One approach could be using morphological reconstruction, although it would probably be an ad-hoc solution.
Assuming the maze is always in the center of the photo, you could use a "window" of the central portion of the maze as seed/mark for a morphological reconstruction of the maze given that all borders are connected.
As a result you would have isolated the maze out of the photo.
The (x,y) of the corners would be "easy" to get by obtaining the bounding box of the isolated maze.
For example:
from skimage.morphology import reconstruction
from skimage.io import imread, imshow
import matplotlib.pyplot as plt
import numpy as np
maze = imread('/home/user/.../maze.jpg', as_gray=True)
h, w = maze.shape
#creating the seed
seed = np.zeros_like(maze)
size = 40
#we create a window in the center of the image
seed[h//2-size:h//2+size, w//2-size:w//2+size] = maze[h//2-size:h//2+size, w//2-size:w//2+size]
seed
would contain a centered portion of the maze with a black frame. Keep in mind, seed
must be the same size as maze
, thus the existence of the frame.
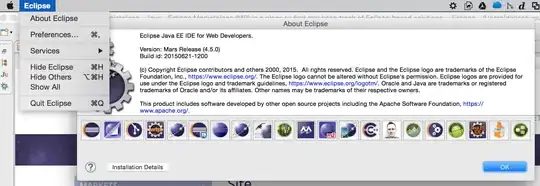
We apply the reconstruction for the first time and binarize the result. By default, reconstruction
uses a structural element with (3,3) shape. The values for the threshold can be adjusted depending the image.
rec_1 = reconstruction(seed, maze)
rec_1[rec_1 < 0.70] = 0.
rec_1[rec_1 >= 0.70] = 1.
This is rec_1
:
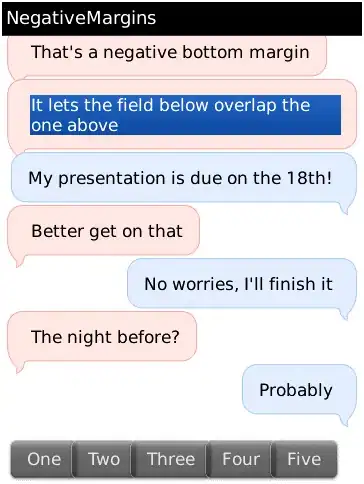
It's good but we can make it a little bit better. Let's apply reconstruction
again, but this time using a bigger window and erosion
instead of dilation
as the reconstruction method:
seed_2 = np.ones_like(rec_1)
size_2 = 240
seed_2[h//2-size_2:h//2+size_2, w//2-size_2:w//2+size_2] = recon[h//2-size_2:h//2+size_2, w//2-size_2:w//2+size_2]
rec_2 = reconstruction(seed_2, rec_1, method='erosion', selem=np.ones((11,11)))
Notice I'm using a bigger structural element too, with a shape of (11,11)
.
The final reconstruction step gives us this result:
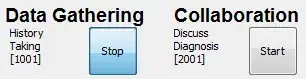
The following step would be to use a bounding box method to get the top left and bottom right (x, y) coordinates.
Those results will be an approximation given that in the original image, the maze is not perfectly flat and we would be relying the fact that the maze's entry and exit are exactly in those positions and not anywhere else in the maze.
Also, this can probably be done with fewer steps.
UPDATE:
As suggested, the exact coordinates can be obtained by using convex hull, binary erosion and corner detection techniques instead of a bounding box method.
First we invert rec_2
, then we get the convex hull, apply erosion to shrink the size of the rectangle and calculate the corner peaks coordinates.
from skimage.util import invert
from skimage.morphology import binary_erosion
from skimage.morphology.convex_hull import convex_hull_image
from skimage.feature import corner_harris, corner_subpix
rec_2_inv = invert(rec_2)
hull = convex_hull_image(rec_2_inv)
hull_eroded = binary_erosion(hull, selem=np.ones(30,30))
coords = corner_peaks(corner_harris(hull_eroded), min_distance=5, threshold_rel=0.02)
coords_subpix = corner_subpix(rec_2_inv, coords, window_size=13)[2:4]
This is the eroded convex hull:
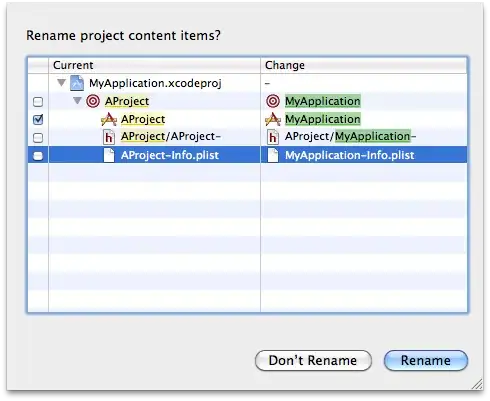
Finally we plot the final image with the coordinates over it:
fig, ax = plt.subplots()
ax.imshow(rec_2_inv, cmap=plt.cm.gray)
ax.plot(coords_subpix[:, 1], coords_subpix[:, 0], '+r', markersize=15)
plt.savefig('maze_with_coordinates.png', bbox_tight=True)
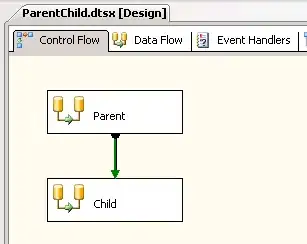
Where coords_subpix
holds the numerical values of the coordinates and I purposely assign two values instead of four.
Those values are:
[[1611.48876404 104.50561798]
[2695.07777778 1679.67222222]]
Most of the update code was done using scikit-image example's parameters with minimal tweaking.