The cross correlation is already telling you how similar they are, only that the similarity is expressed in the metric of the original signals so there's no reason to expect the resulting correlations to be normalized or constrained between 0-1
If you want to normalize the resulting correlation (like in MATLAB, for example), you could try scaling the values by the product of their norms:
a = np.array([0, 80, 83, 86, 85, 82, 84, 85, 86, 85, 87, 84, 85, 88, 89, 88, 87, 88, 86, 87, 88, 85, 86, 84, 83, 85, 83, 82, 84, 83, 81, 83, 80, 82, 83, 81, 79, 80])
b = np.array([0, 81, 82, 85, 86, 81, 85, 84, 87, 84, 88, 83, 86, 89, 88, 87, 88, 87, 87, 86, 89, 86, 85, 83, 84, 86, 84, 81, 83, 84, 82, 84, 81, 83, 82, 82, 80, 79])
xcorr = np.correlate(a,b,'full') / (np.linalg.norm(a) * np.linalg.norm(b))
plt.plot(xcorr)
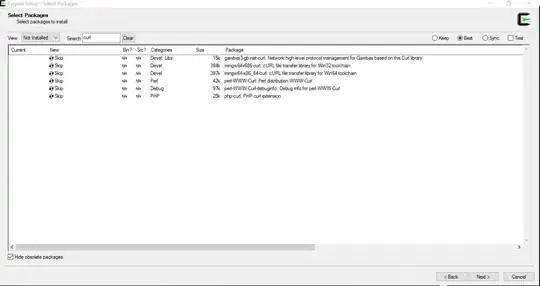
As for why it looks like a triangle, that is due to the way a cross correlation is calculated. You are essentially calculating a correlation at every single time step - imagine sliding one signal over the other. You do this with both the positive lag values (which give you the correlations after your peak), as well as the negative lags (which give you the values before the peak). Your 0-lag correlation will be the peak in the middle.
Also, if you print
your cross correlation values, you'll notice that the values aren't actually perfectly symmetrical, it only looks that way in the figure due to the similarity of your 2 signals:
[0. 0.02400722 0.0492186 0.07594942 0.10241434 0.12830187
0.1541742 0.1812317 0.20800811 0.2351112 0.26253336 0.28842089
0.31552398 0.34409333 0.37248414 0.40026338 0.42832372 0.45693105
0.48596003 0.51442302 0.54342921 0.57155412 0.60023743 0.62805465
0.65586048 0.68463116 0.7118596 0.7378231 0.76583785 0.79238634
0.81931089 0.84595435 0.87183428 0.89713682 0.92421712 0.95038574
0.9751033 0.99993102 0.97567309 0.95038574 0.92356755 0.89708744
0.87113913 0.84522121 0.81801557 0.79107962 0.76396894 0.7364518
0.71045791 0.68327505 0.65454616 0.62608697 0.59827735 0.57016003
0.54142354 0.51244774 0.48403794 0.45500136 0.42702839 0.3982881
0.37053925 0.34283979 0.31426664 0.28709518 0.26126083 0.23321189
0.20673178 0.18000475 0.15355503 0.1276599 0.10241054 0.07536063
0.04922619 0.024615 0. ]