You can do it starting with bounding box using snake algorithm with balloon force added.
Snake's algo is defined such that it minimizes 3 energies - Continuity, Curvature and Gradient. The first two (together called internal energy) get minimized when points (on curve) are pulled closer and closer i.e. contract. If they expand then energy increases which is not allowed by snake algorithm.
But this initial algo proposed in 1987 has a few problems. One of the problem is that in flat areas (where gradient is zero) algo fails to converge and does nothing. There are several modifications proposed to solve this problem. The solution of interest here is - Balloon Force proposed by LD Cohen in 1989.
Balloon force guides the contour in non-informative areas of the image, i.e., areas where the gradient of the image is too small to push the contour towards a border. A negative value will shrink the contour, while a positive value will expand the contour in these areas. Setting this to zero will disable the balloon force.
Another improvement is - Morphological Snakes which use morphological operators (such as dilation or erosion) over a binary array instead of solving PDEs over a floating point array, which is the standard approach for active contours. This makes Morphological Snakes faster and numerically more stable than their traditional counterpart.
Scikit-image's implementation using the above two improvements is morphological_geodesic_active_contour
. It has a parameter balloon
Using your image
import numpy as np
import matplotlib.pyplot as plt
from skimage.segmentation import morphological_geodesic_active_contour, inverse_gaussian_gradient
from skimage.color import rgb2gray
from skimage.util import img_as_float
from PIL import Image, ImageDraw
im = Image.open('knife.jpg')
im = np.array(im)
im = rgb2gray(im)
im = img_as_float(im)
plt.imshow(im, cmap='gray')
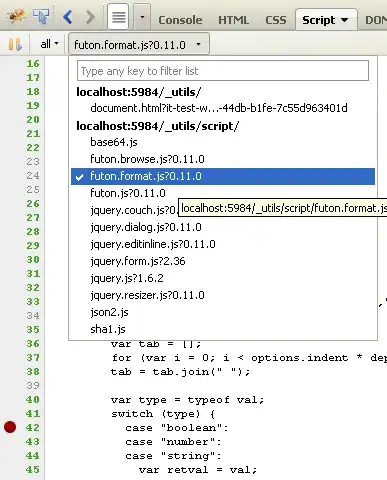
Now let us create a function which will help us to store iterations:
def store_evolution_in(lst):
"""Returns a callback function to store the evolution of the level sets in
the given list.
"""
def _store(x):
lst.append(np.copy(x))
return _store
This method needs image to be preprocessed to highlight the contours. This can be done using the function inverse_gaussian_gradient
, although the user might want to define their own version. The quality of the MorphGAC segmentation depends greatly on this preprocessing step.
gimage = inverse_gaussian_gradient(im)
Below we define our starting point - a bounding box.
init_ls = np.zeros(im.shape, dtype=np.int8)
init_ls[200:-400, 20:-30] = 1
List with intermediate results for plotting the evolution
evolution = []
callback = store_evolution_in(evolution)
Now required magic line for morphological_geodesic_active_contour with balloon contraction is below:
ls = morphological_geodesic_active_contour(gimage, 200, init_ls,
smoothing=1, balloon=-0.75,
threshold=0.7,
iter_callback=callback)
Now let us plot the results:
fig, axes = plt.subplots(1, 2, figsize=(8, 8))
ax = axes.flatten()
ax[0].imshow(im, cmap="gray")
ax[0].set_axis_off()
ax[0].contour(ls, [0.5], colors='b')
ax[0].set_title("Morphological GAC segmentation", fontsize=12)
ax[1].imshow(ls, cmap="gray")
ax[1].set_axis_off()
contour = ax[1].contour(evolution[0], [0.5], colors='r')
contour.collections[0].set_label("Starting Contour")
contour = ax[1].contour(evolution[25], [0.5], colors='g')
contour.collections[0].set_label("Iteration 25")
contour = ax[1].contour(evolution[-1], [0.5], colors='b')
contour.collections[0].set_label("Last Iteration")
ax[1].legend(loc="upper right")
title = "Morphological GAC Curve evolution"
ax[1].set_title(title, fontsize=12)
plt.show()
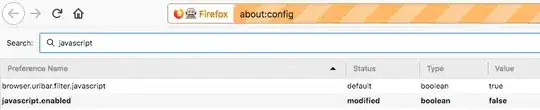
With more balloon force you can get only the blade of knife as well.
ls = morphological_geodesic_active_contour(gimage, 100, init_ls,
smoothing=1, balloon=-1,
threshold=0.7,
iter_callback=callback)
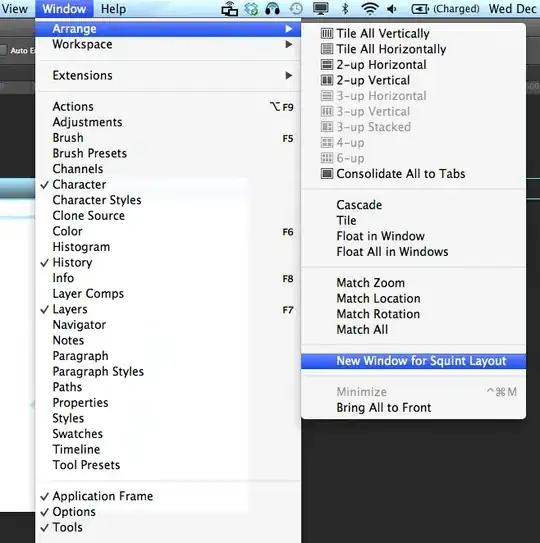
Play with these parameters - smoothing, balloon, threshold
to get your perfect curve