You have to put the total number of pixels in the denominator instead of the number of black pixels.
In [161]: import numpy as np
In [162]: sought = [0, 0, 0]
In [163]: rows = cols = 256
In [164]: original = np.random.randint(
...: low=0, high=256, size=(rows, cols, 3), dtype=np.uint8)
...: original[:rows//4, :, :] = sought
In [165]: masked = original.copy()
...: masked[:, :cols//4, :] = sought
In [166]: black1 = np.count_nonzero((original == sought).all(axis=-1))
In [167]: black2 = np.count_nonzero((masked == sought).all(axis=-1))
In [168]: import matplotlib.pyplot as plt
...: fig, (ax0, ax1) = plt.subplots(1, 2)
...: ax0.imshow(original)
...: ax0.set_title('Original')
...: ax1.imshow(masked)
...: ax1.set_title('Masked')
...: plt.show(fig)
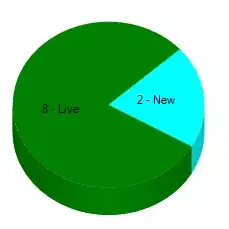
In [169]: reduction = 100*(black2 - black1)/(rows*cols)
In [170]: print(f'Reduction = {reduction:.2f}%')
Reduction = 18.75%
In the example above the increase of black pixels is 1/4 - 1/16 which equals 0,1875.
Edit
Maybe that "the images visually seem to have about 50% reduction", but the reduction is actually 36.61%. Run this code to convince yourself.
In [192]: from skimage import io
In [193]: original = io.imread('https://i.stack.imgur.com/PwVmP.png')
In [194]: masked = io.imread('https://i.stack.imgur.com/OG2aF.png')
In [195]: non_black_pixels_1 = np.logical_not((original == sought).all(axis=-1))
In [196]: black_pixels_2 = (masked == sought).all(axis=-1)
In [197]: new_black_pixels = np.logical_and(black_pixels_2, non_black_pixels_1)
In [198]: import matplotlib.pyplot as plt
...: fig, (ax1, ax2, ax3) = plt.subplots(1, 3)
...: ax1.imshow(original)
...: ax1.set_title('Image 1')
...: ax2.imshow(masked)
...: ax2.set_title('Image 1 Reduced')
...: ax3.imshow(new_black_pixels)
...: ax3.set_title('New black pixels')
...: plt.show(fig)
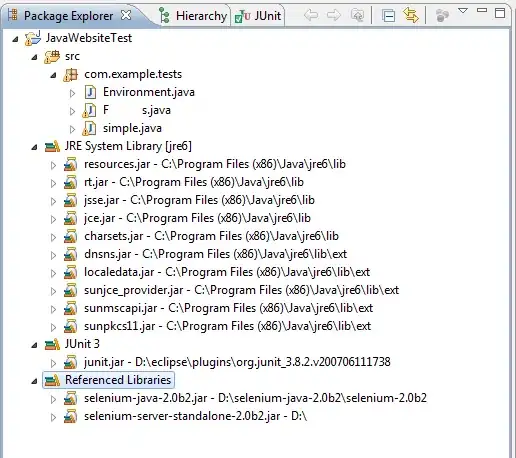
In [199]: reduction = 100*new_black_pixels.mean()
In [200]: print(f'Reduction = {reduction:.2f}%')
Reduction = 36.61%
Edit #2
As per the comments, it seems that you are trying to compute the percentage of non-black pixels that become black after masking. The following snippet does exactly that:
In [219]: nonblack1 = np.count_nonzero((original != sought).any(axis=-1))
In [220]: nonblack2 = np.count_nonzero((masked != sought).any(axis=-1))
In [221]: reduction = 100*(nonblack1 - nonblack2)/nonblack1
In [222]: print(f'Reduction = {reduction:.2f}%')
Reduction = 55.85%