In case you're interested in a quick visual comparison of Levenshtein and Difflib similarity, I calculated both for ~2.3 million book titles:
import codecs, difflib, Levenshtein, distance
with codecs.open("titles.tsv","r","utf-8") as f:
title_list = f.read().split("\n")[:-1]
for row in title_list:
sr = row.lower().split("\t")
diffl = difflib.SequenceMatcher(None, sr[3], sr[4]).ratio()
lev = Levenshtein.ratio(sr[3], sr[4])
sor = 1 - distance.sorensen(sr[3], sr[4])
jac = 1 - distance.jaccard(sr[3], sr[4])
print diffl, lev, sor, jac
I then plotted the results with R:
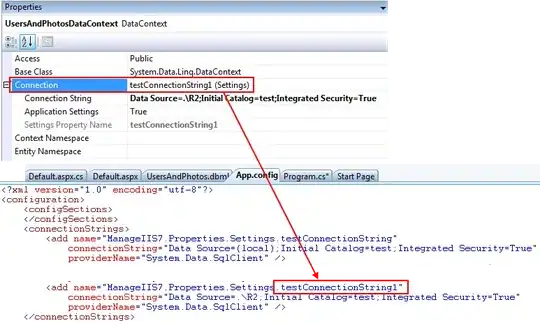
Strictly for the curious, I also compared the Difflib, Levenshtein, Sørensen, and Jaccard similarity values:
library(ggplot2)
require(GGally)
difflib <- read.table("similarity_measures.txt", sep = " ")
colnames(difflib) <- c("difflib", "levenshtein", "sorensen", "jaccard")
ggpairs(difflib)
Result:
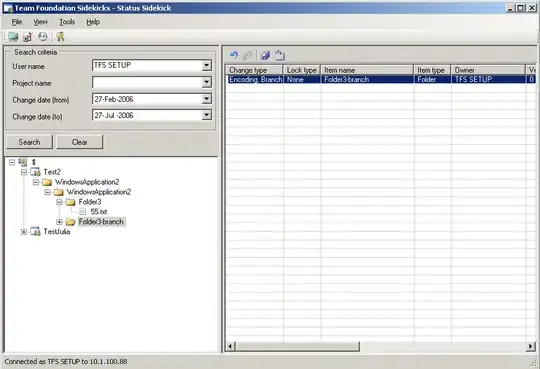
The Difflib / Levenshtein similarity really is quite interesting.
2018 edit: If you're working on identifying similar strings, you could also check out minhashing--there's a great overview here. Minhashing is amazing at finding similarities in large text collections in linear time. My lab put together an app that detects and visualizes text reuse using minhashing here: https://github.com/YaleDHLab/intertext