Without having to resort to something as complex (and large/slow to import) as SITK or CV with complicated image analysis - you can do it easily just using numpy.
That's going to be a lot faster and reliable IMHO:
# if a is your image:
same_cols = np.all(a == a[0, :], axis=0)
same_cols_index = np.where(same_cols==False)[0]
C0,C1 = same_cols_index[0], same_cols_index[-1] + 1
same_rows = np.all(a == a[:, 0], axis=1)
same_rows_index = np.where(same_rows==False)[0]
R0,R1 = same_rows_index[0], same_rows_index[-1] + 1
print('rows', R0, R1)
print('cols', C0, C1)
a_snipped = a[R0:R1, C0:C1]
The logic here is
- Find all rows and columns that have all values the same as the first row or column. You could replace that to be all rows/cols with value == 0 if you want
- Get the indexes of the rows/columns from (1) where they are not all the same (ie == False)
- Get the first and last index where they aren't all the same
- Use the row/column first and last indicies to get the corresponding slice of your array (note you need to add 1 to the last index to include it in the slice)
Example
# make a sample image
a = np.zeros((512,512), dtype=np.int32)
r0, r1 = 53, 421
c0, c1 = 43, 470
rnd = np.random.randint(-3000, 2000, (r1-r0, c1-c0))
a[r0:r1, c0:c1] = rnd
plt.imshow(a, cmap='gray', vmin=-50, vmax=50)
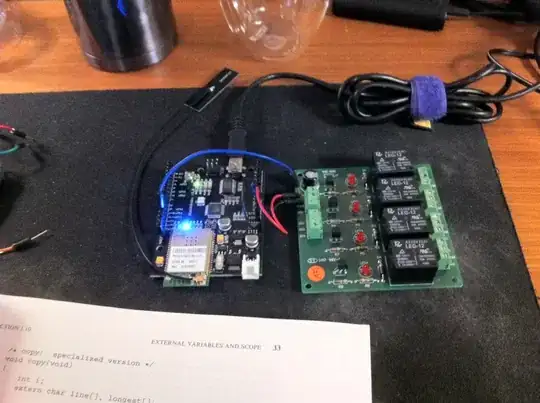
same_cols = np.all(a == a[0, :], axis=0)
same_cols_index = np.where(same_cols==False)[0]
C0,C1 = same_cols_index[0], same_cols_index[-1] + 1
same_rows = np.all(a == a[:, 0], axis=1)
same_rows_index = np.where(same_rows==False)[0]
R0,R1 = same_rows_index[0], same_rows_index[-1] + 1
print('rows', R0, R1)
print('cols', C0, C1)
a_snipped = a[R0:R1, C0:C1]
plt.imshow(a_snipped, cmap='gray', vmin=-3000, vmax=2000)
rows 53 421
cols 43 470
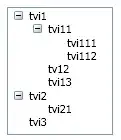