After properly masking all red pixels, you can calculate the cumulative sum per each column in that mask:
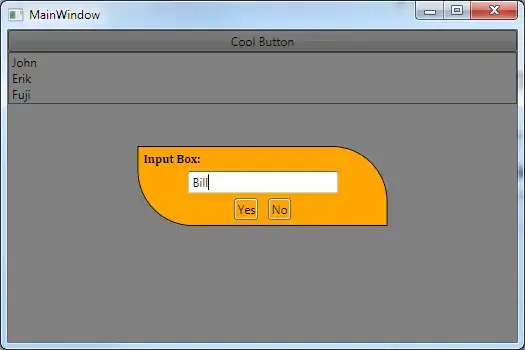
Below each red line, you have a large area with a constant value: Below the first red line, it's the thickness of that red line. Below the second red line, it's the cumulative thickness of both red lines, and so on (if there would be even more red lines).
So, now, for each column, calculate the histogram from the cumulative sum, and filter out these peaks; leaving out the 0
in the histogram, that'd be the large black area at the top. Per column, you get the above mentioned (cumulative) thickness values for all red lines. The remainder is to extract the actual, single thickness values, and calculate the mean over all those.
Here's my code:
import cv2
import numpy as np
# Read image
img = cv2.imread('Dc4zq.png')
# Mask RGB pure red
mask = (img == [0, 0, 255]).all(axis=2)
# We check for two lines
n = 2
# Cumulative sum for each column
cs = np.cumsum(mask, axis=0)
# Thickness values for each column
tvs = np.zeros((n, img.shape[1]))
for c in range(img.shape[1]):
# Calculate histogram of cumulative sum for a column
hist = np.histogram(cs[:, c], bins=np.arange(img.shape[1]+1))
# Get n highest histogram values
# These are the single thickness values for a column
tv = np.sort(np.argsort(hist[0][1:])[::-1][0:n]+1)
tv[1:] -= tv[:-1]
tvs[:, c] = tv
# Get mean thickness value
mtv = np.mean(tvs.flatten())
print('Mean thickness value:', mtv)
The final result is:
Mean thickness value: 18.92982456140351
----------------------------------------
System information
----------------------------------------
Platform: Windows-10-10.0.16299-SP0
Python: 3.9.1
NumPy: 1.20.1
OpenCV: 4.5.1
----------------------------------------
EDIT: I'll provide some more details on the "NumPy magic" involved.
# Calculate the histogram of the cumulative sum for a single column
hist = np.histogram(cs[:, c], bins=np.arange(img.shape[1] + 1))
Here, bins
represent the intervals for the histogram, i.e. [0, 1]
, [1, 2]
, and so on. To also get the last interval [569, 570]
, you need to use img.shape[1] + 1
in the np.arange
call, because the right limit is not included in np.arange
.
# Get the actual histogram starting from bin 1
hist = hist[0][1:]
In general, np.histogram
returns a tuple, where the first element is the actual histogram. We extract that, and only look at all bins larger 0
(remember, the large black area).
Now, let's disassemble this code:
tv = np.sort(np.argsort(hist[0][1:])[::-1][0:n]+1)
This line can be rewritten as:
# Get the actual histogram starting from bin 1
hist = hist[0][1:]
# Get indices of sorted histogram; these are the actual bins
hist_idx = np.argsort(hist)
# Reverse the found indices, since we want those bins with the highest counts
hist_idx = hist_idx[::-1]
# From that indices, we only want the first n elements (assuming there are n red lines)
hist_idx = hist_idx[:n]
# Add 1, because we cut the 0 bin
hist_idx = hist_idx + 1
# As a preparation: Sort the (cumulative) thickness values
tv = np.sort(hist_idx)
By now, we have the (cumulative) thickness values for each column. To reconstruct the actual, single thickness values, we need the "inverse" of the cumulative sum. There's this nice Q&A on that topic.
# The "inverse" of the cumulative sum to reconstruct the actual thickness values
tv[1:] -= tv[:-1]
# Save thickness values in "global" array
tvs[:, c] = tv