to see the column names, one can run:
library(readr)
file_url <- "http://samhda.s3-us-gov-west-1.amazonaws.com/s3fs-public/field-uploads-protected/studies/NSDUH-2002-2018/NSDUH-2002-2018-datasets/NSDUH-2002-2018-DS0001/NSDUH-2002-2018-DS0001-bundles-with-study-info/NSDUH-2002-2018-DS0001-bndl-data-tsv.zip"
zip <- tempfile(fileext = ".zip")
tsv_file <- download.file(file_url, zip, mode = "wb")
unzip_f <- unzip(zip,exdir="./data")
df <- read_tsv(unzip_f, col_names= TRUE, n_max = 10)
At this point we can retrieve the column names with the colnames()
function.
col_names <- colnames(df)
We'll now validate the amount of RAM consumed by 100,000 rows of the data, and calculate the load time for 100,000 observations.
system.time(df_100000 <- read_tsv("./data/NSDUH_2002_2018_tab.tsv",
col_names = TRUE, n_max = 100000))
format(object.size(df_100000),units = "auto")
user system elapsed
55.276 4.136 60.559
> format(object.size(df_100000),units = "auto")
[1] "2.7 Gb"
At this point we can safely read about 200,000 observations at a time from the raw data file on a machine that has 8Gb of RAM.
Next, we'll figure out how many rows of data are in the raw data file. We can use the col_types =
argument of read_tsv()
and set all columns except the first one to -
, which tells read_tsv()
not to read a column. We also calculate the size of the data frame with one column and all observations.
theTypes <- c("n",rep("_",3661))
system.time(df_obs <- read_tsv("./data/NSDUH_2002_2018_tab.tsv",col_types = theTypes,
col_names = TRUE))
nrow(df_obs)
format(object.size(df_obs),units = "auto")
user system elapsed
175.208 27.694 210.948
> nrow(df_obs)
[1] 949285
> format(object.size(df_obs),units = "auto")
[1] "39.8 Mb"
It took almost 4 minutes to read all observations for a single column of data from the raw data file on a MacBook Pro 15 with an Intel i7-4870HQ processor at 2.5Ghz.
The result of nrow()
tells us that there are 949,285 rows in the raw data file. If we break the file up into 200,000 observation chunks, we can read them and save them as RDS files with saveRDS()
for subsequent processing.
read the file iteratively and write as RDS
for(i in 1:5){
df <- read_tsv("./data/NSDUH_2002_2018_tab.tsv",
skip = (i - 1) * 200000,
n_max = 200000,
col_names = c_names)
saveRDS(df,paste0("./data/usnuh_",i,".RDS"))
}
At this point, usnuh_1.RDS
through usnuh_5.RDS
can be read individually into R and analyzed.
NOTE: the for()
loop overwrites the data frame created during the previous iteration, therefore we can read and write all of the files without running out of RAM. It's important to remember that one can only load 1 file with 200,000 observations and use it for data analysis on a machine with 8Gb of RAM. In order to use a different portion of the data, one needs to use the rm()
function to remove the current data before loading another 200,000 observation RDS file into RAM.
Reading the last 200,000 rows
Per the comments, here is code that can be used to read the last 200K rows of the file.
# read last 200K rows. first read one row to obtain column names
library(readr)
df <- read_tsv("./data/NSDUH_2002_2018_tab.tsv",
col_names = TRUE, n_max = 1)
c_names <- colnames(df)
# next, configure skip = relative to end of file and read
df <- read_tsv("./data/NSDUH_2002_2018_tab.tsv",
skip = (949258 - 200000),
n_max = 200000,
col_names = c_names)
When we view the data frame in the environment viewer, we can see that it contains 200,000 observations.
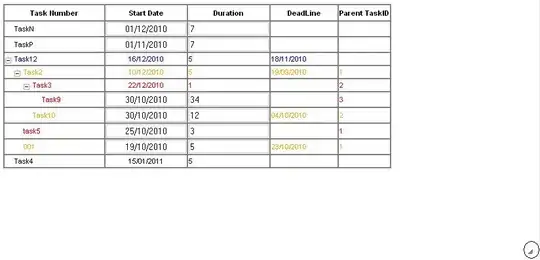