Welcome to SO!
You've run into a subtle problem that confuses a lot of people with far more experience than yourself. The problem is that ggplot2
evaluates lazily. Put simply, that means that it "makes a note" of what it needs to do when you tell it what you want, but doesn't actually do anything until the last possible moment.
Here, you tell ggplot that you want to add a geom
in your for
loop. ggplot makes a note of the geom
's definition, but doesn't evaluate it. "At the last moment" is when you call ggplotly
. Now ggplot
realises it's got some work to do. For each geom
, it notices that it needs to know the value of i
. So it looks it up and finds the value 5
. Hence your problem.
There are several ways to solve this. With your code, my preferred option is to replace the for
loop with an lapply
. Unlike a for
loop, lapply
forces evaluation of variables at the time of execution.
I believe you could also keep the for
loop and wrap each reference to i
in force()
, though I've not personally tried that.
The best approach in the long run, in my opinion, would be to make your workflow tidy and avoid the need for either the for
loop or lapply
altogether. This will also give you the benefits of more compact, robust and readable code that will almost certainly run faster. [I did some work the other day that converted a loop similar to yours to a tidy solution and the run time was reduced from nearly 40 seconds to under 2.]
Also, please read this post for advice on how to create a minimum working example. Providing MWEs will maximise your chances of getting a useful answer.
Update
To expand on my comment about the advantages of using a tidy data approach...
First synthesize some data as you haven't provided any. I'll try to match the structure of your data, but not your values. The only difference to your datasets is that I've added an ID
variable to identify the simulation run/real world dataset that each observation comes from.
library(lubridate)
library(tidyverse)
inVivoBG <- tibble(
ID="Real-world data",
DateTime2=seq(as_date("2006-03-01"), as_date("2015-03-01"), "3 months"),
VALUE=100 + rnorm(37, mean=150, sd=20)
)
listOfSimResults <- lapply(
1:5,
function(x) {
tibble(
ID=paste0("simRun-", x),
DateTime2=seq(as_date("2006-03-01"), as_date("2015-03-01"), "3 months"),
VALUE=100 + rnorm(37, mean=150, sd=20)
)
}
)
Now combine the various data frames into a single one.
data <- bind_rows(inVivoBG, listOfSimResults)
At this point, the construction of your plot is a single line call.
data %>%
ggplot() +
geom_line(mapping = aes(x = DateTime2, y = VALUE, color = ID))
Giving
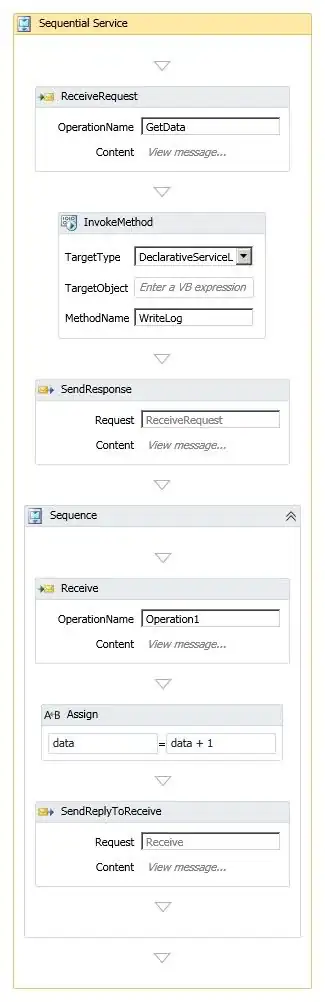
This approach avoids the need for a custom function or the need for lapply
. It is also robust with respect to the number of lines required and their labels. Personally, I also think it's far easier to understand.